I compared the performance of 3 approaches on M2090 with CUDA 5.0.
- [173.179 us] cublas implementation as shown in the question
- [733.734 us] pure Thrust implementation with
thrust::reduce_by_key
from @talonmies
- [1.508 ms] pure Thrust implementation with
thrust::inclusive_scan_by_key
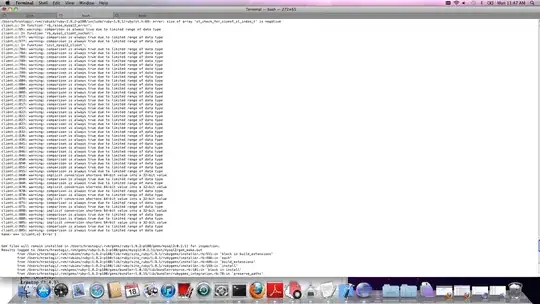
It can be seen that,
- cublas has highest performance in this case;
- both
thrust::reduce_by_key
& thrust::inclusive_scan_by_key
launch multiple kernels, which lead to extra overhead;
thrust::inclusive_scan_by_key
writes much more data to DRAM compared to thrust::reduce_by_key
, which can be one of the reasons for longer kernel time;
- the main performance difference between cublas and thrust approach is the matrix column summation. thrust is slower possibly because
thrust::reduce_by_key
is designed to do reduction on segments with variant length, but cublas_gemv()
can only apply to fixed length segments (row/col).
When the matrix A is large enough to ignore the kernel launching overhead, the cublas appoach still performs best. The profiling result on A_{20,000 x 2,000} is shown as follows.
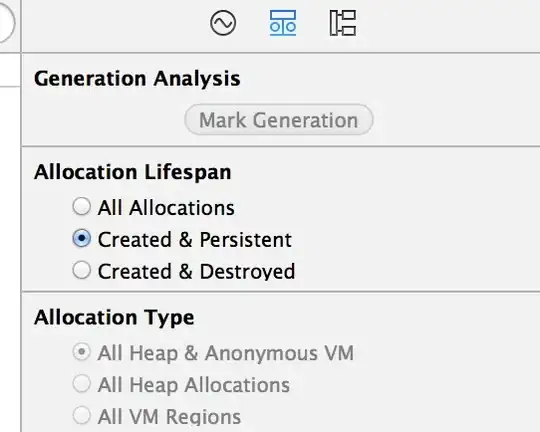
Fusing the first for_each
operation with the cublasSgemv
call as indicated by @talonmies may further improve the performance, but I think kernel written by hand should be used instead of thrust::reduce_by_key
.
The code for the 3 approaches is shown as follows.
#include <cuda.h>
#include <curand.h>
#include <cublas_v2.h>
#include <thrust/device_vector.h>
#include <thrust/device_ptr.h>
#include <thrust/transform.h>
#include <thrust/reduce.h>
#include <thrust/scan.h>
#include <thrust/iterator/counting_iterator.h>
#include <thrust/iterator/transform_iterator.h>
#include <thrust/iterator/discard_iterator.h>
#include <thrust/iterator/permutation_iterator.h>
#include <math.h>
struct Exp: public thrust::unary_function<double, double>
{
__host__ __device__ double operator()(double x)
{
return exp(x);
}
};
struct Inv: public thrust::unary_function<double, double>
{
__host__ __device__ double operator()(double x)
{
return (double) 1.0 / x;
}
};
template<typename T>
struct MulC: public thrust::unary_function<T, T>
{
T C;
__host__ __device__ MulC(T c) :
C(c)
{
}
__host__ __device__ T operator()(T x)
{
return x * C;
}
};
template<typename T>
struct line2col: public thrust::unary_function<T, T>
{
T C;
__host__ __device__ line2col(T C) :
C(C)
{
}
__host__ __device__ T operator()(T i)
{
return i / C;
}
};
int main()
{
cudaDeviceSetCacheConfig(cudaFuncCachePreferShared);
cublasHandle_t hd;
curandGenerator_t rng;
cublasCreate(&hd);
curandCreateGenerator(&rng, CURAND_RNG_PSEUDO_DEFAULT);
const size_t m = 2000, n = 200;
const double c1 = 1.0;
const double c0 = 0.0;
thrust::device_vector<double> A(m * n);
thrust::device_vector<double> B(m * n);
thrust::device_vector<double> C(m * n);
thrust::device_vector<double> sum1(1 * n);
thrust::device_vector<double> sum2(1 * n);
thrust::device_vector<double> one(m * n, 1);
double* pA = thrust::raw_pointer_cast(&A[0]);
double* pB = thrust::raw_pointer_cast(&B[0]);
double* pSum1 = thrust::raw_pointer_cast(&sum1[0]);
double* pSum2 = thrust::raw_pointer_cast(&sum2[0]);
double* pOne = thrust::raw_pointer_cast(&one[0]);
curandGenerateUniformDouble(rng, pA, A.size());
const int count = 2;
for (int i = 0; i < count; i++)
{
thrust::transform(A.begin(), A.end(), B.begin(), Exp());
cublasDgemv(hd, CUBLAS_OP_T, m, n, &c1, pB, m, pOne, 1, &c0, pSum1, 1);
thrust::transform(sum1.begin(), sum1.end(), sum1.begin(), Inv());
cublasDdgmm(hd, CUBLAS_SIDE_RIGHT, m, n, pB, m, pSum2, 1, pB, m);
}
for (int i = 0; i < count; i++)
{
thrust::reduce_by_key(
thrust::make_transform_iterator(thrust::make_counting_iterator(0), line2col<int>(m)),
thrust::make_transform_iterator(thrust::make_counting_iterator(0), line2col<int>(m)) + A.size(),
thrust::make_transform_iterator(A.begin(), Exp()),
thrust::make_discard_iterator(),
sum2.begin());
thrust::transform(
A.begin(), A.end(),
thrust::make_permutation_iterator(
sum2.begin(),
thrust::make_transform_iterator(thrust::make_counting_iterator(0), line2col<int>(m))),
C.begin(),
thrust::divides<double>());
}
for (int i = 0; i < count; i++)
{
thrust::inclusive_scan_by_key(
thrust::make_transform_iterator(thrust::make_counting_iterator(0), line2col<int>(m)),
thrust::make_transform_iterator(thrust::make_counting_iterator(0), line2col<int>(m)) + A.size(),
thrust::make_transform_iterator(A.begin(), Exp()),
C.begin());
thrust::copy(
thrust::make_permutation_iterator(
C.begin() + m - 1,
thrust::make_transform_iterator(thrust::make_counting_iterator(0), MulC<int>(m))),
thrust::make_permutation_iterator(
C.begin() + m - 1,
thrust::make_transform_iterator(thrust::make_counting_iterator(0), MulC<int>(m))) + n,
sum2.begin());
thrust::transform(
A.begin(), A.end(),
thrust::make_permutation_iterator(
sum2.begin(),
thrust::make_transform_iterator(thrust::make_counting_iterator(0), line2col<int>(m))),
C.begin(),
thrust::divides<double>());
}
curandDestroyGenerator(rng);
cublasDestroy(hd);
return 0;
}