The points in my comment withstanding, here is the canonical way to generate output from a fitted model in R for combinations of predictors. It really isn't clear what the plots you want are showing, but the ones that make sense to me are partial plots; where one variable is varied over its range whilst holding the others at some common value. Here I use the sample mean when holding a variable constant.
First some dummy data, with only to covariates, but this extends to any number
set.seed(1)
dat <- data.frame(y = rnorm(100))
dat <- transform(dat,
x1 = 0.2 + (0.4 * y) + rnorm(100),
x2 = 2.4 + (2.3 * y) + rnorm(100))
Fit the regression model
mod <- lm(y ~ x1 + x2, data = dat)
Next some data values to predict at using the model. You could do all variables in a single prediction and then subset the resulting object to plot only the relevant rows. Alternatively, more clearly (though more verbose), you can deal with each variable separately. Below I create two data frames, one per covariate in the model. In a data frame I generate 100 values over the range of the covariate being varied, and repeat the mean value of the other covariate(s).
pdatx1 <- with(dat, data.frame(x1 = seq(min(x1), max(x1), length = 100),
x2 = rep(mean(x2), 100)))
pdatx2 <- with(dat, data.frame(x1 = rep(mean(x1), 100),
x2 = seq(min(x2), max(x2), length = 100)))
In the linear regression with straight lines, you really don't need 100 values --- the two end points of the range of the covariate will do. However for models where the fitted function is not linear you need to predict at more locations.
Next, use the model to predict at these data points
pdatx1 <- transform(pdatx1, yhat = predict(mod, pdatx1))
pdatx2 <- transform(pdatx2, yhat = predict(mod, pdatx2))
Now we are ready to draw the partial plots. First compute a range for the y
axis - again it is mostly redundant here but if you are adding confidence intervals you will need to include their values below,
ylim <- range(pdatx1$y, pdatx2$y, dat$y)
To plot (here putting two figures on the same plot device) we can use the following code
layout(matrix(1:2, ncol = 2))
plot(y ~ x1, data = dat)
lines(yhat ~ x1, data = pdatx1, col = "red", lwd = 2)
plot(y ~ x2, data = dat)
lines(yhat ~ x2, data = pdatx2, col = "red", lwd = 2)
layout(1)
Which produces
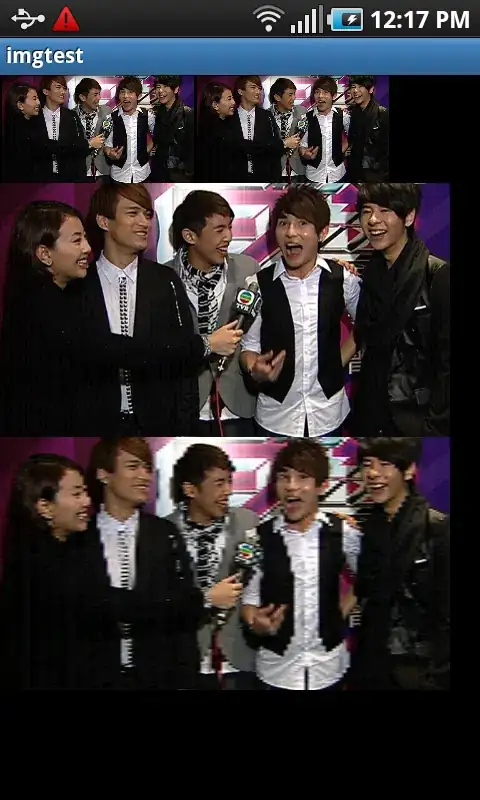