I have tested some solutions using numpy. Through trial and error method, I found out that ± variation
divided by 3 is a good guess for standard deviation.
Following you have some examples:
The basics
import numpy as np
import matplotlib.pyplot as plt
val_min = 1000
val_max = 2000
variation = (val_max - val_min)/2
std_dev = variation/3
mean = (val_max + val_min)/2
dist_normal = np.random.normal(mean, std_dev, 1000)
print('Normal distribution\n\tMin: {0:.2f}, Max: {1:.2f}'
.format(dist_normal.min(), dist_normal.max()))
plt.hist(dist_normal, bins=30)
plt.show()
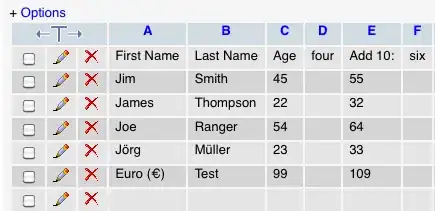
A comparative case
import numpy as np
import matplotlib.pyplot as plt
val_min = 1400
val_max = 2800
variation = (val_max - val_min)/2
std_dev = variation/3
mean = (val_max + val_min)/2
fig, ax = plt.subplots(3, 3)
plt.suptitle("Histogram examples by Davidson Lima (github.com/davidsonlima)",
fontweight='bold')
i = 0
j = 0
pos = 1
while (i < 3):
while (j < 3):
dist_normal = np.random.normal(mean, std_dev, 1000)
max_min = 'Min: {0:.2f}, Max: {1:.2f}'.format(dist_normal.min(), dist_normal.max())
ax[i, j].hist(dist_normal, bins=30, label='Dist' + str(pos))
ax[i, j].set_title('Normal distribution ' + str(pos))
ax[i, j].legend()
ax[i, j].text(mean, 0, max_min, horizontalalignment='center', color='white',
bbox={'facecolor': 'red', 'alpha': 0.5})
print('Normal distribution {0}\n\tMin: {1:.2f}, Max: {2:.2f}'
.format(pos, dist_normal.min(), dist_normal.max()))
j += 1
pos += 1
j = 0
i += 1
plt.show()

If someone has a better approach with numpy, please comment below.