Other possible approaches to count occurrences could be to use (i) Counter
from collections
module, (ii) unique
from numpy
library and (iii) groupby
+ size
in pandas
.
To use collections.Counter
:
from collections import Counter
out = pd.Series(Counter(df['word']))
To use numpy.unique
:
import numpy as np
i, c = np.unique(df['word'], return_counts = True)
out = pd.Series(c, index = i)
To use groupby
+ size
:
out = pd.Series(df.index, index=df['word']).groupby(level=0).size()
One very nice feature of value_counts
that's missing in the above methods is that it sorts the counts. If having the counts sorted is absolutely necessary, then value_counts
is the best method given its simplicity and performance (even though it still gets marginally outperformed by other methods especially for very large Series).
Benchmarks
(if having the counts sorted is not important):
If we look at runtimes, it depends on the data stored in the DataFrame columns/Series.
If the Series is dtype object, then the fastest method for very large Series is collections.Counter
, but in general value_counts
is very competitive.
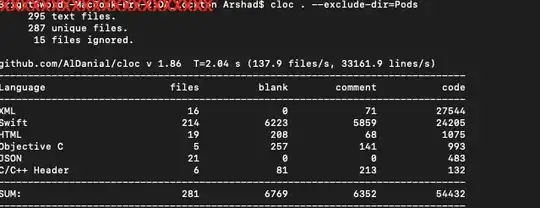
However, if it is dtype int, then the fastest method is numpy.unique
:
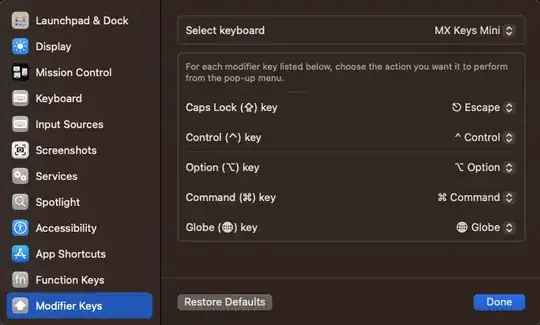
Code used to produce the plots:
import perfplot
import numpy as np
import pandas as pd
from collections import Counter
def creator(n, dt='obj'):
s = pd.Series(np.random.randint(2*n, size=n))
return s.astype(str) if dt=='obj' else s
def plot_perfplot(datatype):
perfplot.show(
setup = lambda n: creator(n, datatype),
kernels = [lambda s: s.value_counts(),
lambda s: pd.Series(Counter(s)),
lambda s: pd.Series((ic := np.unique(s, return_counts=True))[1], index = ic[0]),
lambda s: pd.Series(s.index, index=s).groupby(level=0).size()
],
labels = ['value_counts', 'Counter', 'np_unique', 'groupby_size'],
n_range = [2 ** k for k in range(5, 25)],
equality_check = lambda *x: (d:= pd.concat(x, axis=1)).eq(d[0], axis=0).all().all(),
xlabel = '~len(s)',
title = f'dtype {datatype}'
)
plot_perfplot('obj')
plot_perfplot('int')