For multiple conditions e.g. COUNTIFS/SUMIFS, a convenient method is query
because it's very fast for large frames (where performance actually matters) and you don't need to worry about parentheses, bitwise-and etc. For example, to compute =SUMIFS(C2:C8, A2:A8,">1", B2:B8, "<3")
, you can use
df.query("A>1 and B<3")['C'].sum()
# or
df.iloc[:8].query("A>1 and B<3")['C'].sum() # where the range is specified as in SUMIFS
For COUNTIFS, you can simply sum over the condition. For example, to compute =COUNTIFS(A2:A8,">0", B2:B8, "<3")
, you can do:
countifs = ((df['A']>1) & (df['B']<3)).sum()
or just call query
and compute the length of the result.
countifs = len(df.query("A>1 and B<3"))
You can also specify the range similar to how range is fed to COUNTIFS using iloc
:
countifs = len(df.iloc[:8].query("A>1 and B<3"))
To perform row-wise COUNTIF/SUMIF, you can use axis=1
argument. Again, the range is given as a list of columns (['A', 'B']
) similar to how range is fed to COUNTIF.
Also for COUNTIF (similar to the pandas equivalent of COUNTIFS), it suffices to sum over the condition while for SUMIF, we need to index the frame.
df['COUNTIF'] = (df[['A', 'B']] > 1).sum(axis=1)
df['SUMIF'] = df[df[['A', 'B']] > 1].sum(axis=1)
# equivalently, we can use `where` to make a filter as well
df['SUMIF'] = df.where(df[['A', 'B']] > 1, 0).sum(axis=1)
# can use `agg` to compute countif and sumif in one line.
df[['COUNTIF', 'SUMIF']] = df[df[['A', 'B']] > 1].agg(['count', 'sum'], axis=1)
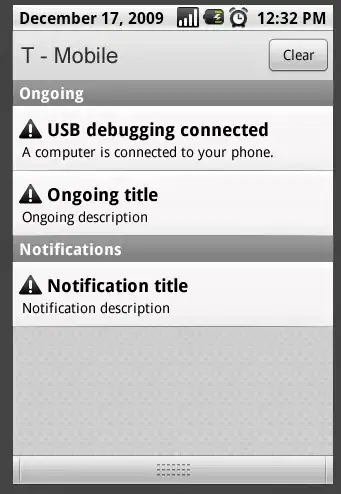
To perform column-wise COUNTIF/SUMIF, you can use axis=0
argument (which it is by default). The range here (the first 3 rows) is selected using iloc
.
df.loc['COUNTIF'] = (df.iloc[:3] > 1).sum()
df.loc['SUMIF'] = df.where(df.iloc[:3] > 1, 0).sum()
# or
df.loc['SUMIF'] = df[df.iloc[:3] > 1].sum()
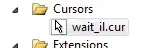
For COUNTIF/SUMIF across multiple rows/columns, e.g. =COUNTIF(A2:B4, ">1")
, call sum
twice (once for the column-wise sum and then across columns-sums).
countif = (df.iloc[:4, :2]>1).sum().sum() # the range is determined using iloc
sumif = df[df.iloc[:4, :2] > 1].sum().sum() # first 4 rows and first 2 columns