Asymptotic complexity (which is what both big-O and big-Theta represent) completely ignores the constant factors involved - it's only intended to give an indication of how running time will change as the size of the input gets larger.
So it's certainly possible that an Θ(n)
algorithm can take longer than an Θ(n2)
one for some given n
- which n
this will happen for will really depend on the algorithms involved - for your specific example, this will be the case for n < 100
, ignoring the possibility of optimizations differing between the two.
For any two given algorithms taking Θ(n)
and Θ(n2)
time respectively, what you're likely to see is that either:
- The
Θ(n)
algorithm is slower when n
is small, then the Θ(n2)
one becomes slower as n
increases
(which happens if the Θ(n)
one is more complex, i.e. has higher constant factors), or
- The
Θ(n2)
one is always slower.
Although it's certainly possible that the Θ(n)
algorithm can be slower, then the Θ(n2)
one, then the Θ(n)
one again, and so on as n
increases, until n
gets very large, from which point onwards the Θ(n2)
one will always be slower, although it's greatly unlikely to happen.
In slightly more mathematical terms:
Let's say the Θ(n2)
algorithm takes cn2
operations for some c
.
And the Θ(n)
algorithm takes dn
operations for some d
.
This is in line with the formal definition since we can assume this holds for n
greater than 0 (i.e. for all n
) and that the two functions between which the running time is lies is the same.
In line with your example, if you were to say c = 1
and d = 100
, then the Θ(n)
algorithm would be slower until n = 100
, at which point the Θ(n2)
algorithm would become slower.
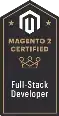
(courtesy of WolframAlpha).
Notation note:
Technically big-O is only an upper bound, meaning you can say an O(1)
algorithm (or really any algorithm taking O(n2)
or less time) takes O(n2)
as well. Thus I instead used big-Theta (Θ) notation, which is just a tight bound. See the formal definitions for more information.
Big-O is often informally treated as or taught to be a tight bound, so you may already have been essentially using big-Theta without knowing it.
If we're talking about an upper bound only (as per the formal definition of big-O), that would rather be an "anything goes" situation - the O(n)
one can be faster, the O(n2)
one can be faster or they can take the same amount of time (asymptotically) - one usually can't make particularly meaningful conclusions with regard to comparing the big-O of algorithms, one can only say that, given a big-O of some algorithm, that that algorithm won't take any longer than that amount of time (asymptotically).