It is an open problem and there are dozen of strategies based on situations. based on my knowledge, I list a short highlights of some of well known Auto Completion strategies and their corresponding data structures. also I tried to summarizing their major pros and cons related to Auto Completion problem.
Brute Force :
- pros: can achieved by checking all universe(input) as the next step
- pros: super easy
- pros: it's good for tiny dataset with limited states
- cons: connections doesn't stored so each time you have to perform a search
- cons: has the worst time complexity.
Prefixed Tree( Trie ) :
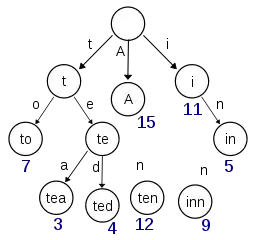
- pros: it is the simplest data structure designed for these kind of problems.
- pros: list of all available next states are stored in each state.
- cons: data size should be small( at most should be a low fractional of RAM size).
Directed Acyclic Graph (DAG) :
Trie has space problem so other data structures main goal is reducing the space complexity. Directed Acyclic Graph (DAG) is one of the options. by using DAG you can merge all similar subpath to only one. as a result much of space will be reserved.
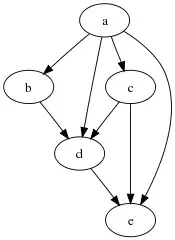
fast-autocomplete repository is in this area which uses Directed Word Graph (DWG) and Levenshtein Edit Distance.
Some other Tree options :
On each state(or Node) there is a search problem. linear search is the worst case option so most strategies improved their search time by using either sorting( O(nlog(n) ) and then using Binary search( O(log(n)) ) or using a hashtable ( O(1), fast but has more space complexity). encountering so many trade-off dilemmas, other tree data structure variants like Radix Tree, Suffix Tree, Suggest Tree and Merkle Tree may come useful.
Prioritizig Offers :
Markov Chain can be used to prioritize next states. it stores connection probabilities which leads to more accurate results.
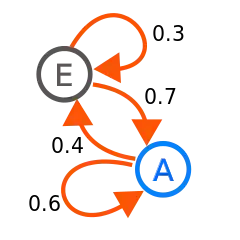
Artificial Intelligence strategies:
- pros: good models are small and running fast.
- pros: good models just stores discriminative features.
- pros: huge dataset friedly
- pros: Natural Language Processing (NLP ) frameworks has predefined algorithms (higher level API)
- pros: long term learning power is greatly increased.
- cons: understanding input topology is important.
- cons: preprocessing is hard process.
- cons: training time is long.
- cons: convergence may needs too many retrying and retraining which is a hard process.
Long short term memory (LSTM ) :
there are so many useful Machine Learning and Deep Learning strategies. a good strategy, you can consider Auto Completion as a time series problem so you can use some models like LSTM.
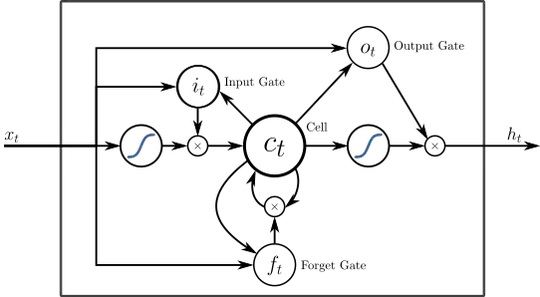
Transformers :
The last but not least, I recommend Transformer models. currently they are game changers. a great Transformer based language model is Google BERT. it is highly promising on predicting future sequences.
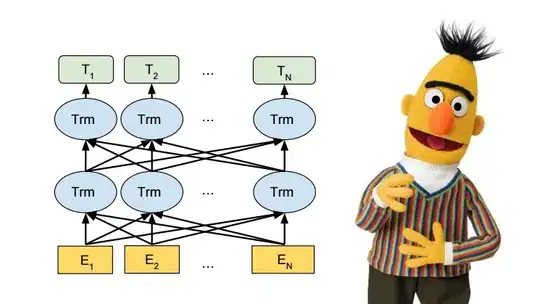
I also recommend, before starting your Auto Completion project using transformers, please take a look at python_autocomplete repository which uses Transformers and LSTMs to learn Python source code.
Good luck!