What happens when you run these routines over a set? Do you get a bias
like rand()?
The answer is: this depends on the relation between the size of range returned by the generator and the divisor in modulo operation. If divisor not evenly divides the range then distribution will be skewed. The bias ratio is in the range [ 1, 2], where 1 means no bias ( as for uniform distribution) and the bias increases with divisor. Regarding arcrandom4()
this translates to skewed distribution obtained in all cases when modulo divisor is not an even divisor of 2^32. The rationale behind it is explained below.
Introduction. The bias
Imagine that we are trying to simulate uniform int distribution over interval [0, 99] with
int x = rand() % 100;
Operator % makes the probability distribution of X skewed because RAND_MAX which is maximum value for rand() can be not equal to k * 100 + 99. This results in that if you imagine all 100-length parts of 0-RAND_MAX range then you can see that last part will probably not produce a full range 0-99. Therefore you have more numbers that generates 0, 1, 2..., p but not necessary p + 1, ..., 98, 99 ( 1 more occurrence for each number in 0, 1, 2, ..., p). The inaccuracy of this approach increases with bigger divisor that not divides the range evenly and maximum bias compared to uniform distribution is equal 2.
In the following sections below we show that the bias measured as a ratio of probability of getting number from [ 0, p] to probability of number from [ p + 1, n] is equal to ( k + 1) / k and we confirm this with 2 examples.
Formula
We will show what exactly is the bias introduced by operation modulo ( operation that is applied to generator of uniform distribution in order to trim the output range). We will operate in terms of formula
x = rand() % ( n + 1)
where rand()
is some generator and ( n + 1)
is divisor in modulo operation. The picture below shows our standpoint:
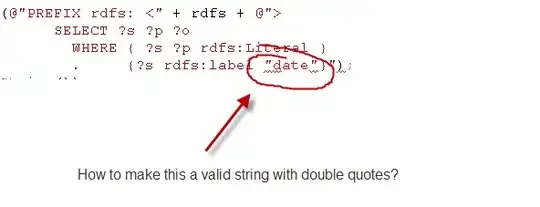
We can see how numbers in range [ 0, n]
are divided into these that repeat k + 1
times (numbers [ 0, p]
) and these that repeats k
times ( numbers [ p + 1, n]
) in a single trial, which is "take the number from the distribution obtained by x = rand() % (n+1)
". The p is defined as a remainder when dividing the maximum number ( i.e. Rand_MAX) given by the generator by the ( n + 1) which is the size of desired range:
p = ( N - 1) % ( n + 1)
N - 1 = k * ( n + 1) + p
and the k is the quotient
k = ( N - 1 - p) / ( n + 1)
In a single trial there are
( p + 1) * ( k + 1) + ( n - p) * k =
= p + 1 + k( n + 1) = N
possible outcomes. Thus the probability of receiving the element that repeats k times is k / N. Let's denote
f_0 = ( k + 1) / N, probability for each element from [ 0, p]
f_1 = k / N, probability for each element from [ p + 1, n]
Let's say that we will express the bias of sampling from this, transformed distribution over the uniform distribution as the ratio of probability of element that belongs to [ 0, p]
to probability of element from the range [ p + 1, n]
:
bias = f_0 / f_1 = ( k + 1) / k
So, are numbers twice as often?
No. The fact that when we look at the picture numbers repeats doesn't imply the ratio of 2. This ratio is just a special case, if range of the generator is divided into exactly 2 subranges. In general the bias ratio is( k + 1) / k and decreases asymptotically, when divisor n + 1 tends to 1, ( and k tends to N).
Examples
We now consider two simple examples (as suggested by @dyp). First we will generate 1000 * 1000 samples from a distribution given by
x = rand() % m
with generator being std::uniform_int_distribution<> dist(0, 19)
and divisor m = n + 1 equal to 15 and next equal to 6.
Example 1
int x = rand() % 15; // n + 1 = 15, rand is uniform distribution over [0,19]
Test program is:
#include <iostream>
#include <random>
#include <vector>
int main()
{
std::random_device rd;
std::mt19937 mt(rd());
std::uniform_int_distribution<> dist(0, 19);
std::vector<int> v(15);
const int runs = 1000 * 1000;
for (int i = 0; i < runs; ++i)
{
++v[dist(mt) % v.size()];
}
for (int i = 0; i < v.size(); ++i)
{
std::cout << i << ": " << v[i] << "\n";
}
}
code
result:
0: 100500
1: 100016
2: 99724
3: 99871
4: 99936
5: 50008
6: 49762
7: 50023
8: 50123
9: 49963
10: 50117
11: 50049
12: 49885
13: 49760
14: 50263
We can see that in this case numbers in range [ 0, p] = [ 0, 4] appears about twice as often as the rest. This is in accordance with our bias formula
bias = f_0 / f_1 = ( k + 1) / k = 2 / 1
Example 2
int x = rand() % 6; // n + 1 = 6, rand is uniform distribution over [0,19]
Test program is:
#include <iostream>
#include <random>
#include <vector>
int main()
{
std::random_device rd;
std::mt19937 mt(rd());
std::uniform_int_distribution<> dist(0, 19);
std::vector<int> v(6);
const int runs = 1000 * 1000;
for (int i = 0; i < runs; ++i)
{
++v[dist(mt) % v.size()];
}
for (int i = 0; i < v.size(); ++i)
{
std::cout << i << ": " << v[i] << "\n";
}
}
code
result:
0: 199875
1: 199642
2: 149852
3: 149789
4: 150237
5: 150605
In this case we observe that numbers in range [ 0, p] = [ 0, 1] appears not about twice as often as the rest but in the ratio of about 20/15. In fact this is 4/3 as our bias formula in this case is
bias = f_0 / f_1 = ( k + 1) / k = 4 / 3
The picture below helps to understand this outcome.
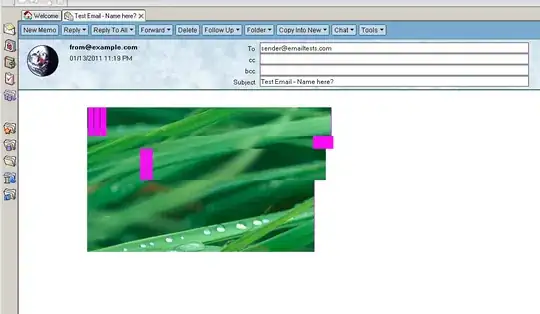
full code