An obvious way to solve this problem is to iterate through all string lengths
in some predetermined order, update width of the column where each string
belongs, and stop when sum of column widths exceeds terminal width. Then repeat
this process for decremented number of columns (or for incremented number of rows for
"arrange down" case) until success. Three possible choices for this predetermined
order:
- by row (consider all strings in the first row, then in row 2, 3, ...)
- by column (consider all strings in the first column, then in column 2, 3, ...)
- by value (sort strings by length, then iterate them in sorted order, starting from the longest one).
These 3 approaches are OK for "arrange across" sub-problem. But for "arrange down"
sub-problem they all have worst case time complexity O(n2). And first two
methods show quadratic complexity even for random data. "By value" approach is pretty
good for random data, but it is easy to find worst case scenario: just assign short
strings to first half of the list and long strings - to second half.
Here I describe an algorithm for "arrange down" case that does not have these
disadvantages.
Instead of inspecting each string length separately, it determines width of each
column in O(1) time with the help of range maximum query (RMQ). Width of the first
column is just the maximum value in range (0 .. num_of_rows), width of next one is
the maximum value in range (num_of_rows .. 2*num_of_rows), etc.
And to answer each query in O(1) time, we need to prepare an array of maximum
values in ranges (0 .. 2k), (1 .. 1 + 2k), ..., where k is
the largest integer such that 2k is not greater than current number of rows. Each range maximum query is computed as maximum of two entries from this array.
When number of rows starts to be too large, we should update this query array from k to k+1 (each such update needs O(n) range queries).
Here is C++14 implementation:
template<class PP>
uint32_t arrangeDownRMQ(Vec& input)
{
auto rows = getMinRows(input);
auto ranges = PP{}(input, rows);
while (rows <= input.size())
{
if (rows >= ranges * 2)
{ // lazily update RMQ data
transform(begin(input) + ranges, end(input), begin(input),
begin(input), maximum
);
ranges *= 2;
}
else
{ // use RMQ to get widths of columns and check if all columns fit
uint32_t sum = 0;
for (Index i = 0; sum <= kPageW && i < input.size(); i += rows)
{
++g_complexity;
auto j = i + rows - ranges;
if (j < input.size())
sum += max(input[i], input[j]);
else
sum += input[i];
}
if (sum <= kPageW)
{
return uint32_t(ceilDiv(input.size(), rows));
}
++rows;
}
}
return 0;
}
Here PP
is optional, for simple case this function object does nothing and
returns 1.
To determine the worst case time complexity of this algorithm, note that outer loop starts
with rows = n * v / m
(where v
is average string length, m
is page width) and
stops with at most rows = n * w / m
(where w
is largest string length).
Number of iterations in "query" loop is not greater than the number of columns or
n / rows
. Adding these iterations together gives O(n * (ln(n*w/m) - ln(n*v/m)))
or O(n * log(w/v))
. Which means linear time with small constant factor.
We should add here time to perform all update operations which is O(n log n) to get
complexity of whole algorithm: O(n * log n).
If we perform no update operations until some query operations are done, time needed
for update operations as well as algorithm complexity decreases to O(n * log(w/v))
.
To make this possible we need some algorithm that fills RMQ array with maximums
of sub-arrays of given length. I tried two possible approaches and it seems
algorithm with pair of stacks
is slightly faster. Here is C++14 implementation (pieces of input array are used to implement both stacks to lower memory requirements and to simplify the code):
template<typename I, typename Op>
auto transform_partial_sum(I lbegin, I lend, I rbegin, I out, Op op)
{ // maximum of the element in first enterval and prefix of second interval
auto sum = typename I::value_type{};
for (; lbegin != lend; ++lbegin, ++rbegin, ++out)
{
sum = op(sum, *rbegin);
*lbegin = op(*lbegin, sum);
}
return sum;
}
template<typename I>
void reverse_max(I b, I e)
{ // for each element: maximum of the suffix starting from this element
partial_sum(make_reverse_iterator(e),
make_reverse_iterator(b),
make_reverse_iterator(e),
maximum);
}
struct PreprocRMQ
{
Index operator () (Vec& input, Index rows)
{
if (rows < 4)
{ // no preprocessing needed
return 1;
}
Index ranges = 1;
auto b = begin(input);
while (rows >>= 1)
{
ranges <<= 1;
}
for (; b + 2 * ranges <= end(input); b += ranges)
{
reverse_max(b, b + ranges);
transform_partial_sum(b, b + ranges, b + ranges, b, maximum);
}
// preprocess inconvenient tail part of the array
reverse_max(b, b + ranges);
const auto d = end(input) - b - ranges;
const auto z = transform_partial_sum(b, b + d, b + ranges, b, maximum);
transform(b + d, b + ranges, b + d, [&](Data x){return max(x, z);});
reverse_max(b + ranges, end(input));
return ranges;
}
};
In practice there is much higher chance to see a short word than a long word.
Shorter words outnumber longer ones in English texts, shorter text representations
of natural numbers prevail as well. So I chosen (slightly modified) geometric distribution of string
lengths to evaluate various algorithms. Here is whole benchmarking program (in
C++14 for gcc). Older version of the same program contains some obsolete tests and different implementation of some algorithms: TL;DR. And here are the results:
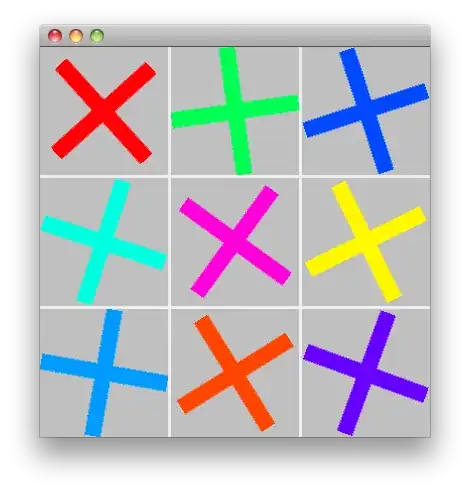
For "arrange across" case:
- "by column" approach is the slowest
- "by value" approach is good for n = 1000..500'000'000
- "by row" approach is better for small and very large sizes
For "arrange down" case:
- "by value" approach is OK, but slower than other alternatives (one possibility to make it faster is to implement divisions via multiplications), also it uses more memory and has quadratic worst-case time
- simple RMQ approach is better
- RMQ with preprocessing the best: it has linear time complexity and its memory bandwidth requirements are pretty low.
It may be also interesting to see number of iterations needed for each algorithm.
Here all predictable parts are excluded (sorting, summing, preprocessing, and RMQ
updates):
