This is a not trivial problem and there are many possible solutions.
Note: I haven't used imgradientxy
and imgradient
as in your code because my matlab version is too old (R2011b).
In my opinion, there are at least two necessary things to do:
Personally I first execute a phase of imerode
/imdilate
operations to slice the initial objects.
I think it's better to erase sub-objects too small:
- with an additional erosion passage, using a small struct-element
bwareaopen
with a small percentage of the total area of the initial object.
If any sub-objects still exists, should be possible to better separate the sub-objects with one of the technique listed above.
I use math morphology (erosion is a little slow), Otsu segmentation again, image difference and eventually bwselect
to control the result.
Here my code and output.
clear all;
close all;
clc;
n_level = 4;
channel_n = 2;
R1_perc = 1 / 8;
R2_perc = 5 / 100;
area2_perc = 2 / 100;
R3_small = 1;
I = imread('~/Downloads/25443957.jpg');
I_hsv = rgb2hsv(I);
% % indentify image background as the object with greatest area
% original Otsu implementation
Ihsv_otsu = otsu(I_hsv, n_level);
areas_extension = zeros(1, n_level);
for x = 1:n_level
img_area_object = Ihsv_otsu == x; % img_zona = (Ihsv_otsu_bwl==n);
areas_extension(1, x) = bwarea(img_area_object);
end
[background_val, background_idx] = max(areas_extension);
Ihsv_otsu_filled = imfill(Ihsv_otsu ~= background_idx, 'holes');
% % divide et impera
% analyze the objects one by one
Ihsv_otsu_bwl = bwlabel(Ihsv_otsu_filled);
img_morph = zeros(size(Ihsv_otsu_bwl));
img_eroded = zeros(size(Ihsv_otsu_bwl));
SE1px1 = strel('disk', 1);
SE3small = strel('disk', R3_small);
fprintf('start loop MORPH, Operations:: erosion & dilate...\n')
for n = 1:max(unique(Ihsv_otsu_bwl))
fprintf('morph loop... iter:%d\n', n)
img_zona = Ihsv_otsu_bwl == n;
temp = regionprops(img_zona, 'MinorAxisLength');
minoraxis = temp.MinorAxisLength;
img_zona_out = img_zona;
R = ceil(minoraxis * R1_perc); % use 'ceil', not 'round', to avoid R<1 processing small regions
SE2R1 = strel('disk', R);
CC = bwconncomp(img_zona_out, 8);
k = 0;
while CC.NumObjects == 1
img_zona_out = imerode(img_zona_out, SE2R1);
CC = bwconncomp(img_zona_out, 8);
k = k + 1;
% %% it is possible to erode with size~1-pixel object or not
% if CC.NumObjects>1
% fprintf('FOUND! iter:%d, c_EROSION:%d, n_Obj:%d\n', n, k, CC.NumObjects)
% elseif CC.NumObjects==0
% fprintf('BREAK! iter:%d, c_EROSION:%d, n_Obj:%d\n', n, k, CC.NumObjects)
% break
% %elseif CC.NumObjects==1
% % fprintf('keep carry on... iter:%d, c_EROSION:%d, n_Obj:%d\n', n, k, CC.NumObjects)
% end
if CC.NumObjects > 1
img_zona_out = imerode(img_zona_out, SE3small);
CC = bwconncomp(img_zona_out, 8);
if CC.NumObjects > 1
fprintf('FOUND! iter:%d, c_EROSION:%d, n_Obj:%d\n', n, k, CC.NumObjects)
elseif CC.NumObjects == 0
% fprintf('BREAK! iter:%d, c_EROSION:%d, n_Obj:%d\n', n, k, CC.NumObjects)
break
end
end
end
% %% post-erosion:
% if the object number is 0, drop the eroded image and mantain the pre-eroded image
if CC.NumObjects == 0
img_morph = imadd(img_morph > 0, img_zona);
img_eroded = imadd(img_eroded > 0, img_zona);
continue
% if the object number is greater than 1, dilate the objects until
% they touch, when the CC.NumObjects is 1
elseif CC.NumObjects > 1
k = 0;
img_zona_dilate = img_zona_out;
while CC.NumObjects > 1
k = k + 1;
img_zona_old = img_zona_dilate;
% a small radius is better for a uniform expansion
img_zona_dilate = imdilate(img_zona_dilate, SE3small);
CC = bwconncomp(img_zona_dilate > 0, 8);
if CC.NumObjects == 1
% %% results the last objects immediatly before they touch
img_eroded = imadd(img_eroded > 0, img_zona_old);
fprintf('UNITED! iter:%d, c_DILATE:%d, n_n_Obj:%d\n\n', n, k, CC.NumObjects)
end
end
% modified Otsu function (otsuSeparation.m)
img_splitted = otsuSeparation(I_hsv, img_zona, channel_n, R2_perc, area2_perc);
img_morph = imadd(img_morph > 0, img_splitted > 0);
elseif CC.NumObjects == 1
fprintf('# only one object... strange at this point.\n#')
fprintf('# iter:%d, c_DILATE:%d, CC.NumObjects:%g\n', n, k, CC.NumObjects)
end
end
% %
fprintf('start loop BWSELECT:: img_morph & img_eroded...\n')
img_eroded_bwl = bwlabel(img_eroded);
img_result = zeros(size(img_eroded_bwl));
for X = 1:max(unique(img_eroded_bwl))
fprintf('# BWSELECT, iter:%d\n', X)
obj2select = img_eroded_bwl == X;
centr = regionprops(obj2select, 'centroid');
xc = round(centr.Centroid(1));
yc = round(centr.Centroid(2));
temp = bwselect(img_morph, xc, yc);
img_result = imadd(img_result > 0, temp);
end
img_result = img_result > 0;
%%
close all
figure('Name', 'morph', 'NumberTitle', 'off', 'WindowStyle', 'docked');
imagesc(img_morph)
figure('Name', 'erodeed', 'NumberTitle', 'off', 'WindowStyle', 'docked');
imagesc(img_eroded)
figure('Name', 'hsv', 'NumberTitle', 'off', 'WindowStyle', 'docked');
imagesc(I_hsv)
figure('Name', 'image', 'NumberTitle', 'off', 'WindowStyle', 'docked');
imagesc(I)
figure('Name', 'result', 'NumberTitle', 'off', 'WindowStyle', 'docked');
imagesc(img_result)
Here my modification of the Otsu function, OtsuSeparation.m
.
function [img_splitted] = otsuSeparation(I, bw, channel_n, R2_perc, area2_perc)
img_splitted = zeros(size(bw));
SE1px1 = strel('disk', 1);
% fprintf('start loop: Otsu segmentation...\n')
% for Y=1:max(unique(img_bwl)) %for Y=41:41
% fprintf('# OtsuSep: iter:%d\n', Y)
% bw = img_bwl==Y;
img_channel = I(:, :, channel_n);
img_channel(bw == 0) = 0;
% image segmentation by intensity
img_channel_otsu = otsu(img_channel);
% processing "BW" to avoid multiple objects and use only one valor for MinorAxisLength
temp2 = regionprops(bw, 'MinorAxisLength', 'Area');
R2 = ceil(temp2.MinorAxisLength * R2_perc);
area2 = ceil(temp2.Area * area2_perc);
SE3R2 = strel('disk', R2);
% %% PART1: cleaning principal object selected by Otsu segmentation
% removing small objects external to main selection with bwareaopen to
% avoid modification at object contours
img_part1 = bwareaopen(img_channel_otsu > 1, area2, 8);
img_part1 = imfill(img_part1, 'holes');
% execting imdilate to avoid intersection between PART1 and PART2 complements
img_part1 = imdilate(img_part1, SE3R2);
img_complement1 = imcomplement(img_part1);
% %% PART2: cleaning external area around PART1 object
% execting imerode to avoid intersection between PART1 and PART2 complements
img_bw_eroded = imerode(bw, SE3R2);
img_complement2 = imcomplement(img_bw_eroded);
img_part2 = img_complement1 - img_complement2;
img_part2 = bwareaopen(img_part2 > 0, area2, 8);
% dilate (after erosion) to restore original object size and morphology
img_part2 = imdilate(img_part2, SE3R2);
% securing a well-done separation between objects
img_intersez = imadd(img_part1, img_part2) > 1;
img_intersez = imdilate(img_intersez, SE1px1);
% use manual substraction here instead of imabsdiff for a good separation
img_part1 = (img_part1 - img_intersez) > 0;
img_part2 = (img_part2 - img_intersez) > 0;
img_splitted = imadd(img_splitted, imadd(img_part1, img_part2));
Of course it's possible perform different choices.
To improve the accuracy, it could be necessary try the algorithm with few more sample image and test it with a control group of different images.
Here my output.
Grayscale bwlabel (color version):
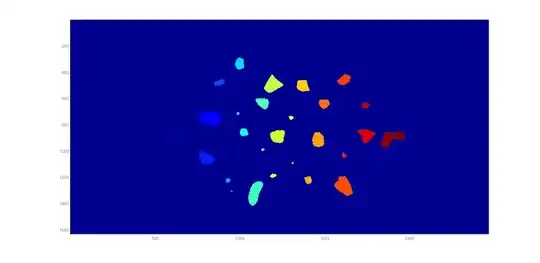
Grayscale bwlabel (matlab raw image):
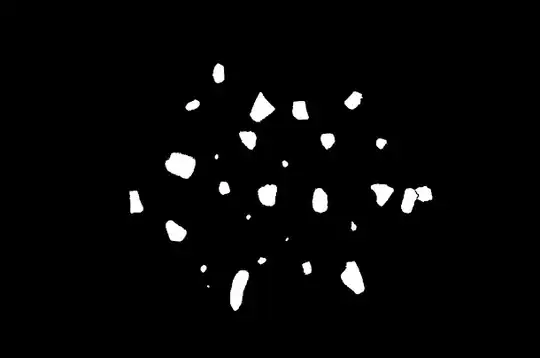
UPDATE:
My code needs an improvement. During the "imdilate after imerode" phase, I assume that the binary object should be divided into two parts. To emprove the algorithm, It's possible to use also edge detection:
http://robotics.eecs.berkeley.edu/~sastry/ee20/index.html
http://it.mathworks.com/help/coder/examples/edge-detection-on-images.html
http://www.mathworks.com/matlabcentral/fileexchange/45459-canny-edge-detection-in-2-d-and-3-d
Other generic resources about image segmentation:
https://en.wikipedia.org/wiki/Outline_of_object_recognition
http://it.mathworks.com/help/images/object-analysis.html
http://it.mathworks.com/help/images/texture-analysis-1.html
http://it.mathworks.com/help/images/image-segmentation-1.html
As alternative, you could use this approach (example in python) using connected components with stats:
Alternative segmentation techniques other than watershed for soil particles in images
UPDATE2: You could also change approach using deep learning, like in these examples:
UPDATE3: I think that segment-anything deserves a special mention because of its effectiveness. Here my result with the question image: note that segment-anything separates correctly the two overlapping stones on the right but doesn't find some small other ones
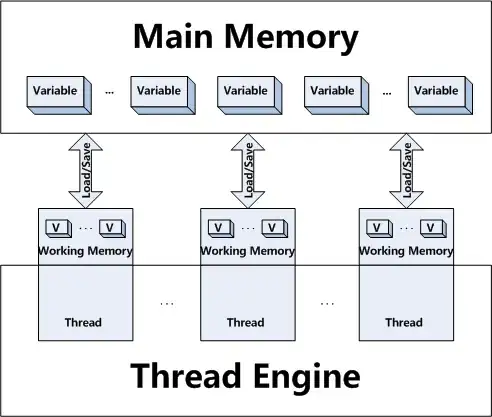