disclaimer - I am not an expert on Krigging. Krigging is complex and takes a good understanding of the underlying data, the method and the purpose to achieve the correct result. You may wish to try to get input from @whuber [on the GIS Stack Exchange or contact him through his website (http://www.quantdec.com/quals/quals.htm)] or another expert you know.
That said, if you just want to achieve the visual effect you requested and are not using this for some sort of statistical analysis, I think there are some relatively simple solutions.
EDIT:
As you commented, though the suggestions below to use theta
and smoothness
arguments do even out the prediction surface, they apply equally to all measurements and thus do not extend the "sphere of influence" of more densely populated counties relative to less-densely populated. After further consideration, I think there are two ways to achieve this: by altering the covariance function to depend on population density or by using weights, as you have. Your weighting approach, as I wrote below, alters the error term of the krigging function. That is, it inversely scales the nugget variance.
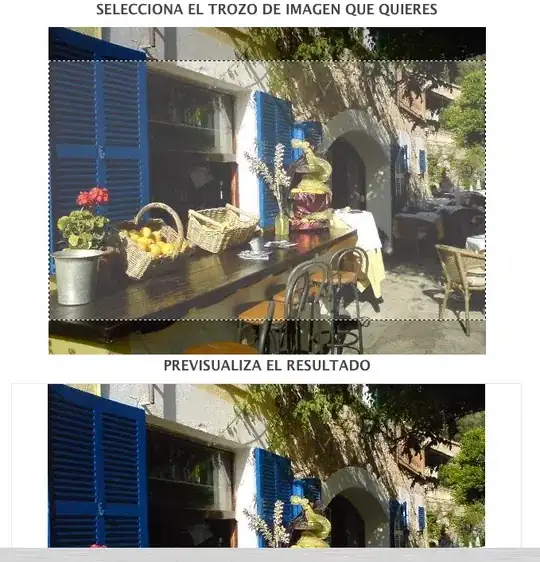
As you can see in the semivariogram image, the nugget is essentially the y-intercept, or the error between measurements at the same location. Weights affect the nugget variance (sigma2) as sigma2/weight. Thus, greater weights mean less error at small-scale distances. This does not, however, change the shape of the semivariance function or have much effect on the range or sill.
I think that the best solution would be to have your covariance function depend on population. however, I'm not sure how to accomplish that and I don't see any arguments to Krig
to do so. I tried playing with defining my own covariance function as in the Krig
example, but only got errors.
Sorry I couldn't help more!
Another great resource to help understand Krigging is: http://www.epa.gov/airtrends/specialstudies/dsisurfaces.pdf
As I said in my comment, the sill and nugget values as well as the range of the semivariogram are things you can alter to affect the smoothing. By specifying weights
in the call to Krig
, you are altering the variance of the measurement errors. That is, in a normal use, weights are expected to be proportional to the accuracy of the measurement value so that higher weights represent more accurate measurements, essentially. This isn't actually true with your data, but it may be giving you the effect you desire.
To alter the way your data is interpolated, you can adjust two (and many more) parameters in the simple Krig
call you are using: theta
and smoothness
. theta
adjusts the semivariance range, meaning that measured points farther away contribute more to the estimates as you increase theta
. Your data range is
range <- data.frame(lon=range(ct.data$lon),lat=range(ct.data$lat))
range[2,]-range[1,]
lon lat
2 1.383717 0.6300484
so, your measurement points vary by ~1.4 degrees lon and ~0.6 degrees lat. Thus, you can play with specifying your theta
value in that range to see how that affects your result. In general, a larger theta
leads to more smoothing since you are drawing from more values for each prediction.
Krig.output.wt <- Krig( cbind(ct.data$lon,ct.data$lat) , ct.data$county.poverty.rate ,
weights=c( size , 1 , 1 , 1 , 1 , size , size , 1 ),Covariance="Matern", theta=.8)
r <- interpolate(ras, Krig.output.wt)
r <- mask(r, ct.map)
plot(r, col=colRamp(100) ,axes=FALSE,legend=FALSE)
title(main="Theta = 0.8", outer = FALSE)
points(cbind(ct.data$lon,ct.data$lat))
text(ct.data$lon, ct.data$lat-0.05, ct.data$NAME, cex=0.5)
Gives:
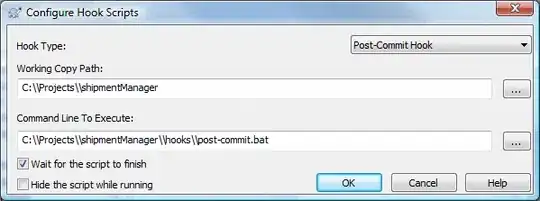
Krig.output.wt <- Krig( cbind(ct.data$lon,ct.data$lat) , ct.data$county.poverty.rate ,
weights=c( size , 1 , 1 , 1 , 1 , size , size , 1 ),Covariance="Matern", theta=1.6)
r <- interpolate(ras, Krig.output.wt)
r <- mask(r, ct.map)
plot(r, col=colRamp(100) ,axes=FALSE,legend=FALSE)
title(main="Theta = 1.6", outer = FALSE)
points(cbind(ct.data$lon,ct.data$lat))
text(ct.data$lon, ct.data$lat-0.05, ct.data$NAME, cex=0.5)
Gives:
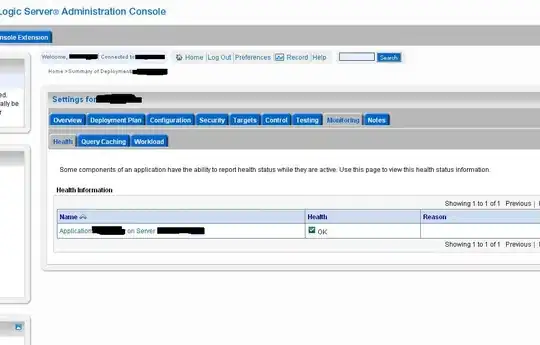
Adding the smoothness
argument, will change the order of the function used to smooth your predictions. The default is 0.5 leading to a second-order polynomial.
Krig.output.wt <- Krig( cbind(ct.data$lon,ct.data$lat) , ct.data$county.poverty.rate ,
weights=c( size , 1 , 1 , 1 , 1 , size , size , 1 ),
Covariance="Matern", smoothness = 0.6)
r <- interpolate(ras, Krig.output.wt)
r <- mask(r, ct.map)
plot(r, col=colRamp(100) ,axes=FALSE,legend=FALSE)
title(main="Theta unspecified; Smoothness = 0.6", outer = FALSE)
points(cbind(ct.data$lon,ct.data$lat))
text(ct.data$lon, ct.data$lat-0.05, ct.data$NAME, cex=0.5)
Gives:
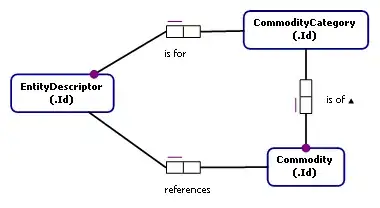
This should give you a start and some options, but you should look at the manual for fields
. It is pretty well-written and explains the arguments well.
Also, if this is in any way quantitative, I would highly recommend talking to someone with significant spatial statistics know how!