Updated Answer
Actually, if you use Connected Components Analysis, a.k.a Blob Analysis, you can do it with ImageMagick much more succinctly and accurately like this:
convert 3J3qz.jpg \
-define connected-components:verbose=true \
-define connected-components:area-threshold=100 \
-connected-components 8 null:
Output:
Objects (id: bounding-box centroid area mean-color):
0: 720x576+0+0 370.6,322.1 213779 srgb(0,0,0)
13: 488x513+104+0 347.7,250.7 200941 srgb(255,255,255) <-- answer
which shows your largest blob (the speech bubble) has its centroid at coordinates 347,250 from the top-left corner, and also gives you the bounding box which measures 488x513 pixels and its top-left corner is at 104,0 from which you can derive a radius.
I can mark these with ImageMagick like this:
convert 3J3qz.jpg \
-fill red -draw "rectangle 342,245 352,255"
-stroke red -fill none -draw "rectangle 104,0 592,513"
out.png
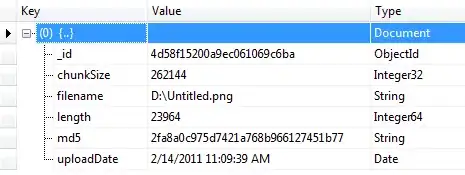
Original Answer
As you are curious... you can do what I was suggesting with ImageMagick in two lines:
convert 3J3qz.jpg -resize 1x! -colorspace gray txt:
# ImageMagick pixel enumeration: 1,576,255,gray
0,0: (66,66,66) #424242 gray(66)
0,1: (70,70,70) #464646 gray(70)
0,2: (72,72,72) #484848 gray(72)
0,3: (76,76,76) #4C4C4C gray(76)
...
0,152: (176,176,176) #B0B0B0 gray(176)
0,153: (176,176,176) #B0B0B0 gray(176)
0,154: (177,177,177) #B1B1B1 gray(177)
0,155: (177,177,177) #B1B1B1 gray(177)
0,156: (177,177,177) #B1B1B1 gray(177)
0,157: (177,177,177) #B1B1B1 gray(177)
0,158: (178,178,178) #B2B2B2 gray(178)
0,159: (178,178,178) #B2B2B2 gray(178)
0,160: (179,179,179) #B3B3B3 gray(179)
0,161: (179,179,179) #B3B3B3 gray(179)
0,162: (179,179,179) #B3B3B3 gray(179)
0,163: (179,179,179) #B3B3B3 gray(179)
0,164: (179,179,179) #B3B3B3 gray(179)
0,165: (179,179,179) #B3B3B3 gray(179)
0,166: (179,179,179) #B3B3B3 gray(179)
0,167: (179,179,179) #B3B3B3 gray(179)
0,168: (180,180,180) #B4B4B4 gray(180)
0,169: (180,180,180) #B4B4B4 gray(180)
0,170: (180,180,180) #B4B4B4 gray(180)
0,171: (180,180,180) #B4B4B4 gray(180)
0,172: (180,180,180) #B4B4B4 gray(180)
0,173: (180,180,180) #B4B4B4 gray(180)
0,174: (180,180,180) #B4B4B4 gray(180)
0,175: (180,180,180) #B4B4B4 gray(180)
0,176: (181,181,181) #B5B5B5 gray(181)
0,177: (181,181,181) #B5B5B5 gray(181)
0,178: (182,182,182) #B6B6B6 gray(182)
0,179: (182,182,182) #B6B6B6 gray(182)
0,180: (182,182,182) #B6B6B6 gray(182)
0,181: (182,182,182) #B6B6B6 gray(182)
0,182: (182,182,182) #B6B6B6 gray(182)
0,183: (182,182,182) #B6B6B6 gray(182)
0,184: (183,183,183) #B7B7B7 gray(183)
0,185: (183,183,183) #B7B7B7 gray(183)
0,186: (183,183,183) #B7B7B7 gray(183)
0,187: (183,183,183) #B7B7B7 gray(183)
0,188: (183,183,183) #B7B7B7 gray(183)
0,189: (183,183,183) #B7B7B7 gray(183)
0,190: (183,183,183) #B7B7B7 gray(183)
0,191: (183,183,183) #B7B7B7 gray(183)
0,192: (184,184,184) #B8B8B8 gray(184)
0,193: (184,184,184) #B8B8B8 gray(184)
0,194: (184,184,184) #B8B8B8 gray(184)
0,195: (184,184,184) #B8B8B8 gray(184)
0,196: (184,184,184) #B8B8B8 gray(184)
0,197: (184,184,184) #B8B8B8 gray(184)
0,198: (184,184,184) #B8B8B8 gray(184)
0,199: (184,184,184) #B8B8B8 gray(184)
0,200: (185,185,185) #B9B9B9 gray(185)
0,201: (185,185,185) #B9B9B9 gray(185)
0,202: (185,185,185) #B9B9B9 gray(185)
0,203: (185,185,185) #B9B9B9 gray(185)
0,204: (185,185,185) #B9B9B9 gray(185)
0,205: (185,185,185) #B9B9B9 gray(185)
0,206: (185,185,185) #B9B9B9 gray(185)
0,207: (185,185,185) #B9B9B9 gray(185)
0,208: (186,186,186) #BABABA gray(186)
0,209: (186,186,186) #BABABA gray(186)
0,210: (186,186,186) #BABABA gray(186)
0,211: (186,186,186) #BABABA gray(186)
0,212: (185,185,185) #B9B9B9 gray(185)
0,213: (186,186,186) #BABABA gray(186)
0,214: (186,186,186) #BABABA gray(186)
0,215: (186,186,186) #BABABA gray(186)
0,216: (186,186,186) #BABABA gray(186)
0,217: (186,186,186) #BABABA gray(186)
0,218: (186,186,186) #BABABA gray(186)
0,219: (186,186,186) #BABABA gray(186)
0,220: (186,186,186) #BABABA gray(186)
0,221: (186,186,186) #BABABA gray(186)
0,222: (186,186,186) #BABABA gray(186)
0,223: (186,186,186) #BABABA gray(186)
0,224: (186,186,186) #BABABA gray(186)
0,225: (186,186,186) #BABABA gray(186)
0,226: (186,186,186) #BABABA gray(186)
0,227: (186,186,186) #BABABA gray(186)
0,228: (187,187,187) #BBBBBB gray(187)
0,229: (187,187,187) #BBBBBB gray(187)
0,230: (187,187,187) #BBBBBB gray(187)
0,231: (187,187,187) #BBBBBB gray(187)
0,232: (187,187,187) #BBBBBB gray(187)
0,233: (187,187,187) #BBBBBB gray(187)
0,234: (187,187,187) #BBBBBB gray(187) <---- max=234
0,235: (187,187,187) #BBBBBB gray(187)
0,236: (187,187,187) #BBBBBB gray(187)
0,237: (187,187,187) #BBBBBB gray(187)
0,238: (187,187,187) #BBBBBB gray(187)
0,239: (187,187,187) #BBBBBB gray(187)
0,240: (187,187,187) #BBBBBB gray(187)
0,241: (187,187,187) #BBBBBB gray(187)
0,242: (187,187,187) #BBBBBB gray(187)
0,243: (187,187,187) #BBBBBB gray(187)
0,244: (187,187,187) #BBBBBB gray(187)
0,245: (187,187,187) #BBBBBB gray(187)
0,246: (187,187,187) #BBBBBB gray(187)
0,247: (187,187,187) #BBBBBB gray(187)
0,248: (187,187,187) #BBBBBB gray(187)
0,249: (187,187,187) #BBBBBB gray(187)
0,250: (187,187,187) #BBBBBB gray(187)
...
0,573: (0,0,0) #000000 gray(0)
0,574: (0,0,0) #000000 gray(0)
0,575: (0,0,0) #000000 gray(0)
And the other side
convert 3J3qz.jpg -resize x1! -colorspace gray txt:
# ImageMagick pixel enumeration: 720,1,255,gray
0,0: (0,0,0) #000000 gray(0)
1,0: (0,0,0) #000000 gray(0)
2,0: (0,0,0) #000000 gray(0)
3,0: (0,0,0) #000000 gray(0)
4,0: (0,0,0) #000000 gray(0)
...
241,0: (219,219,219) #DBDBDB gray(219)
242,0: (220,220,220) #DCDCDC gray(220)
243,0: (220,220,220) #DCDCDC gray(220)
244,0: (221,221,221) #DDDDDD gray(221)
245,0: (222,222,222) #DEDEDE gray(222)
246,0: (223,223,223) #DFDFDF gray(223)
247,0: (223,223,223) #DFDFDF gray(223)
248,0: (224,224,224) #E0E0E0 gray(224)
249,0: (224,224,224) #E0E0E0 gray(224)
250,0: (225,225,225) #E1E1E1 gray(225)
251,0: (227,227,227) #E3E3E3 gray(227)
252,0: (229,229,229) #E5E5E5 gray(229)
253,0: (230,230,230) #E6E6E6 gray(230)
254,0: (231,231,231) #E7E7E7 gray(231)
255,0: (232,232,232) #E8E8E8 gray(232) <--- max=255
256,0: (231,231,231) #E7E7E7 gray(231)
257,0: (231,231,231) #E7E7E7 gray(231)
258,0: (231,231,231) #E7E7E7 gray(231)
259,0: (231,231,231) #E7E7E7 gray(231)
260,0: (230,230,230) #E6E6E6 gray(230)
261,0: (230,230,230) #E6E6E6 gray(230)
262,0: (230,230,230) #E6E6E6 gray(230)
263,0: (230,230,230) #E6E6E6 gray(230)
264,0: (230,230,230) #E6E6E6 gray(230)
265,0: (230,230,230) #E6E6E6 gray(230)
266,0: (230,230,230) #E6E6E6 gray(230)
267,0: (230,230,230) #E6E6E6 gray(230)
268,0: (229,229,229) #E5E5E5 gray(229)
269,0: (230,230,230) #E6E6E6 gray(230)
270,0: (229,229,229) #E5E5E5 gray(229)
271,0: (229,229,229) #E5E5E5 gray(229)
272,0: (229,229,229) #E5E5E5 gray(229)
273,0: (229,229,229) #E5E5E5 gray(229)
274,0: (229,229,229) #E5E5E5 gray(229)
275,0: (229,229,229) #E5E5E5 gray(229)
276,0: (229,229,229) #E5E5E5 gray(229)
277,0: (229,229,229) #E5E5E5 gray(229)
278,0: (229,229,229) #E5E5E5 gray(229)
279,0: (229,229,229) #E5E5E5 gray(229)
280,0: (229,229,229) #E5E5E5 gray(229)
281,0: (229,229,229) #E5E5E5 gray(229)
282,0: (229,229,229) #E5E5E5 gray(229)
283,0: (229,229,229) #E5E5E5 gray(229)
284,0: (229,229,229) #E5E5E5 gray(229)
285,0: (229,229,229) #E5E5E5 gray(229)
286,0: (229,229,229) #E5E5E5 gray(229)
287,0: (230,230,230) #E6E6E6 gray(230)
288,0: (230,230,230) #E6E6E6 gray(230)
289,0: (230,230,230) #E6E6E6 gray(230)
290,0: (230,230,230) #E6E6E6 gray(230)
291,0: (230,230,230) #E6E6E6 gray(230)
292,0: (230,230,230) #E6E6E6 gray(230)
293,0: (230,230,230) #E6E6E6 gray(230)
294,0: (230,230,230) #E6E6E6 gray(230)
295,0: (231,231,231) #E7E7E7 gray(231)
296,0: (231,231,231) #E7E7E7 gray(231)
297,0: (231,231,231) #E7E7E7 gray(231)
298,0: (231,231,231) #E7E7E7 gray(231)
299,0: (231,231,231) #E7E7E7 gray(231)
300,0: (231,231,231) #E7E7E7 gray(231)
301,0: (231,231,231) #E7E7E7 gray(231)
302,0: (231,231,231) #E7E7E7 gray(231)
303,0: (231,231,231) #E7E7E7 gray(231)
304,0: (232,232,232) #E8E8E8 gray(232)
305,0: (231,231,231) #E7E7E7 gray(231)
306,0: (231,231,231) #E7E7E7 gray(231)
307,0: (231,231,231) #E7E7E7 gray(231)
308,0: (231,231,231) #E7E7E7 gray(231)
309,0: (232,232,232) #E8E8E8 gray(232)
310,0: (232,232,232) #E8E8E8 gray(232)
311,0: (232,232,232) #E8E8E8 gray(232)
312,0: (233,233,233) #E9E9E9 gray(233)
313,0: (232,232,232) #E8E8E8 gray(232)
314,0: (232,232,232) #E8E8E8 gray(232)
315,0: (232,232,232) #E8E8E8 gray(232)
316,0: (232,232,232) #E8E8E8 gray(232)
317,0: (232,232,232) #E8E8E8 gray(232)
318,0: (232,232,232) #E8E8E8 gray(232)
319,0: (232,232,232) #E8E8E8 gray(232)
320,0: (232,232,232) #E8E8E8 gray(232)
321,0: (233,233,233) #E9E9E9 gray(233)
322,0: (233,233,233) #E9E9E9 gray(233)
323,0: (233,233,233) #E9E9E9 gray(233)
324,0: (233,233,233) #E9E9E9 gray(233)
325,0: (233,233,233) #E9E9E9 gray(233)
326,0: (233,233,233) #E9E9E9 gray(233)
327,0: (233,233,233) #E9E9E9 gray(233)
328,0: (233,233,233) #E9E9E9 gray(233)
329,0: (233,233,233) #E9E9E9 gray(233)
330,0: (233,233,233) #E9E9E9 gray(233)
331,0: (233,233,233) #E9E9E9 gray(233)
332,0: (233,233,233) #E9E9E9 gray(233)
333,0: (233,233,233) #E9E9E9 gray(233)
334,0: (233,233,233) #E9E9E9 gray(233)
335,0: (233,233,233) #E9E9E9 gray(233)
336,0: (233,233,233) #E9E9E9 gray(233)
337,0: (233,233,233) #E9E9E9 gray(233)
338,0: (233,233,233) #E9E9E9 gray(233)
339,0: (233,233,233) #E9E9E9 gray(233)
340,0: (233,233,233) #E9E9E9 gray(233)
341,0: (233,233,233) #E9E9E9 gray(233)
342,0: (233,233,233) #E9E9E9 gray(233)
343,0: (233,233,233) #E9E9E9 gray(233)
344,0: (233,233,233) #E9E9E9 gray(233)
345,0: (233,233,233) #E9E9E9 gray(233)
346,0: (233,233,233) #E9E9E9 gray(233)
347,0: (233,233,233) #E9E9E9 gray(233)
348,0: (233,233,233) #E9E9E9 gray(233)
349,0: (233,233,233) #E9E9E9 gray(233)
350,0: (233,233,233) #E9E9E9 gray(233)
351,0: (233,233,233) #E9E9E9 gray(233)
352,0: (233,233,233) #E9E9E9 gray(233)
353,0: (233,233,233) #E9E9E9 gray(233)
354,0: (233,233,233) #E9E9E9 gray(233)
...
717,0: (0,0,0) #000000 gray(0)
718,0: (0,0,0) #000000 gray(0)
719,0: (0,0,0) #000000 gray(0)