I think this is indeed not very friendly. I will write here the code and explain why it works.
The equation of a multivariate gaussian is as follows:
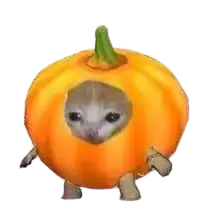
In the 2D case,
and
are 2D column vectors,
is a 2x2 covariance matrix and n=2.
So in the 2D case, the vector
is actually a point (x,y), for which we want to compute function value, given the 2D mean vector
, which we can also write as (mX, mY), and the covariance matrix
.
To make it more friendly to implement, let's compute the result of
:
So
is the column vector (x - mX, y - mY). Therefore, the result of computing
is the 2D row vector:
(CI[0,0] * (x - mX) + CI[1,0] * (y - mY) , CI[0,1] * (x - mX) + CI[1,1] * (y - mY)), where CI is the inverse of the covariance matrix, shown in the equation as
, which is a 2x2 matrix, like
is.
Then, the current result, which is a 2D row vector, is multiplied (inner product) by the column vector
, which finally gives us the scalar:
CI[0,0](x - mX)^2 + (CI[1,0] + CI[0,1])(y - mY)(x - mX) + CI[1,1](y - mY)^2
This is going to be easier to implement this expression using NumPy, in comparison to
, even though they have the same value.
>>> m = np.array([[0.2],[0.6]]) # defining the mean of the Gaussian (mX = 0.2, mY=0.6)
>>> cov = np.array([[0.7, 0.4], [0.4, 0.25]]) # defining the covariance matrix
>>> cov_inv = np.linalg.inv(cov) # inverse of covariance matrix
>>> cov_det = np.linalg.det(cov) # determinant of covariance matrix
# Plotting
>>> x = np.linspace(-2, 2)
>>> y = np.linspace(-2, 2)
>>> X,Y = np.meshgrid(x,y)
>>> coe = 1.0 / ((2 * np.pi)**2 * cov_det)**0.5
>>> Z = coe * np.e ** (-0.5 * (cov_inv[0,0]*(X-m[0])**2 + (cov_inv[0,1] + cov_inv[1,0])*(X-m[0])*(Y-m[1]) + cov_inv[1,1]*(Y-m[1])**2))
>>> plt.contour(X,Y,Z)
<matplotlib.contour.QuadContourSet object at 0x00000252C55773C8>
>>> plt.show()
The result:
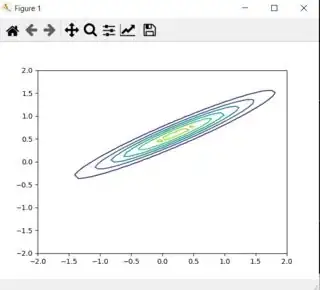