The approach described in this post is more complex than needed for such a question. As you noted, simple sorting by distance will suffice. However, to help explain your confusion about what your sample answer author was trying to get at, maybe consider the k nearest neighbors problem which can be solved with a k-d tree, a structure that applies space partitioning to the k-d dataset. For 2-dimensional space, that is indeed a binary tree. This tree is inherently sorted and doesn't need any "heap sorting."
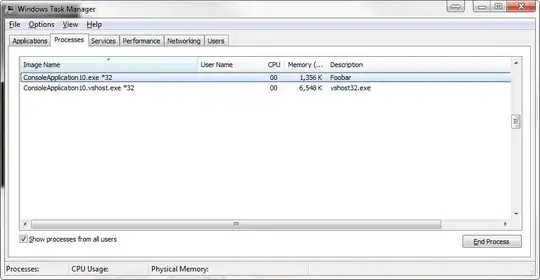
It should be noted that building the k-d tree will take O(n log n), and is only worth the cost if you need to do repeated nearest neighbor searches on the structure. If you only need to perform one search to find k nearest neighbors from the origin, it can be done with a naive O(n) search.
How to build a k-d tree, straight from Wiki:
One adds a new point to a k-d tree in the same way as one adds an element to any other search tree. First, traverse the tree, starting from the root and moving to either the left or the right child depending on whether the point to be inserted is on the "left" or "right" side of the splitting plane. Once you get to the node under which the child should be located, add the new point as either the left or right child of the leaf node, again depending on which side of the node's splitting plane contains the new node.
Adding points in this manner can cause the tree to become unbalanced, leading to decreased tree performance. The rate of tree performance degradation is dependent upon the spatial distribution of tree points being added, and the number of points added in relation to the tree size. If a tree becomes too unbalanced, it may need to be re-balanced to restore the performance of queries that rely on the tree balancing, such as nearest neighbour searching.
Once have have built the tree, you can find k nearest neighbors to some point (the origin in your case) in O(k log n) time.
Straight from Wiki:
Searching for a nearest neighbour in a k-d tree proceeds as follows:
- Starting with the root node, the algorithm moves down the tree recursively, in the same way that it would if the search point were being inserted (i.e. it goes left or right depending on whether the point is lesser than or greater than the current node in the split dimension).
- Once the algorithm reaches a leaf node, it saves that node point as the "current best"
- The algorithm unwinds the recursion of the tree, performing the following steps at each node:
- If the current node is closer than the current best, then it becomes the current best.
- The algorithm checks whether there could be any points on the other side of the splitting plane that are closer to the search point than the current best. In concept, this is done by intersecting the splitting hyperplane with a hypersphere around the search point that has a radius equal to the current nearest distance. Since the hyperplanes are all axis-aligned this is implemented as a simple comparison to see whether the difference between the splitting coordinate of the search point and current node is lesser than the distance (overall coordinates) from the search point to the current best.
- If the hypersphere crosses the plane, there could be nearer points on the other side of the plane, so the algorithm must move down the other branch of the tree from the current node looking for closer points, following the same recursive process as the entire search.
- If the hypersphere doesn't intersect the splitting plane, then the algorithm continues walking up the tree, and the entire branch on the other side of that node is eliminated.
- When the algorithm finishes this process for the root node, then the search is complete.
This is a pretty tricky algorithm that I would hate to need to describe as an interview question! Fortunately the general case here is more complex than is needed, as you pointed out in your post. But I believe this approach may be close to what your (wrong) sample answer was trying to describe.