Introduction & Test Setup
To perform memory tests to inquire about the points raised in the question, let's define the inputs A
and B
:
A = rand(M,N)
B = rand(1,N)
Here, M
and N
are the size parameters and are kept as really large numbers.
I would be using repmat
for comparisons as that seems like the closest alternative to bsxfun
. So, the idea here to run the bsxfun
and repmat
equivalent codes and watch out for the bumps in memory usages from the Task Manager (on Windows).
This solution that compared bsxfun
and repmat
for runtime efficiency led to the conclusions that using relational operations with bsxfun
is hugely runtime efficient, so it would be interesting to extend the basis of memory efficiency
to the comparisons.
Thus, the bsxfun
and repmat
equivalents would look something like these -
REPMAT version: A == repmat(B,size(A,1),1)
BSXFUN version: bsxfun(@eq,A,B))
Results
On running the repmat
and then bsxfun
codes, the Windows Task Manager showed something like this with the first bump denoting the run for repmat
and the next one is for the bsxfun
one -
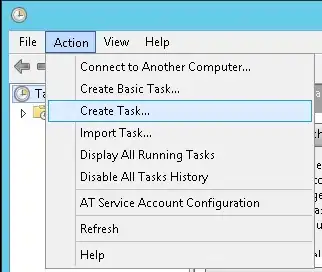
The repmat
bump has the same height as that when an actual copy of A
is created. This basically shows that repmat
makes an actual replication of B
and then does the equality check. Since, B
is to be replicated to a bigger floating point array, the memory requirements are huge as again shown in the memory graph earlier. On the other hand with bsxfun
, from its bump height it seems is not replicating the actual floating point values and that leads to an efficient memory usage.
Now, after converting both A
and B
to logical arrays, the memory usage bumps changed to this -
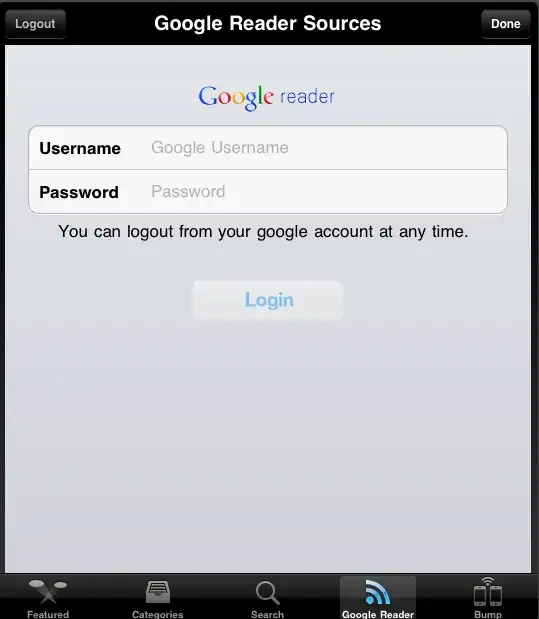
Thus, it suggests that repmat
was then able to optimize memory, as this time the replication was of logical datatype.
Using anonymous functions with bsxfun
: One can experiment a bit with the anonymous functions usage with bsxfun
and see if MATLAB shows the same smartness with it in optimizing memory requirements as with the built-in.
So, bsxfun(@eq,A,B)
could be replaced by bsxfun(@(k1,k2) k1==k2,A,B)
. The resultant memory usage with this built-in and anonymous function implementation when operated on floating point input arrays, resulted in a memory graph as shown below -
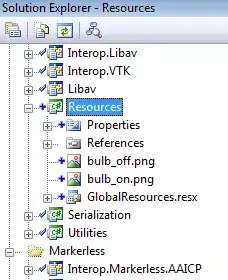
The plot indicates that the use of anonymous function keeps the memory efficiency as with the built-in, even though the runtime is hampered quite a bit. The test results were similar when other relational operations were used instead.
Conclusions
When working with relational operations on floating-point arrays, it's definitely preferable to use bsxfun
over repmat
for both runtime and memory efficiency. So, this just proves that there are more reasons to go with bsxfun
!