update 3
Here's a concrete example of what I describe in update 2. If you don't have mayavi
for visualization, I suggest installing it via edm using edm install mayavi pyqt matplotlib
.
Toy 2D contours stacked in 3D

Contours -> 3D surface
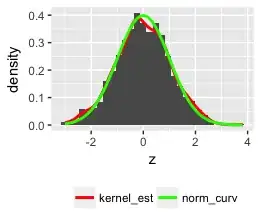
Code to generate the figures
from matplotlib import path as mpath
from mayavi import mlab
import numpy as np
def make_star(amplitude=1.0, rotation=0.0):
""" Make a star shape
"""
t = np.linspace(0, 2*np.pi, 6) + rotation
star = np.zeros((12, 2))
star[::2] = np.c_[np.cos(t), np.sin(t)]
star[1::2] = 0.5*np.c_[np.cos(t + np.pi / 5), np.sin(t + np.pi / 5)]
return amplitude * star
def make_stars(n_stars=51, z_diff=0.05):
""" Make `2*n_stars-1` stars stacked in 3D
"""
amps = np.linspace(0.25, 1, n_stars)
amps = np.r_[amps, amps[:-1][::-1]]
rots = np.linspace(0, 2*np.pi, len(amps))
zamps = np.linspace
stars = []
for i, (amp, rot) in enumerate(zip(amps, rots)):
star = make_star(amplitude=amp, rotation=rot)
height = i*z_diff
z = np.full(len(star), height)
star3d = np.c_[star, z]
stars.append(star3d)
return stars
def polygon_to_boolean(points, xvals, yvals):
""" Convert `points` to a boolean indicator mask
over the specified domain
"""
x, y = np.meshgrid(xvals, yvals)
xy = np.c_[x.flatten(), y.flatten()]
mask = mpath.Path(points).contains_points(xy).reshape(x.shape)
return x, y, mask
def plot_contours(stars):
""" Plot a list of stars in 3D
"""
n = len(stars)
for i, star in enumerate(stars):
x, y, z = star.T
mlab.plot3d(*star.T)
#ax.plot3D(x, y, z, '-o', c=(0, 1-i/n, i/n))
#ax.set_xlim(-1, 1)
#ax.set_ylim(-1, 1)
mlab.show()
if __name__ == '__main__':
# Make and plot the 2D contours
stars3d = make_stars()
plot_contours(stars3d)
xvals = np.linspace(-1, 1, 101)
yvals = np.linspace(-1, 1, 101)
volume = np.dstack([
polygon_to_boolean(star[:,:2], xvals, yvals)[-1]
for star in stars3d
]).astype(float)
mlab.contour3d(volume, contours=[0.5])
mlab.show()
update 2
I now do this as follows:
- I use the fact that the paths in each z-slice are closed and simple and use
matplotlib.path
to determine points inside and outside of the contour. Using this idea, I convert the contours in each slice to a boolean-valued image, which is combined into a boolean-valued volume.
- Next, I use
skimage
's marching_cubes
method to obtain a triangulation of the surface for visualization.
Here's an example of the method. I think the data is slightly different, but you can definitely see that the results are much cleaner, and can handle surfaces that are disconnected or have holes.
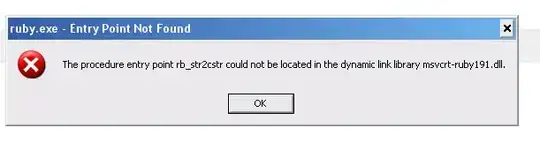
Original answer
Ok, here's the solution I came up with. It depends heavily on my data being roughly spherical and sampled at uniformly in z I think. Some of the other comments provide more information about more robust solutions. Since my data is roughly spherical I triangulate the azimuth and zenith angles from the spherical coordinate transform of my data points.
import numpy as np
import matplotlib.pyplot as plt
from mpl_toolkits.mplot3d import Axes3D
import matplotlib.tri as mtri
X = np.load('./mydatars.npy')
# My data points are strictly positive. This doesn't work if I don't center about the origin.
X -= X.mean(axis=0)
rad = np.linalg.norm(X, axis=1)
zen = np.arccos(X[:,-1] / rad)
azi = np.arctan2(X[:,1], X[:,0])
tris = mtri.Triangulation(zen, azi)
fig = plt.figure()
ax = fig.add_subplot(111, projection='3d')
ax.plot_trisurf(X[:,0], X[:,1], X[:,2], triangles=tris.triangles, cmap=plt.cm.bone)
plt.show()
Using the sample data from the pastebin above, this yields:
