IPOPT comes with Gekko if you don't want the headaches of compiling the solver on any platform that runs Python (Windows, MacOS, Linux, ARM Linux - Raspberry Pi). Here is a simple example:
pip install gekko
Solve HS71 with IPOPT
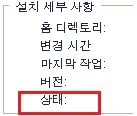
from gekko import GEKKO
import numpy as np
m = GEKKO()
x = m.Array(m.Var,4,value=1,lb=1,ub=5)
x1,x2,x3,x4 = x
# change initial values
x2.value = 5; x3.value = 5
m.Equation(x1*x2*x3*x4>=25)
m.Equation(x1**2+x2**2+x3**2+x4**2==40)
m.Minimize(x1*x4*(x1+x2+x3)+x3)
m.solve()
print('x: ', x)
print('Objective: ',m.options.OBJFCNVAL)
Results with IPOPT
******************************************************************************
This program contains Ipopt, a library for large-scale nonlinear optimization.
Ipopt is released as open source code under the Eclipse Public License (EPL).
For more information visit http://projects.coin-or.org/Ipopt
******************************************************************************
This is Ipopt version 3.12.10, running with linear solver ma57.
Number of nonzeros in equality constraint Jacobian...: 9
Number of nonzeros in inequality constraint Jacobian.: 0
Number of nonzeros in Lagrangian Hessian.............: 10
Total number of variables............................: 5
variables with only lower bounds: 1
variables with lower and upper bounds: 4
variables with only upper bounds: 0
Total number of equality constraints.................: 2
Total number of inequality constraints...............: 0
inequality constraints with only lower bounds: 0
inequality constraints with lower and upper bounds: 0
inequality constraints with only upper bounds: 0
iter objective inf_pr inf_du lg(mu) ||d|| lg(rg) alpha_du alpha_pr ls
0 1.6109693e+01 1.12e+01 2.50e+00 0.0 0.00e+00 - 0.00e+00 0.00e+00 0
1 1.6905655e+01 7.44e-01 5.14e-01 -0.9 1.36e-01 - 1.00e+00 1.00e+00f 1
2 1.7136202e+01 1.71e-01 4.57e-01 -1.0 9.40e-02 - 8.95e-01 1.00e+00h 1
3 1.6956645e+01 1.57e-01 7.85e-02 -2.0 1.78e-01 - 9.95e-01 1.00e+00h 1
4 1.7009269e+01 1.63e-02 1.20e-02 -2.8 3.94e-02 - 9.94e-01 1.00e+00h 1
5 1.7013888e+01 4.04e-04 1.76e-04 -4.6 6.22e-03 - 1.00e+00 1.00e+00h 1
6 1.7014017e+01 3.92e-07 6.03e-07 -10.4 1.46e-04 - 9.99e-01 1.00e+00h 1
Number of Iterations....: 6
(scaled) (unscaled)
Objective...............: 1.7014017127073458e+01 1.7014017127073458e+01
Dual infeasibility......: 6.0264909533529361e-07 6.0264909533529361e-07
Constraint violation....: 3.9234873865091858e-07 3.9234873865091858e-07
Complementarity.........: 7.2865190881096349e-08 7.2865190881096349e-08
Overall NLP error.......: 6.0264909533529361e-07 6.0264909533529361e-07
Number of objective function evaluations = 7
Number of objective gradient evaluations = 7
Number of equality constraint evaluations = 7
Number of inequality constraint evaluations = 0
Number of equality constraint Jacobian evaluations = 7
Number of inequality constraint Jacobian evaluations = 0
Number of Lagrangian Hessian evaluations = 6
Total CPU secs in IPOPT (w/o function evaluations) = 0.003
Total CPU secs in NLP function evaluations = 0.000
EXIT: Optimal Solution Found.
The solution was found.
The final value of the objective function is 17.0140171270735
---------------------------------------------------
Solver : IPOPT (v3.12)
Solution time : 9.299999990616925E-003 sec
Objective : 17.0140171270735
Successful solution
---------------------------------------------------
x: [[1.000000057] [4.74299963] [3.8211500283] [1.3794081795]]
Objective: 17.014017127
The default is to use a cloud compute service with more capable linear solvers. A local option is available for IPOPT on Windows but uses the MUMPS linear solver as one of the free distribution options.