One requirement for the reduceByKey
function is that is must be associative. To build some intuition on how reduceByKey
works, let's first see how an associative associative function helps us in a parallel computation:
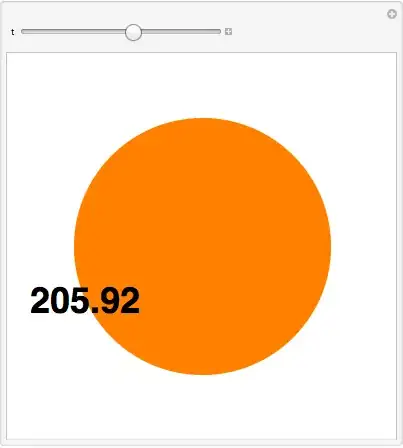
As we can see, we can break an original collection in pieces and by applying the associative function, we can accumulate a total. The sequential case is trivial, we are used to it: 1+2+3+4+5+6+7+8+9+10.
Associativity lets us use that same function in sequence and in parallel. reduceByKey
uses that property to compute a result out of an RDD, which is a distributed collection consisting of partitions.
Consider the following example:
// collection of the form ("key",1),("key,2),...,("key",20) split among 4 partitions
val rdd =sparkContext.parallelize(( (1 to 20).map(x=>("key",x))), 4)
rdd.reduceByKey(_ + _)
rdd.collect()
> Array[(String, Int)] = Array((key,210))
In spark, data is distributed into partitions. For the next illustration, (4) partitions are to the left, enclosed in thin lines. First, we apply the function locally to each partition, sequentially in the partition, but we run all 4 partitions in parallel. Then, the result of each local computation are aggregated by applying the same function again and finally come to a result.
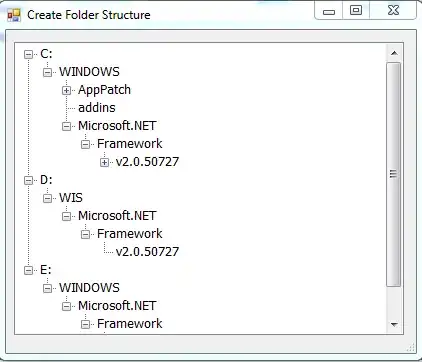
reduceByKey
is an specialization of aggregateByKey
aggregateByKey
takes 2 functions: one that is applied to each partition (sequentially) and one that is applied among the results of each partition (in parallel). reduceByKey
uses the same associative function on both cases: to do a sequential computing on each partition and then combine those results in a final result as we have illustrated here.