You'll need to subclass matplotlib.colors.Normalize
and pass in an instance of your new norm
to imshow
/contourf
/whatever plotting function you're using.
The basic idea is illustrated in the first option here: Shifted colorbar matplotlib (Not to shill one of my own questions too much, but I can't think of another example.)
However, that question deals specifically with setting a single data value to correspond to 0.5 in the colormap. It's not too hard to expand the idea to "piecewise" normalization, though:
import numpy as np
import matplotlib.pyplot as plt
from matplotlib.colors import Normalize
class PiecewiseNormalize(Normalize):
def __init__(self, xvalues, cvalues):
self.xvalues = xvalues
self.cvalues = cvalues
Normalize.__init__(self)
def __call__(self, value, clip=None):
# I'm ignoring masked values and all kinds of edge cases to make a
# simple example...
if self.xvalues is not None:
x, y = self.xvalues, self.cvalues
return np.ma.masked_array(np.interp(value, x, y))
else:
return Normalize.__call__(self, value, clip)
data = np.random.random((10,10))
data = 10 * (data - 0.8)
fig, ax = plt.subplots()
norm = PiecewiseNormalize([-8, -1, 0, 1.5, 2], [0, 0.1, 0.5, 0.7, 1])
im = ax.imshow(data, norm=norm, cmap=plt.cm.seismic, interpolation='none')
fig.colorbar(im)
plt.show()
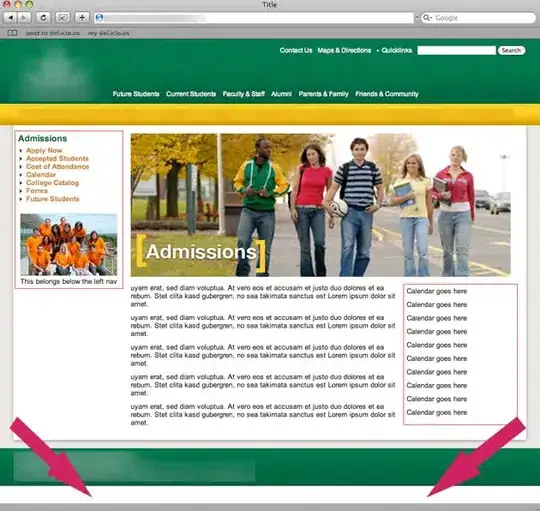
Note that 0.5 in the colormap (white) corresponds to a data value of 0, and the red and blue regions of the colormap are asymmetric (note the broad "pink" range vs the much narrower transition to dark blue).