The wave is pretty simple, so we'll fit a polynomial curve to the primary edge defined by the output of cv2
. First we want to get the points of that primary edge. Let's assume your origin is as it is on the image, at the top left. Looking at the original image, I think we'll have a good approximation for our points of interest if we just take the points with the largest y
in the range (750, 1500).
import cv2
import numpy as np
from matplotlib import pyplot as plt
from numba import jit
# Show plot
img = cv2.imread('wave.jpg',0)
edges = cv2.Canny(img,100,200)
# http://stackoverflow.com/a/29799815/1698058
# Get index of matching value.
@jit(nopython=True)
def find_first(item, vec):
"""return the index of the first occurence of item in vec"""
for i in range(len(vec)):
if item == vec[i]:
return i
return -1
bounds = [750, 1500]
# Now the points we want are the lowest-index 255 in each row
window = edges[bounds[1]:bounds[0]:-1].transpose()
xy = []
for i in range(len(window)):
col = window[i]
j = find_first(255, col)
if j != -1:
xy.extend((i, j))
# Reshape into [[x1, y1],...]
data = np.array(xy).reshape((-1, 2))
# Translate points back to original positions.
data[:, 1] = bounds[1] - data[:, 1]
If we graph these points, we can see they're very close to the ones we were aiming for.
plt.figure(1, figsize=(8, 16))
ax1 = plt.subplot(211)
ax1.imshow(edges,cmap = 'gray')
ax2 = plt.subplot(212)
ax2.axis([0, edges.shape[1], edges.shape[0], 0])
ax2.plot(data[:,1])
plt.show()
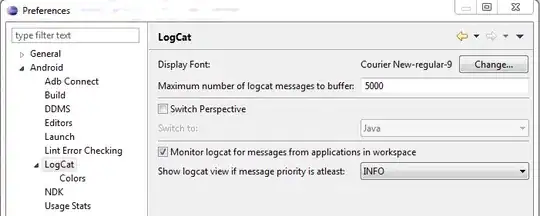
And now that we've got an array of coordinate pairs we can use numpy.polyfit
to generate the coefficients for a best-fit polynomial, and numpy.poly1d
to generate the function from those coefficients.
xdata = data[:,0]
ydata = data[:,1]
z = np.polyfit(xdata, ydata, 5)
f = np.poly1d(z)
and then plot to verify
t = np.arange(0, edges.shape[1], 1)
plt.figure(2, figsize=(8, 16))
ax1 = plt.subplot(211)
ax1.imshow(edges,cmap = 'gray')
ax2 = plt.subplot(212)
ax2.axis([0, edges.shape[1], edges.shape[0], 0])
ax2.plot(t, f(t))
plt.show()
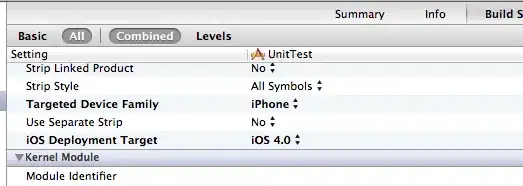