For completeness, another way to combine this down to a single cos()
call is to prepare an angle array where the second half has a phase shift of pi/2.
Borrowing the profiling code from Nico Schlömer, we get:
import perfplot
import numpy as np
def sin_cos(x):
return np.sin(x), np.cos(x)
def exp_ix(x):
eix = np.exp(1j * x)
return eix.imag, eix.real
def cos_shift(x):
angles = x[np.newaxis, :] + np.array(((-np.pi/2,), (0,)))
return tuple(np.cos(angles))
perfplot.save(
"out.png",
setup=lambda n: np.linspace(0.0, 2 * np.pi, n),
kernels=[sin_cos, exp_ix, cos_shift],
n_range=[2 ** k for k in range(1, 16)],
xlabel="n",
)
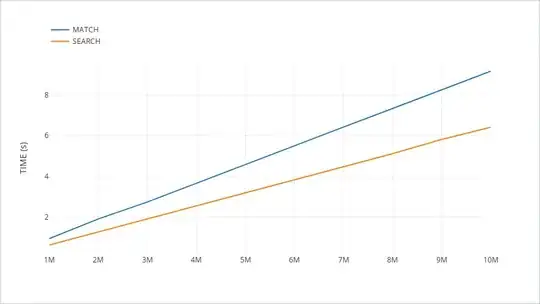
So it's slower than the separate sin
/cos
calls, but in some (narrow) contexts might be more convenient because - from the cos()
onward - it only needs to deal with a single array.