By using the cluster-mode
, the resource allocation has the structure shown in the following diagram.
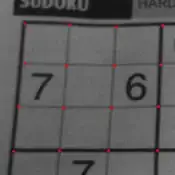
I will attempt to provide an illustration of the calculations for the resources allocation as made by YARN. First of all, the specs of each of the core nodes are the following (you can confirm here):
- memory: 244 GB
- cores/vCPUs: 32
This means that you can run at maximum:
- 2 executors per core node, which is calculated based on the memory and cores requested. Specifically,
available_cores / requested_cores = 32 / 13 = 2.46 -> 2
& available_mem / requested_mem = 244 / 90 = 2.71 = 2
.
- a single driver, without any more executors in a single core node. This is because when a driver runs in a core node, it leaves 244 - 180 = 64 GB of memory and 32 and 32-26 = 6 cores/vCPUS, which are not enough to run a separate executor.
So, from the existing pool of 60 core nodes, 1 node is used for the driver, leaving 59 remaining core nodes, which are running 59*2 = 118 executors.
Does that mean my Master node was not used?
If you mean whether the master node was used in order to execute the driver, then the answer is no. However, note that master was probably running a bunch of other applications in the meanwhile, which are out-of-scope in the context of this discussion (e.g. YARN resource manager, HDFS namenode etc.).
Is the driver running on the Master node or Core node?
The latter, the driver is running on the core node (since you used the --deploy-mode cluster
parameter).
Can I make the driver run on the Master node and let the 60 Cores hosting 120 working executors?
Yes! The way to do that is to execute the same command but with --deploy-mode client
(or leave that parameter unspecified, since at the time of writing this is used as default by Spark) in the master node.
By doing that, the resource allocation will have the structure shown in the following diagram.

Note that the Application Master will still consume some resources from the cluster ("stealing some resources from the executors). However, the AM resources are by default minimal, as can be seen here (spark.yarn.am.memory
and spark.yarn.am.cores
options), so it should not have a big impact.