How do you determine that the worst case is O(nLog(n)) and Ω(nLog(n)). In "Introduction to Algorithms" you determine the Theta time of insertion sort by counting number of times and cost of the execution of each statement. Is there a similar method for O and Omega?
- Merge Sort is a divide and conquer algorithm.
- You take a sequence of numbers, you break it in half recursively, you sort the halves individually and finally you merge the halves up recursively again leading to a sorted sequence of numbers.
Take the most simplistic case. How many elements do you touch† if you run the Merge Sort on that case? It is easy to visualize with the help of the image below. The elements touched at each level are cn and there are a total of lg n levels. So total elements touched, even in the easiest case, is cnLog(n).

Image Source
Thus, Merge Sort will always have a minimum complexity ≈ cnLog(n) and that's also written as Merge Sort is Ω(nLog(n)).
So even in the most simplistic run of this algorithm we have to touch the elements of the sequence a total of cnLog(n) times. Now...
How many times do we touch the elements of the sequence otherwise? Well, in any case whatsoever, the recursion tree of Merge Sort will be exactly the same as above. So, it turns out that we will always have a run-time complexity of Merge Sort proportional to nLog(n)! This means that both, the upper as well as the lower bound of Merge Sort is cnLog(n).
Hence, Merge Sort's run time complexity is not only Ω(nLog(n)) but also O(nLog(n)) which means that it is Ө(nLog(n)).
You can see/visualize the calculations done for Merge Sort by the graphical explanation above, which shows the calculations done at each level of the tree.
How can merge sort's worst-case be O(n*log(n)) but also O(n^2)?
That's straightforward, and I like the image below for understanding the terminologies.
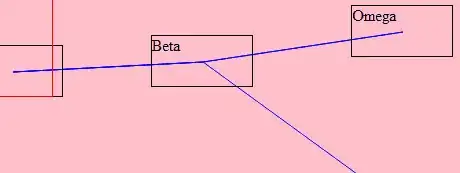
Image Source
Big-O only stresses on bounding above. It does not talk about how far above. So an algorithm can be described by more than one function as its Big-O complexity. However, the most informative of all of those is the one that is immediately above, otherwise every algorithm can simply be said to be O(infinity).
Now with that definition in place, we can easily say that Merge Sort is O(nLog(n)) as well as O(n2).
†'Touch' word in this explanation is the equivalent of what you have written in the question as "counting [the] number of times". During the run of the algorithm, every time a number is encountered - we say that the number is touched. So effectively we count the number of times a number is touched, to determine the complexity of the algorithm.