Finding directly the ellipses in that image can be quite tricky. You can, however, have a look here for a few info and references to code.
In this case, it's much easier to segment the 3 color: blue, red and yellow, find the outer contours, and fit an ellipse to them.
So, on this input image:

So, you first convert your image to HSV, and then apply some thresholds to recover the masks. Using morphological close operations will get rid of some holes, and link nearby blobs.
blue mask:
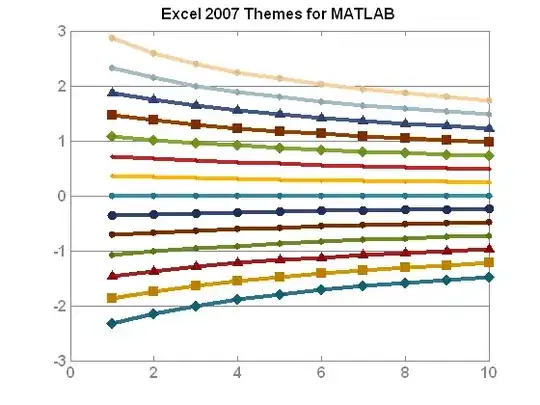
red mask:
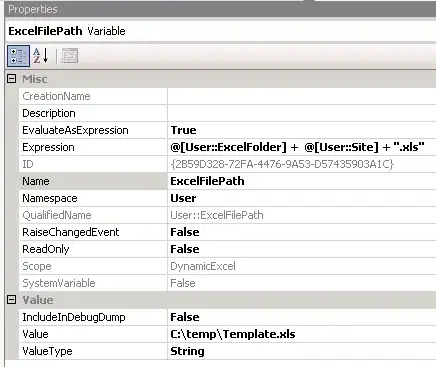
yellow mask:
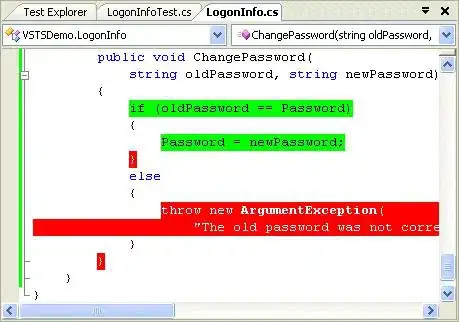
Then, you can retrieve the external contours from this masks, and keep only the largest (in case you find some smaller blob not belonging to the target).
Now you just need to fit an ellipse to these contours:

Please note that I tried also on the image in your other question. Blue target is deformed and so it's not an ellipse, so fitting an ellipse is not a good choice here:
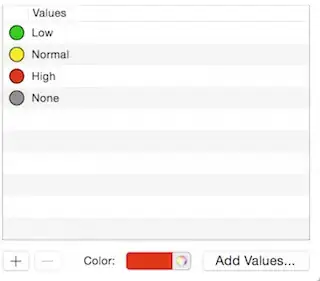
In this case may be better to use the convex hull of the contours, which will be more robust than the contour itself, if the mask is not perfect (code is given below):
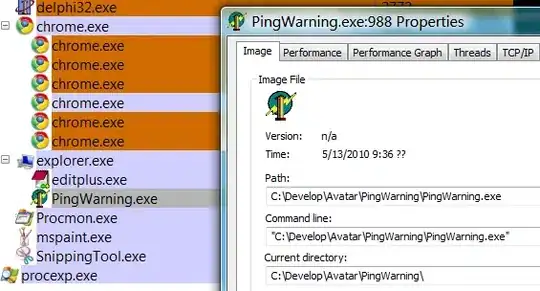
Code:
#include <opencv2/opencv.hpp>
#include <vector>
#include <string>
using namespace std;
using namespace cv;
int main()
{
// Load image
Mat3b img = imread("path_to_image");
// Convert to hsv
Mat3b hsv;
cvtColor(img, hsv, COLOR_BGR2HSV);
// Find masks for different colors
Mat1b blue_mask;
inRange(hsv, Scalar(90, 150, 150), Scalar(110, 255, 255), blue_mask);
Mat1b red_mask;
inRange(hsv, Scalar(160, 150, 100), Scalar(180, 255, 255), red_mask);
Mat1b yellow_mask;
inRange(hsv, Scalar(20, 150, 100), Scalar(30, 255, 255), yellow_mask);
// Apply morphological close
Mat1b kernel = getStructuringElement(MORPH_ELLIPSE, Size(11,11));
morphologyEx(blue_mask, blue_mask, MORPH_CLOSE, kernel);
morphologyEx(red_mask, red_mask, MORPH_CLOSE, kernel);
morphologyEx(yellow_mask, yellow_mask, MORPH_CLOSE, kernel);
// Find largest blob and draw it
vector<Point> blue_contour, red_contour, yellow_contour;
{
vector<vector<Point>> contours;
findContours(blue_mask.clone(), contours, RETR_EXTERNAL, CHAIN_APPROX_NONE);
blue_contour = *max_element(contours.begin(), contours.end(), [](const vector<Point>& lhs, const vector<Point>& rhs){
return contourArea(lhs) < contourArea(rhs); });
}
{
vector<vector<Point>> contours;
findContours(red_mask.clone(), contours, RETR_EXTERNAL, CHAIN_APPROX_NONE);
red_contour = *max_element(contours.begin(), contours.end(), [](const vector<Point>& lhs, const vector<Point>& rhs){
return contourArea(lhs) < contourArea(rhs); });
}
{
vector<vector<Point>> contours;
findContours(yellow_mask.clone(), contours, RETR_EXTERNAL, CHAIN_APPROX_NONE);
yellow_contour = *max_element(contours.begin(), contours.end(), [](const vector<Point>& lhs, const vector<Point>& rhs){
return contourArea(lhs) < contourArea(rhs); });
}
// Fit ellipse
RotatedRect blue_ellipse = fitEllipse(blue_contour);
RotatedRect red_ellipse = fitEllipse(red_contour);
RotatedRect yellow_ellipse = fitEllipse(yellow_contour);
// Draw ellipses
ellipse(img, blue_ellipse, Scalar(255, 0, 0), 3);
ellipse(img, red_ellipse, Scalar(0, 0, 255), 3);
ellipse(img, yellow_ellipse, Scalar(0, 255, 255), 3);
imshow("Result", img);
waitKey();
return 0;
}
Code for convex hull:
// Get convex hulls
vector<Point> blue_hull, red_hull, yellow_hull;
convexHull(blue_contour, blue_hull);
convexHull(red_contour, red_hull);
convexHull(yellow_contour, yellow_hull);
// Draw convex hulls
drawContours(img, vector < vector<Point> > {blue_hull}, 0, Scalar(255,0,0), 3);
drawContours(img, vector < vector<Point> > {red_hull}, 0, Scalar(0, 0, 255), 3);
drawContours(img, vector < vector<Point> > {yellow_hull}, 0, Scalar(0, 255, 255), 3);