Based off this incredible answer, I was able to create a monkey patch to beautifully do what you are looking for.
import pandas as pd
import seaborn as sns
import seaborn.timeseries
def _plot_range_band(*args, central_data=None, ci=None, data=None, **kwargs):
upper = data.max(axis=0)
lower = data.min(axis=0)
#import pdb; pdb.set_trace()
ci = np.asarray((lower, upper))
kwargs.update({"central_data": central_data, "ci": ci, "data": data})
seaborn.timeseries._plot_ci_band(*args, **kwargs)
seaborn.timeseries._plot_range_band = _plot_range_band
cluster_overload = pd.read_csv("TSplot.csv", delim_whitespace=True)
cluster_overload['Unit'] = cluster_overload.groupby(['Cluster','Week']).cumcount()
ax = sns.tsplot(time='Week',value="Overload", condition="Cluster", unit="Unit", data=cluster_overload,
err_style="range_band", n_boot=0)
Output Graph:
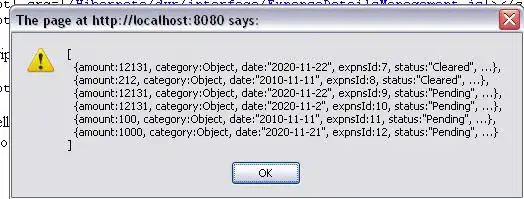
Notice that the shaded regions line up with the true maximum and minimums in the line graph!
If you figure out why the unit
variable is required, please let me know.
If you do not want them all on the same graph then:
import pandas as pd
import seaborn as sns
import seaborn.timeseries
def _plot_range_band(*args, central_data=None, ci=None, data=None, **kwargs):
upper = data.max(axis=0)
lower = data.min(axis=0)
#import pdb; pdb.set_trace()
ci = np.asarray((lower, upper))
kwargs.update({"central_data": central_data, "ci": ci, "data": data})
seaborn.timeseries._plot_ci_band(*args, **kwargs)
seaborn.timeseries._plot_range_band = _plot_range_band
cluster_overload = pd.read_csv("TSplot.csv", delim_whitespace=True)
cluster_overload['subindex'] = cluster_overload.groupby(['Cluster','Week']).cumcount()
def customPlot(*args,**kwargs):
df = kwargs.pop('data')
pivoted = df.pivot(index='subindex', columns='Week', values='Overload')
ax = sns.tsplot(pivoted.values, err_style="range_band", n_boot=0, color=kwargs['color'])
g = sns.FacetGrid(cluster_overload, row="Cluster", sharey=False, hue='Cluster', aspect=3)
g = g.map_dataframe(customPlot, 'Week', 'Overload','subindex')
Which produces the following, (you can obviously play with the aspect ratio if you think the proportions are off)
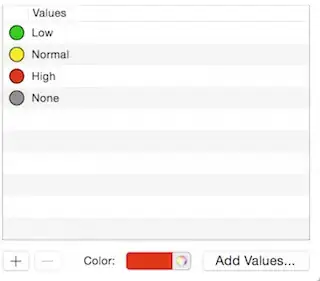