Similar to Romain X.'s answer but operates on the DataFrame instead of Series.
Random data:
np.random.seed(0)
df = pd.DataFrame(np.random.randn(100, 5), columns=list('ABCDE'))
df.iloc[::10] += np.random.randn() * 2 # this hopefully introduces some outliers
df.head()
Out:
A B C D E
0 2.529517 1.165622 1.744203 3.006358 2.633023
1 -0.977278 0.950088 -0.151357 -0.103219 0.410599
2 0.144044 1.454274 0.761038 0.121675 0.443863
3 0.333674 1.494079 -0.205158 0.313068 -0.854096
4 -2.552990 0.653619 0.864436 -0.742165 2.269755
Quartile calculations:
Q1 = df.quantile(0.25)
Q3 = df.quantile(0.75)
IQR = Q3 - Q1
And these are the numbers for each column:
((df < (Q1 - 1.5 * IQR)) | (df > (Q3 + 1.5 * IQR))).sum()
Out:
A 1
B 0
C 0
D 1
E 2
dtype: int64
In line with seaborn's calculations:
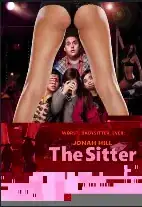
Note that the part before the sum ((df < (Q1 - 1.5 * IQR)) | (df > (Q3 + 1.5 * IQR))
) is a boolean mask so you can use it directly to remove outliers. This sets them to NaN, for example:
mask = (df < (Q1 - 1.5 * IQR)) | (df > (Q3 + 1.5 * IQR))
df[mask] = np.nan