I wrote a function to reverse-engineer the steps used in StatSmooth
's setup_params
function to get the actual method / formula parameters used for plotting.
The function expects a ggplot object as its input, with an additional optional parameter specifying the layer that corresponds to geom_smooth
(defaults to 1 if unspecified). It returns a text string in the form "Method: [method used], Formula: [formula used]"
, and also prints out all the parameters to console.
The envisaged use case is two-fold:
- Add the text string as-is to the plot as plot title / subtitle / caption, for quick reference during analysis;
- Read off the console printout, & include the information elsewhere or manually format it nicely (e.g. parsed plotmath expressions) for annotation in the plot, for report / presentation.
Function:
get.params <- function(plot, layer = 1){
# return empty string if the specified geom layer doesn't use stat = "smooth"
if(!"StatSmooth" %in% class(plot$layers[[layer]]$stat)){
message("No smoothing function was used in this geom layer.")
return("")
}
# recreate data used by this layer, in the format expected by StatSmooth
# (this code chunk takes heavy reference from ggplot2:::ggplot_build.ggplot)
layer.data <- plot$layers[[layer]]$layer_data(plot$data)
layout <- ggplot2:::create_layout(plot$facet, plot$coordinates)
data <- layout$setup(list(layer.data), plot$data, plot$plot_env)
data[[1]] <- plot$layers[[layer]]$compute_aesthetics(data[[1]], plot)
scales <- plot$scales
data[[1]] <- ggplot2:::scales_transform_df(scales = scales, df = data[[1]])
layout$train_position(data, scales$get_scales("x"), scales$get_scales("y"))
data <- layout$map_position(data)[[1]]
# set up stat params (e.g. replace "auto" with actual method / formula)
stat.params <- suppressMessages(
plot$layers[[layer]]$stat$setup_params(data = data,
params = plot$layers[[layer]]$stat_params)
)
# reverse the last step in setup_params; we don't need the actual function
# for mgcv::gam, just the name
if(identical(stat.params$method, mgcv::gam)) stat.params$method <- "gam"
print(stat.params)
return(paste0("Method: ", stat.params$method, ", Formula: ", deparse(stat.params$formula)))
}
Demonstration:
p <- ggplot(df, aes(x = x1, y = y)) # df is the sample dataset in the question
# default plot for 1000+ observations
# (method defaults to gam & formula to 'y ~ s(x, bs = "cs")')
p1 <- p + geom_smooth()
p1 + ggtitle(get.params(p1))
# specify method = 'gam'
# (formula defaults to `y ~ x`)
p2 <- p + geom_smooth(method='gam')
p2 + ggtitle(get.params(p2))
# specify method = 'gam' and splines for formula
p3 <- p + geom_smooth(method='gam',
method.args = list(family = "binomial"),
formula = y ~ splines::ns(x, 7))
p3 + ggtitle(get.params(p3))
# specify method = 'glm'
# (formula defaults to `y ~ x`)
p4 <- p + geom_smooth(method='glm')
p4 + ggtitle(get.params(p4))
# default plot for fewer observations
# (method defaults to loess & formula to `y ~ x`)
# observe that function is able to distinguish between plot data
# & data actually used by the layer
p5 <- p + geom_smooth(data = . %>% slice(1:500))
p5 + ggtitle(get.params(p5))
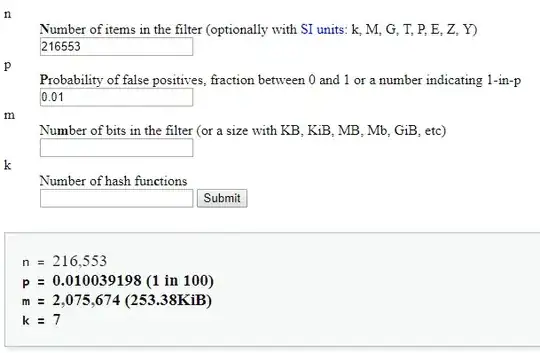