I can explain the concept at a very high level.
The main goal of the algorithm is to find an attribute that we will use for the first split. We can use various impurity metrics to evaluate the most significant attribute. Those impurity metrics can be Information Gain, Entropy, Gain Ratio, etc. But, if the decision variable is a continuous type variable, then we usually use another impurity metric 'standard deviation reduction'. But, whatever metric you use, depending on your algorithm (i.e. ID3, C4.5, etc) you actually find an attribute that will be used for splitting.
When you have a continuous type attribute, then things get a little tricky. You need to find a threshold value for an attribute that will give you the highest impurity (Entropy, Gain Ratio, Information Gain ... whatever). Then, you find which attribute's threshold value gives that highest impurity, and then chose an attribute accordingly, right?
Now, if the attribute is a continuous type and decision variable is also continuous type, then you can simply combine the above two concepts and generate the Regression Tree.
That means, as the decision variable is continuous type, you will use the metric (like Variance reduction) and chose the attribute which will give you the highest value of the chosen metric (i.e. variance reduction) for the threshold value of all attributes.
You can visualize such a regression tree using a Decision Tree Machine Learning software like SpiceLogic Decision Tree Software
Say, you have a data table like this:
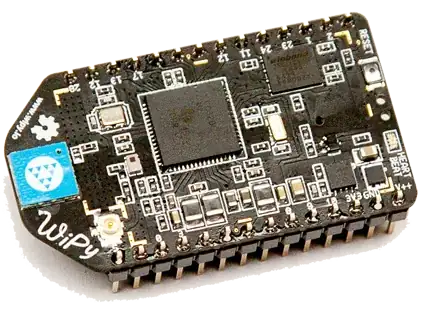
The software will generate the Regression tree like this:
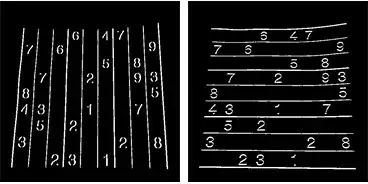