I thought of two ways to do this
use a generator
def multi_shift(a, n):
yield a
while n > 1:
a = np.append(np.nan, a[:-1])
yield a
n -= 1
np.stack(multi_shift(a, 5)).T
build a slice with broadcasting
rng = np.arange(len(a))
slc = rng[:, None] - rng[:5]
np.where(slc >= 0, a[slc], np.nan)
[[ 11. nan nan nan nan]
[ 98. 11. nan nan nan]
[ 74. 98. 11. nan nan]
[ 90. 74. 98. 11. nan]
[ 15. 90. 74. 98. 11.]
[ 55. 15. 90. 74. 98.]
[ 13. 55. 15. 90. 74.]
[ 11. 13. 55. 15. 90.]
[ 13. 11. 13. 55. 15.]
[ 26. 13. 11. 13. 55.]]
time testing
code
Divakar's stride
functions from this post
from scipy.linalg import toeplitz
from numpy.lib.stride_tricks import as_strided as strided
def pir1(a, n):
return np.stack(multi_shift(a, n)).T
def pir2(a, n):
rng = np.arange(len(a))
slc = rng[:, None] - rng[:5]
return np.where(slc >= 0, a[slc], np.nan)
# Suggested by @WarrenWeckesser
def toeplitz1(a, n):
return toeplitz(a, np.array([np.nan] * n))
# from @Divakar
def strided_nan_filled(a, W):
a_ext = np.concatenate((np.full(W-1, np.nan), a))
n = a_ext.strides[0]
out = strided(a_ext, shape=(a.size, W), strides=(n, n))[:,::-1]
return out
def strided_nan_filled_v2(a, W):
a_ext = np.concatenate(( np.full(W-1,np.nan) ,a))
n = a_ext.strides[0]
return strided(a_ext[W-1:], shape=(a.size,W), strides=(n,-n))
trials
from timeit import timeit
cols = pd.MultiIndex.from_product(
[['pir1', 'pir2', 'toeplitz1', 'stride'], [10, 100]])
results = pd.DataFrame(index=[100, 1000], columns=cols)
np.random.seed([3,1415])
for i in results.index:
a = np.random.rand(i)
for j in results.columns:
stmt = '{}(a, {})'.format(*j)
iprt = 'from __main__ import a, {}'.format(j[0])
results.set_value(i, j, timeit(stmt, iprt, number=100))
results.stack().plot.barh()
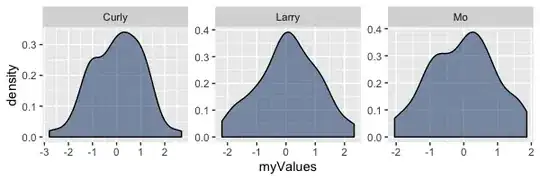
Drop pri1
and toeplitz
Clearly those take too long
This look leaves no doubt stride
is the way to go.
from timeit import timeit
cols = pd.MultiIndex.from_product(
[['pir2', 'strided_nan_filled', 'strided_nan_filled_v2'], [10, 100]])
results = pd.DataFrame(index=[100, 1000, 10000], columns=cols)
np.random.seed([3,1415])
for i in results.index:
a = np.random.rand(i)
for j in results.columns:
stmt = '{}(a, {})'.format(*j)
iprt = 'from __main__ import a, {}'.format(j[0])
results.set_value(i, j, timeit(stmt, iprt, number=100))
results.stack().plot.barh()
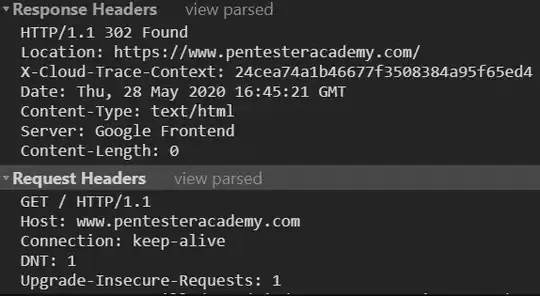