Suppose you have N objects that do not directly have a mathematical representation. For example words.
As neural networks are only able to work with tensors you should look for some way to translate those objects to tensors.
The solution is in a giant matrix (embedding matrix) where it relates each index of an object with its translation to tensor.
object_index_1: vector_1
object_index_1: vector_2
...
object_index_n: vector_n
Selecting the vector of a specific object can be translated to a matrix product in the following way:
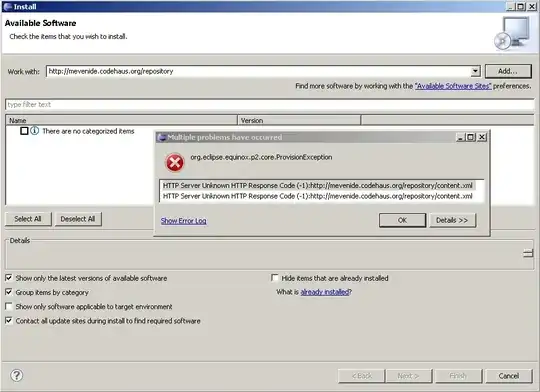
Where v is the one-hot vector that determines which word need to be translated. And M is the embedding matrix.
If we propose the usual pipeline, it would be the following:
- We have a list of objects.
objects = ['cat', 'dog', 'snake', 'dog', 'mouse', 'cat', 'dog', 'snake', 'dog']
- We transform these objects into indices (we calculate the unique objects).
unique = ['cat', 'dog', 'snake', 'mouse'] # list(set(objects))
objects_index = [0, 1, 2, 1, 3, 0, 1, 2, 1] #map(unique.index, objects)
- We transform these indices to a one hot vector (remember that there is only one where the index is)
objects_one_hot = [[1, 0, 0, 0], [0, 1, 0, 0], [0, 0, 1, 0], [0, 1, 0, 0],
[0, 0 , 0, 1], [1, 0, 0, 0], [0, 1, 0, 0], [0, 0, 1, 0], [0, 1, 0, 0]] # map(lambda x: [int(i==x) for i in range(len(unique))], objects_index)
#objects_one_hot is matrix is 4x9
- We create or use the embedding matrix:
#M = matrix of dim x 4 (where dim is the number of dimensions you want the vectors to have).
#In this case dim=2
M = np.array([[1, 1], [1, 2], [2, 2], [3,3]]).T # or... np.random.rand(2, 4)
#objects_vectors = M * objects_one_hot
objects_vectors = [[1, 1], [1, 2], [2, 2], [1, 2],
[3, 3], [1, 1], [1, 2], [2,2], [1, 2]] # M.dot(np.array(objects_one_hot).T)
Normally the embedding matrix is learned during the same model learning, to adapt the best vectors for each object. We already have the mathematical representation of the objects!
As you have seen we have used one hot and later a matrix product. What you really do is take the column of M that represents that word.
During the learning this M will be adapted to improve the representation of the object and as a consequence the loss goes down.