Well, it would be easier to give you a solution if you provided an example of your data, since it can vary a lot accordingly. I will try to build a case scenario and possible solution below.
If we take the example data and crosstab:
a = np.array(['foo', 'foo', 'foo', 'foo', 'bar', 'bar',
'bar', 'bar', 'foo', 'foo', 'foo'], dtype=object)
c = np.array(['dull', 'dull', 'shiny', 'dull', 'dull', 'weird',
'shiny', 'dull', 'shiny', 'shiny', 'shiny'], dtype=object)
CT = pd.crosstab(a, c, rownames=['a'], colnames=['c'])
CT
We have the following output:
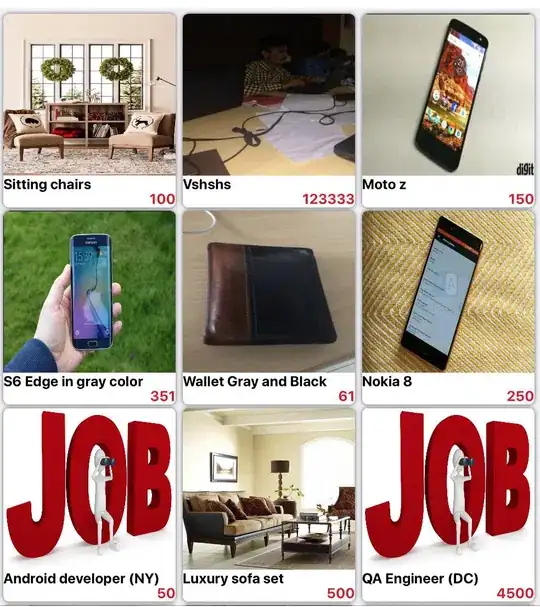
Thats a regular dataframe object, its just "crosstabed" or better yet "pivottabled" accordingly.
You would like to show:
- unique values of either df['col1'] or df['col2'] (cols/rows of the crosstab result)
- by marginal values (e.g. showing higher-count values of df['col1'] closer to the top)
So lets start with "1":
There are different ways you can do that, a simple solution would be to show the same dataframe object with boolean values for singular cases;
[CT == 1]
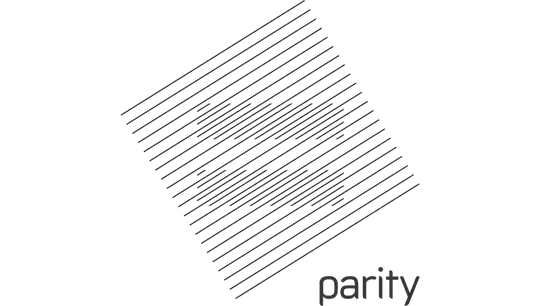
However, that format might not be what you desire in case of large dataframes.
You could just print the positive cases, or list/append 'em, a simple example would be:
for col in CT.columns:
for index in CT.index:
if CT.loc[index,col] == 1:
print (index,col,'singular')
Output:
('bar', 'shiny', 'singular')
('bar', 'weird', 'singular')
The second item/desire is more complicated.
You want to order by higher value. But there might be divergences. A higher value in one column, associated to one set of indexes, will most likely diverge in order from the second column (also associated in the same indexes).
Hence, you can choose to order by one specific column:
CT.sort_values('column_name', ascending=False)
Or, you can define a metric by which you want to order (row mean value) and sort accordingly.
Hope that helps!