You could indeed add noise with preprocessing_function.
Example script:
import random
import numpy as np
def add_noise(img):
'''Add random noise to an image'''
VARIABILITY = 50
deviation = VARIABILITY*random.random()
noise = np.random.normal(0, deviation, img.shape)
img += noise
np.clip(img, 0., 255.)
return img
# Prepare data-augmenting data generator
from keras.preprocessing.image import ImageDataGenerator
datagen = ImageDataGenerator(
rescale=1./255,
rotation_range=40,
width_shift_range=0.2,
height_shift_range=0.2,
zoom_range=0.2,
preprocessing_function=add_noise,
)
# Load a single image as our example
from keras.preprocessing import image
img_path = 'cat_by_irene_mei_flickr.png'
img = image.load_img(img_path, target_size=(299,299))
# Generate distorted images
images = [img]
img_arr = image.img_to_array(img)
img_arr = img_arr.reshape((1,) + img_arr.shape)
for batch in datagen.flow(img_arr, batch_size=1):
images.append( image.array_to_img(batch[0]) )
if len(images) >= 4:
break
# Display
import matplotlib.pyplot as plt
f, xyarr = plt.subplots(2,2)
xyarr[0,0].imshow(images[0])
xyarr[0,1].imshow(images[1])
xyarr[1,0].imshow(images[2])
xyarr[1,1].imshow(images[3])
plt.show()
Example images generated by the script:
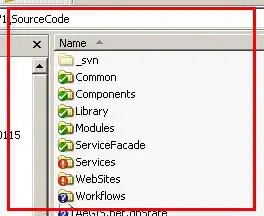