May be the apps are using anti-aliasing to make their binarized output look nicer. To obtain a similar effect, I first tried binarizing the image, but the result didn't look very nice with all the jagged edges. Then I applied pyramid upsampling and then downsampling to the result, and the output was better.
I didn't use adaptive thresholding however. I segmented the text-like regions and processed those regions only, then pasted them to form the final images. It is a kind of local thresholding using the Otsu method or the k-means (using combinations of thr_roi_otsu
, thr_roi_kmeans
and proc_parts
in the code). Below are some results.
Apply Otsu threshold to all text regions, then upsample followed by downsample:
Some text:
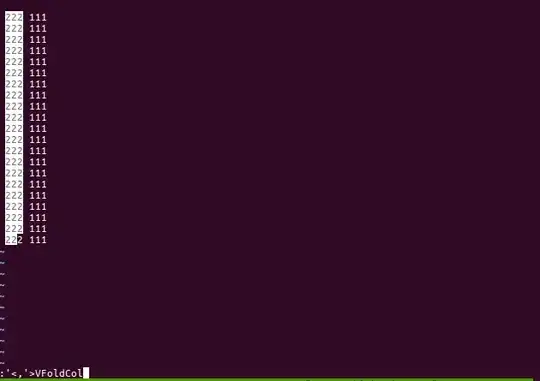
Full image:
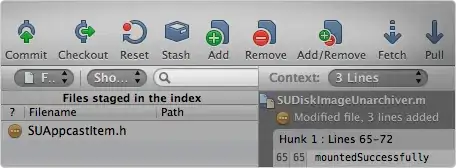
Upsample input image, apply Otsu threshold to individual text regions, downsample the result:
Some text:
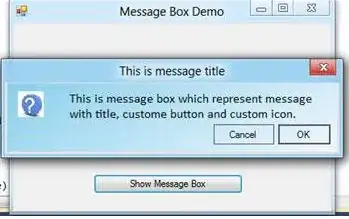
Full image:
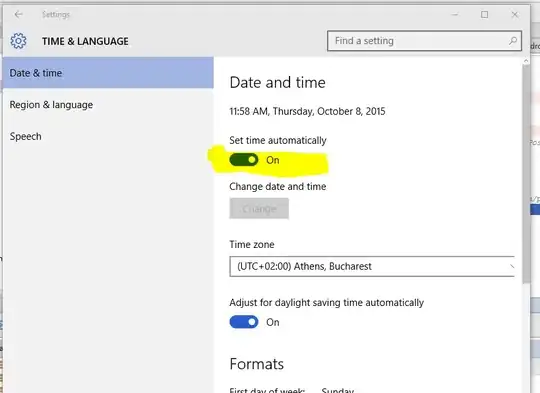
/*
apply Otsu threshold to the region in mask
*/
Mat thr_roi_otsu(Mat& mask, Mat& im)
{
Mat bw = Mat::ones(im.size(), CV_8U) * 255;
vector<unsigned char> pixels(countNonZero(mask));
int index = 0;
for (int r = 0; r < mask.rows; r++)
{
for (int c = 0; c < mask.cols; c++)
{
if (mask.at<unsigned char>(r, c))
{
pixels[index++] = im.at<unsigned char>(r, c);
}
}
}
// threshold pixels
threshold(pixels, pixels, 0, 255, CV_THRESH_BINARY | CV_THRESH_OTSU);
// paste pixels
index = 0;
for (int r = 0; r < mask.rows; r++)
{
for (int c = 0; c < mask.cols; c++)
{
if (mask.at<unsigned char>(r, c))
{
bw.at<unsigned char>(r, c) = pixels[index++];
}
}
}
return bw;
}
/*
apply k-means to the region in mask
*/
Mat thr_roi_kmeans(Mat& mask, Mat& im)
{
Mat bw = Mat::ones(im.size(), CV_8U) * 255;
vector<float> pixels(countNonZero(mask));
int index = 0;
for (int r = 0; r < mask.rows; r++)
{
for (int c = 0; c < mask.cols; c++)
{
if (mask.at<unsigned char>(r, c))
{
pixels[index++] = (float)im.at<unsigned char>(r, c);
}
}
}
// cluster pixels by gray level
int k = 2;
Mat data(pixels.size(), 1, CV_32FC1, &pixels[0]);
vector<float> centers;
vector<int> labels(countNonZero(mask));
kmeans(data, k, labels, TermCriteria(CV_TERMCRIT_EPS+CV_TERMCRIT_ITER, 10, 1.0), k, KMEANS_PP_CENTERS, centers);
// examine cluster centers to see which pixels are dark
int label0 = centers[0] > centers[1] ? 1 : 0;
// paste pixels
index = 0;
for (int r = 0; r < mask.rows; r++)
{
for (int c = 0; c < mask.cols; c++)
{
if (mask.at<unsigned char>(r, c))
{
bw.at<unsigned char>(r, c) = labels[index++] != label0 ? 255 : 0;
}
}
}
return bw;
}
/*
apply procfn to each connected component in the mask,
then paste the results to form the final image
*/
Mat proc_parts(Mat& mask, Mat& im, Mat (procfn)(Mat&, Mat&))
{
Mat tmp = mask.clone();
vector<vector<Point>> contours;
vector<Vec4i> hierarchy;
findContours(tmp, contours, hierarchy, CV_RETR_CCOMP, CV_CHAIN_APPROX_SIMPLE, Point(0, 0));
Mat byparts = Mat::ones(im.size(), CV_8U) * 255;
for(int idx = 0; idx >= 0; idx = hierarchy[idx][0])
{
Rect rect = boundingRect(contours[idx]);
Mat msk = mask(rect);
Mat img = im(rect);
// process the rect
Mat roi = procfn(msk, img);
// paste it to the final image
roi.copyTo(byparts(rect));
}
return byparts;
}
int _tmain(int argc, _TCHAR* argv[])
{
Mat im = imread("1.jpg", 0);
// detect text regions
Mat morph;
Mat kernel = getStructuringElement(MORPH_ELLIPSE, Size(3, 3));
morphologyEx(im, morph, CV_MOP_GRADIENT, kernel, Point(-1, -1), 1);
// prepare a mask for text regions
Mat bw;
threshold(morph, bw, 0, 255, THRESH_BINARY | THRESH_OTSU);
morphologyEx(bw, bw, CV_MOP_DILATE, kernel, Point(-1, -1), 10);
Mat bw2x, im2x;
pyrUp(bw, bw2x);
pyrUp(im, im2x);
// apply Otsu threshold to all text regions, then upsample followed by downsample
Mat otsu1x = thr_roi_otsu(bw, im);
pyrUp(otsu1x, otsu1x);
pyrDown(otsu1x, otsu1x);
// apply k-means to all text regions, then upsample followed by downsample
Mat kmeans1x = thr_roi_kmeans(bw, im);
pyrUp(kmeans1x, kmeans1x);
pyrDown(kmeans1x, kmeans1x);
// upsample input image, apply Otsu threshold to all text regions, downsample the result
Mat otsu2x = thr_roi_otsu(bw2x, im2x);
pyrDown(otsu2x, otsu2x);
// upsample input image, apply k-means to all text regions, downsample the result
Mat kmeans2x = thr_roi_kmeans(bw2x, im2x);
pyrDown(kmeans2x, kmeans2x);
// apply Otsu threshold to individual text regions, then upsample followed by downsample
Mat otsuparts1x = proc_parts(bw, im, thr_roi_otsu);
pyrUp(otsuparts1x, otsuparts1x);
pyrDown(otsuparts1x, otsuparts1x);
// apply k-means to individual text regions, then upsample followed by downsample
Mat kmeansparts1x = proc_parts(bw, im, thr_roi_kmeans);
pyrUp(kmeansparts1x, kmeansparts1x);
pyrDown(kmeansparts1x, kmeansparts1x);
// upsample input image, apply Otsu threshold to individual text regions, downsample the result
Mat otsuparts2x = proc_parts(bw2x, im2x, thr_roi_otsu);
pyrDown(otsuparts2x, otsuparts2x);
// upsample input image, apply k-means to individual text regions, downsample the result
Mat kmeansparts2x = proc_parts(bw2x, im2x, thr_roi_kmeans);
pyrDown(kmeansparts2x, kmeansparts2x);
return 0;
}