The short answer is : you have to actually layout the graph! More exactly, you have to find an embedding of the graph in the plane - assuming that there is one without edge crossings.
So, your embedding above is :
1: [2, 7, 3]
2: [1, 4, 8]
3: [1, 5, 6, 4]
...
which is each vertex with an ordering on its neighbour set. You have to specify if that ordering is clockwise or anti-clockwise, but otherwise that should be all.
Once you have the embedding, it is possible to recover the faces using a combinatorial map. This looks trickier than it really is, although it does involve darts (or flags).
First, break each edge into flags (vertex + half-edge) and make a permutation (sigma in the wiki description) that stores the map. For example, we could label the flags in the same order as the map - then 1: [2, 7, 3] becomes {1->2 : 1, 1->7 : 2, 1->3 : 3} and so on.
For example, a cube (note : removed the middle edge!):
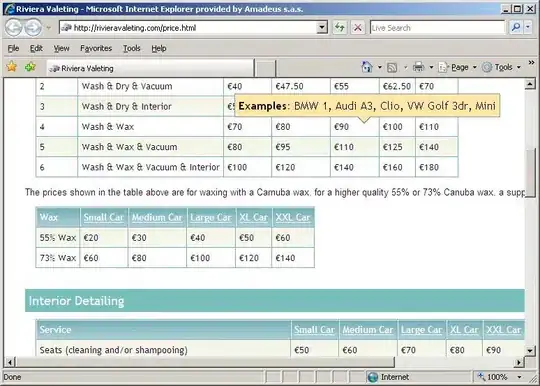
Then calculate alpha (the involution permutation) which just maps flags to the other flag across the edge. Finally, phi is the product of these two permutations, and the cycles of phi gives you the faces.
So, from the phi in the image, we have (1, 6, 24, 19) which is the outer face (note that these are darts, so we consider the vertex it starts from).