I think that using SIFT and a keypoints matcher is the most robust approach to this problem. It should work fine with many different form templates. However, SIFT algorithm being patented, here is another approach that should work well too:
Step 1: Binarize
- Threshold your photo and the template form using
THRESH_OTSU
tag.
- Invert the two binary result
Mat
s with the bitwise_not
function.
Step 2: Find the forms' bounding rect
For the two binary Mat
s from Step 1:
- Find the largest contour.
- Use
approxPolyDP
to approximate the found contour to a quadrilateral (see picture above).
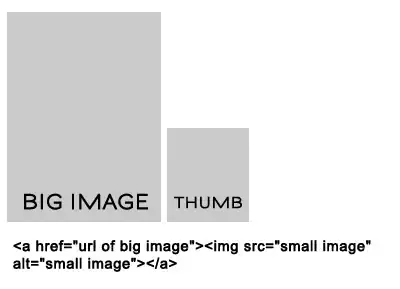
In my code, this is done inside getQuadrilateral()
.
Step 3: Homography and Warping
- Find the transformation between the two forms' bounding rect with
findHomography
- Warp the photo's binary
Mat
using warpPerspective
(and the homography Mat
computed previously).

Step 4: Comparison between template and photo
- Dilate the template form's binary
Mat
.
- Subtract the warped binary
Mat
and the dilated template form's binary Mat
.
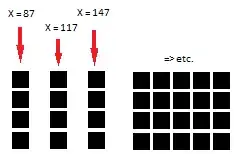
This allows to extract the filled informations. But you can also do it the other way around:
Template form - Dilated Warped Mat
In this case, the result of the subtraction should be totally black. I would then use mean
to get the average pixel's value. Finally, if that value is smaller than (let's say) 2, I would assume the form on the photo is matching the template form.
Here is the C++ code, it shouldn't be too hard to translate to Python :)
vector<Point> getQuadrilateral(Mat & grayscale)
{
vector<vector<Point>> contours;
findContours(grayscale, contours, RETR_EXTERNAL, CHAIN_APPROX_NONE);
vector<int> indices(contours.size());
iota(indices.begin(), indices.end(), 0);
sort(indices.begin(), indices.end(), [&contours](int lhs, int rhs) {
return contours[lhs].size() > contours[rhs].size();
});
vector<vector<Point>> polygon(1);
approxPolyDP(contours[indices[0]], polygon[0], 5, true);
if (polygon[0].size() == 4) // we have found a quadrilateral
{
return(polygon[0]);
}
return(vector<Point>());
}
int main(int argc, char** argv)
{
Mat templateImg, sampleImg;
templateImg = imread("template-form.jpg", 0);
sampleImg = imread("sample-form.jpg", 0);
Mat templateThresh, sampleTresh;
threshold(templateImg, templateThresh, 0, 255, THRESH_OTSU);
threshold(sampleImg, sampleTresh, 0, 255, THRESH_OTSU);
bitwise_not(templateThresh, templateThresh);
bitwise_not(sampleTresh, sampleTresh);
vector<Point> corners_template = getQuadrilateral(templateThresh);
vector<Point> corners_sample = getQuadrilateral(sampleTresh);
Mat homography = findHomography(corners_sample, corners_template);
Mat warpSample;
warpPerspective(sampleTresh, warpSample, homography, Size(templateThresh.cols, templateThresh.rows));
Mat element_dilate = getStructuringElement(MORPH_ELLIPSE, Size(8, 8));
dilate(templateThresh, templateThresh, element_dilate);
Mat diff = warpSample - templateThresh;
imshow("diff", diff);
waitKey(0);
return 0;
}
I Hope it is clear enough! ;)
P.S. This great answer helped me to retrieve the largest contour.