I'm not sure if this is comparable. Kubernetes can be run as a Mesos framework. Its scheduler is described here. It base on filtering and ranking the nodes.
Mesos two step scheduling is more depend on framework algorithm.
- Mesos presents the offers to the framework based on DRF algorithm. Frameworks could be prioritized as well by using roles and weights.
- Frameworks decide which task to run based on give offer. Every framework can implement its own algorithm for matching task with offer. This is a NP hard problem
Appendix Quote from https://medium.com/@ArmandGrillet/comparison-of-container-schedulers-c427f4f7421
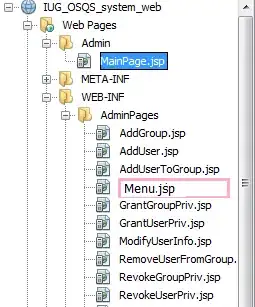
Monolithic scheduling
Monolithic schedulers are composed of a single
scheduling agent handling all the requests, they are commonly used in
high-performance computing. A monolithic scheduler generally applies a
single-algorithm implementation for all incoming jobs thus running
different scheduling logic depending on job types is difficult. Apache
Hadoop YARN [55], a popular architecture for Hadoop that delegates
many scheduling functions to per-application components, is a
monolithic scheduler architecture due to the fact that the resource
requests from application masters have to be sent to a single global
scheduler in the resource master.
Two-level scheduling
A two-level
scheduler adjusts the allocation of resources to each scheduler
dynamically using a central coordinator to decide how many resources
each sub-cluster can have, it is used in Mesos [50] and was used for
Hadoop-on-Demand (now replaced by YARN). With this architecture, the
allocator avoids conflicts by offering a given resource to only one
framework at a time and attempts to achieve dominant resource fairness
by choosing the order and the sizes of the resources it offers. Only
one framework is examining a resource at a time thus the concurrency
control is called pessimistic, a strategy that is less error-prone but
slower compared to an optimistic concurrency control offering a
resource to many frameworks at the same time.
Shared-state scheduling
Omega grants each scheduler full access to the entire cluster,
allowing them to compete in a free-for-all manner. There is no central
resource allocator as all of the resource-allocation decisions take
place in the schedulers. There is no central policy-enforcement
engine, individual schedulers are taking decisions in this variant of
the two-level scheme. By supporting independent scheduler
implementations and exposing the entire allocation state of the
schedulers, Omega can scale to many schedulers and works with
different workloads with their own scheduling policies [54].