You could use the identity (I only found it in the german Wikipedia but there are probably other sources as well):
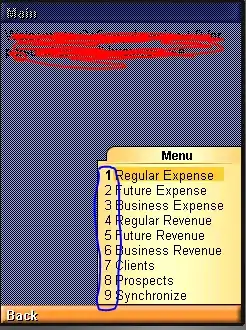
This identity can be constructed using the "logarithm rules" on the normal definition of the geometric mean:
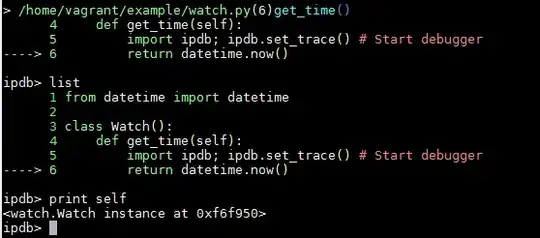
The base a
can be chosen arbitarly, so you could use np.log
(and np.exp
as inverse operation):
import numpy as np
def nangmean(arr, axis=None):
arr = np.asarray(arr)
inverse_valids = 1. / np.sum(~np.isnan(arr), axis=axis) # could be a problem for all-nan-axis
rhs = inverse_valids * np.nansum(np.log(arr), axis=axis)
return np.exp(rhs)
And it seems to work:
>>> l = [[1, 2, 3], [1, np.nan, 3], [np.nan, 2, np.nan]]
>>> nangmean(l)
1.8171205928321397
>>> nangmean(l, axis=1)
array([ 1.81712059, 1.73205081, 2. ])
>>> nangmean(l, axis=0)
array([ 1., 2., 3.])
In NumPy 1.10 also np.nanprod
was added, so you could also use the normal definition:
import numpy as np
def nangmean(arr, axis=None):
arr = np.asarray(arr)
valids = np.sum(~np.isnan(arr), axis=axis)
prod = np.nanprod(arr, axis=axis)
return np.power(prod, 1. / valids)