If you want to see the frequence of each comments related to each Person and a new column Ready you can do this with the following code :
set.seed(123456)
### I use the same data as the previous example, thank you for providing this !
data <-data.frame(date = Sys.Date() - sample(100),
Group = c("Cars","Trucks") %>% sample(100,replace=T),
Reporting_person = c("A","B","C") %>% sample(100,replace=T),
Comments = c("Awesome","Meh","NC") %>% sample(100,replace=T),
Ready = as.character(c("Yes","No") %>% sample(100,replace=T))
)
library(dplyr)
data %>%
group_by(Reporting_person,Ready) %>%
count(Comments) %>%
mutate(prop = prop.table(n))
If what you are asking is to see if a change occurs in the comments over time and to see if that change is correlated with an event (like Ready) you can use the following code:
library(dplyr)
### Creating a column comments at time + plus
new = data %>%
arrange(Reporting_person,Group,date) %>%
group_by(Group,Reporting_person) %>%
mutate(comments_plusone=lag(Comments))
new = na.omit(new)
### Creating the change column 1 is a change , 0 no change
new$Change = as.numeric(new$Comments != new$comments_plusone)
### Get the correlation between Change and the events...
### Chi-test to test if correlation between the event and the change
### Not that using Pearson correlation is not pertinent here :
tbl <- table(new$Ready,new$Change)
chi2 = chisq.test(tbl, correct=F)
c(chi2$statistic, chi2$p.value)
sqrt(chi2$statistic / sum(tbl))
You should get no significative correlation with this example. As you can clearly see when you illustrate the table.
plot(tbl)
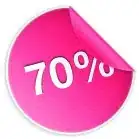
Not that using cor function is not appropriate working with two binary variable.
Here a post in this topic.... Correlation between two binary
Frequence of change by change of State
Following your comments, I am adding this code:
newR = data %>%
arrange(Reporting_person,Group,date) %>%
group_by(Group,Reporting_person) %>%
mutate(Ready_plusone=lag(Ready))
newR = na.omit(newR)
###------------------------Add the column to the new data frame
### Creating the REady change column 1 is a change , 0 no change
### Creating the change of state , I use this because you seem to have more than 2 levels.
new$State_change = paste(newR$Ready,newR$Ready_plusone,sep="_")
### Getting the frequency of Change by Change of State(Ready Yes-no..no-yes..)
result <- new %>%
group_by(Reporting_person,State_change) %>%
count(Change) %>%
mutate(Frequence = prop.table(n))%>%
filter(Change==1)
### Tidyr is a great library for reshape data, you want the wide format of the previous long
### dataframe... However doing this will generate a lot of NA so If I were you I would get
### the result format instead of the following but this could be helpful for future need so here you go.
library(tidyr)
final = as.data.frame(spread(result, key = State_change, value = prop))[,c(1,4:7)]
Hope this help :)