this gives you an idea of the simplest codes.
[ Sample ]:
# https://stackoverflow.com/questions/71748896/how-to-plot-a-graph-of-training-time-and-batch-size-of-neural-network
import os
from os.path import exists
import matplotlib.pyplot as plt
import tensorflow as tf
import time
import h5py
"""""""""""""""""""""""""""""""""""""""""""""""""""""""""
[PhysicalDevice(name='/physical_device:GPU:0', device_type='GPU')]
None
"""""""""""""""""""""""""""""""""""""""""""""""""""""""""
physical_devices = tf.config.experimental.list_physical_devices('GPU')
assert len(physical_devices) > 0, "Not enough GPU hardware devices available"
config = tf.config.experimental.set_memory_growth(physical_devices[0], True)
print(physical_devices)
print(config)
os.environ['TF_GPU_ALLOCATOR'] = 'cuda_malloc_async'
print(os.getenv('TF_GPU_ALLOCATOR'))
"""""""""""""""""""""""""""""""""""""""""""""""""""""""""
Variables
"""""""""""""""""""""""""""""""""""""""""""""""""""""""""
epoch_1_time = [ ]
epoch_5_time = [ ]
epoch_10_time = [ ]
epoch_50_time = [ ]
epoch_100_time = [ ]
database_buffer = "F:\\models\\buffer\\" + os.path.basename(__file__).split('.')[0] + "\\TF_DataSets_01.h5"
database_buffer_dir = os.path.dirname(database_buffer)
if not exists(database_buffer_dir) :
os.mkdir(database_buffer_dir)
print("Create directory: " + database_buffer_dir)
"""""""""""""""""""""""""""""""""""""""""""""""""""""""""
Functions
"""""""""""""""""""""""""""""""""""""""""""""""""""""""""
# ...
"""""""""""""""""""""""""""""""""""""""""""""""""""""""""
DataSet
"""""""""""""""""""""""""""""""""""""""""""""""""""""""""
(train_images, train_labels), (test_images, test_labels) = tf.keras.datasets.cifar10.load_data()
# Create hdf5 file
hdf5_file = h5py.File(database_buffer, mode='w')
# Train images
hdf5_file['x_train'] = train_images
hdf5_file['y_train'] = train_labels
# Test images
hdf5_file['x_test'] = test_images
hdf5_file['y_test'] = test_labels
hdf5_file.close()
# Visualize dataset train sample
hdf5_file = h5py.File(database_buffer, mode='r')
# Load features
# x_train = hdf5_file['x_train'][0: 50000]
# x_test = hdf5_file['x_test'][0: 10000]
# y_train = hdf5_file['y_train'][0: 50000]
# y_test = hdf5_file['y_test'][0: 10000]
x_train = hdf5_file['x_train'][0: 100]
x_test = hdf5_file['x_test'][0: 100]
y_train = hdf5_file['y_train'][0: 100]
y_test = hdf5_file['y_test'][0: 100]
"""""""""""""""""""""""""""""""""""""""""""""""""""""""""
: Model Initialize
"""""""""""""""""""""""""""""""""""""""""""""""""""""""""
model = tf.keras.models.Sequential([
tf.keras.layers.InputLayer(input_shape=( 32, 32, 3 )),
tf.keras.layers.Normalization(mean=3., variance=2.),
tf.keras.layers.Normalization(mean=4., variance=6.),
tf.keras.layers.Conv2DTranspose(2, 3, activation='relu', padding="same"),
tf.keras.layers.MaxPooling2D(pool_size=(2, 2), strides=(1, 1), padding='valid'),
tf.keras.layers.Flatten(),
tf.keras.layers.Dense(4 * 256),
tf.keras.layers.Reshape((4 * 256, 1)),
tf.keras.layers.LSTM(128, return_sequences=True, return_state=False),
tf.keras.layers.LSTM(128, name='LSTM256'),
tf.keras.layers.Dropout(0.2),
])
model.add(tf.keras.layers.Flatten())
model.add(tf.keras.layers.Dense(64, activation='relu', name='dense64'))
model.add(tf.keras.layers.Dense(7))
model.summary()
"""""""""""""""""""""""""""""""""""""""""""""""""""""""""
: Callback
"""""""""""""""""""""""""""""""""""""""""""""""""""""""""
class custom_callback_5(tf.keras.callbacks.Callback):
global epoch_5
val_dir = os.path.join(log_dir, 'validation')
print('val_dir: ' + val_dir)
epoch_5 = 0
def on_epoch_end( self, epoch, logs={} ):
global epoch_5
time_counter = time.perf_counter()
epoch_1_time.append( epoch )
if epoch == 1 :
###
epoch_5 = time_counter
if epoch % 5 == 0 :
epoch_5 = time_counter
epoch_5_time.append( epoch_5 )
### updates ###
with file_writer.as_default():
tf.summary.scalar("epoch_5", epoch_5, step=epoch)
file_writer.flush()
custom_callback_5 = custom_callback_5()
class custom_callback_10(tf.keras.callbacks.Callback):
global epoch_10
epoch_10 = 0
def on_epoch_end( self, epoch, logs={} ):
global epoch_10
time_counter = time.perf_counter()
#epoch_1_time.append( epoch )
if epoch == 1 :
###
epoch_10 = time_counter
if epoch % 10 == 0 :
epoch_10 = time_counter
epoch_10_time.append( epoch_10 )
### updates ###
with file_writer.as_default():
tf.summary.scalar("epoch_10", epoch_10, step=epoch)
file_writer.flush()
custom_callback_10 = custom_callback_10()
class custom_callback_50(tf.keras.callbacks.Callback):
global epoch_50
epoch_50 = 0
def on_epoch_end( self, epoch, logs={} ):
global epoch_50
time_counter = time.perf_counter()
#epoch_1_time.append( epoch )
if epoch == 1 :
###
epoch_50 = time_counter
if epoch % 50 == 0 :
epoch_50 = time_counter
epoch_50_time.append( epoch_50 )
### updates ###
with file_writer.as_default():
tf.summary.scalar("epoch_50", epoch_50, step=epoch)
file_writer.flush()
custom_callback_50 = custom_callback_50()
class custom_callback_100(tf.keras.callbacks.Callback):
global epoch_100
epoch_100 = 0
def on_epoch_end( self, epoch, logs={} ):
global epoch_100
time_counter = time.perf_counter()
#epoch_1_time.append( epoch )
if epoch == 1 :
###
epoch_100 = time_counter
if epoch % 100 == 0 :
epoch_100 = time_counter
epoch_100_time.append( epoch_100 )
### updates ###
with file_writer.as_default():
tf.summary.scalar("epoch_100", epoch_100, step=epoch)
file_writer.flush()
custom_callback_100 = custom_callback_100()
"""""""""""""""""""""""""""""""""""""""""""""""""""""""""
: Optimizer
"""""""""""""""""""""""""""""""""""""""""""""""""""""""""
optimizer = tf.keras.optimizers.Nadam( learning_rate=0.0001, beta_1=0.9, beta_2=0.999, epsilon=1e-07, name='Nadam' )
"""""""""""""""""""""""""""""""""""""""""""""""""""""""""
: Loss Fn
"""""""""""""""""""""""""""""""""""""""""""""""""""""""""
lossfn = tf.keras.losses.MeanSquaredLogarithmicError(reduction=tf.keras.losses.Reduction.AUTO, name='mean_squared_logarithmic_error')
"""""""""""""""""""""""""""""""""""""""""""""""""""""""""
: Model Summary
"""""""""""""""""""""""""""""""""""""""""""""""""""""""""
model.compile(optimizer=optimizer, loss=lossfn)
"""""""""""""""""""""""""""""""""""""""""""""""""""""""""
: Training
"""""""""""""""""""""""""""""""""""""""""""""""""""""""""
history = model.fit(x_train, y_train, epochs=1000, batch_size=5 ,validation_data=(x_test, y_test), callbacks=[custom_callback_5])
history = model.fit(x_train, y_train, epochs=1000, batch_size=10 ,validation_data=(x_test, y_test), callbacks=[custom_callback_10])
history = model.fit(x_train, y_train, epochs=1000, batch_size=50 ,validation_data=(x_test, y_test), callbacks=[custom_callback_50])
history = model.fit(x_train, y_train, epochs=1000, batch_size=100 ,validation_data=(x_test, y_test), callbacks=[custom_callback_100])
plt.plot(epoch_1_time, epoch_5_time)
plt.plot(epoch_1_time, epoch_10_time)
plt.plot(epoch_1_time, epoch_50_time)
plt.plot(epoch_1_time, epoch_100_time)
plt.legend(["epoch_5_time", "epoch_10_time", "epoch_50_time", "epoch_100_time"])
plt.show()
plt.close()
input('...')
## tensorboard --inspect --logdir="F:\\models\\checkpoint\\test_tf_plot_graph\\"
## tensorboard --logdir="F:\\models\\checkpoint\\test_tf_plot_graph\\"
[ Output ]:
Event statistics for F:\\models\\checkpoint\\test_tf_plot_graph\validation:
audio -
graph -
histograms -
images -
scalars -
sessionlog:checkpoint -
sessionlog:start -
sessionlog:stop -
tensor
first_step 20
last_step 6
max_step 140
min_step 0
num_steps 14
outoforder_steps [(20, 0), (40, 1), (60, 2), (80, 3), (100, 4), (120, 5), (140, 6)]
======================================================================
...
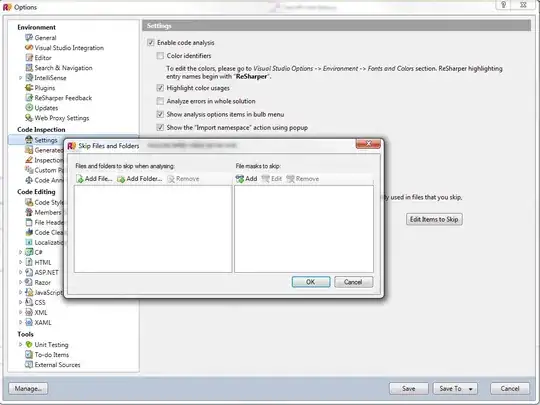
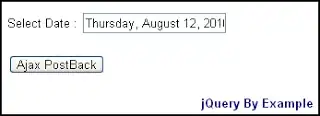