Here's one approach that uses data.table
, and a re-written haversine formula that I did for this question so that it will work inside data.table
operations
The idea is to do a data.table
join on every single point, to every single point, but within the join calculate the distance between each pair of points, and remove those that are outside the threshold. This is inspired by @Jaap 's excellent answer here
Setup
The haversine formula is
## Haversine formula
dt.haversine <- function(lat_from, lon_from, lat_to, lon_to, r = 6378137){
radians <- pi/180
lat_to <- lat_to * radians
lat_from <- lat_from * radians
lon_to <- lon_to * radians
lon_from <- lon_from * radians
dLat <- (lat_to - lat_from)
dLon <- (lon_to - lon_from)
a <- (sin(dLat/2)^2) + (cos(lat_from) * cos(lat_to)) * (sin(dLon/2)^2)
return(2 * atan2(sqrt(a), sqrt(1 - a)) * r)
}
The data I'm using for this example comes from my googleway
package, and it's of the tram stops on the City Loop tram in Melbourne
library(googleway)
## Tram stops data
head(tram_stops)
# stop_id stop_name stop_lat stop_lon
# 1 17880 10-Albert St/Nicholson St (Fitzroy) -37.8090 144.9731
# 2 17892 10-Albert St/Nicholson St (East Melbourne) -37.8094 144.9729
# 3 17893 11-Victoria Pde/Nicholson St (East Melbourne) -37.8083 144.9731
# 4 18010 9-La Trobe St/Victoria St (Melbourne City) -37.8076 144.9709
# 5 18011 8-Exhibition St/La Trobe St (Melbourne City) -37.8081 144.9690
# 6 18030 6-Swanston St/La Trobe St (Melbourne City) -37.8095 144.9641
Calculations
Now we have the data, and the distance formula, we can construct the data.table
join
library(data.table)
## set the tram stop data as a data.table
dt1 <- as.data.table(tram_stops)
## add a column that will be used to do the join on
dt1[, joinKey := 1]
## find the dinstance between each point to every other point
## by joining the data to itself
dt2 <- dt1[
dt1
, {
idx = dt.haversine(stop_lat, stop_lon, i.stop_lat, i.stop_lon) < 500 ## in metres
.(stop_id = stop_id[idx],
near_stop_id = i.stop_id)
}
, on = "joinKey"
, by = .EACHI
]
Result
dt2 now holds two columns of stop_id's that are within 500 metres of each other (including the same stop to itself, so this can be removed)
dt2 <- dt2[stop_id != near_stop_id]
Plot
As we're using googleway
, lets plot at some of the results (to do this you need a Google Maps API key, or use another mapping library such as leaflet)
mapKey <- "your_api_key"
## Just pick one to look at
myStop <- 18048
dt_stops <- dt3[stop_id == myStop ]
## get the lat/lons of each stop_id
dt_stops <- dt_stops[
dt1 ## dt1 contains the lat/lons of all the stops
, on = c(near_stop_id = "stop_id")
, nomatch = 0
]
google_map(key = mapKey) %>%
add_circles(data = dt1[stop_id == myStop], lat = "stop_lat", lon = "stop_lon", radius = 500) %>%
add_markers(dt_stops, lat = "stop_lat", lon = "stop_lon")
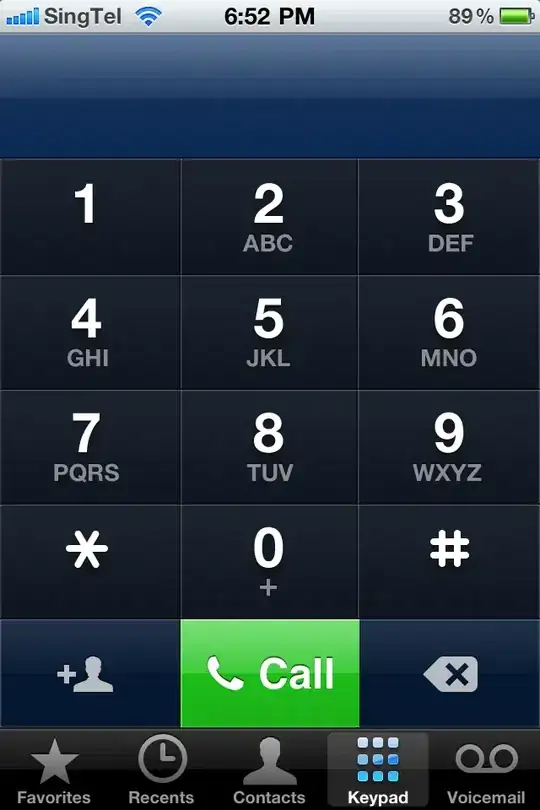
Notes
The data.table
join should be pretty efficient, but obviously the data I've used here is only 51 rows; you'll have to let me know how well this method scales to your data