Let's do a bit more to help you out.
I'm using these packages:
library(rvest)
library(httr)
library(stringi)
library(hrbrthemes)
library(tidyverse)
We'll use this function to clean up column names:
mcga <- function(tbl) {
x <- colnames(tbl)
x <- tolower(x)
x <- gsub("[[:punct:][:space:]]+", "_", x)
x <- gsub("_+", "_", x)
x <- gsub("(^_|_$)", "", x)
x <- make.unique(x, sep = "_")
colnames(tbl) <- x
tbl
}
Since you may want/need to do this for other form combos, we'll start from the main form page:
eval_pg <- read_html("https://opir.fiu.edu/instructor_eval.asp")
We'll eventually grab the actual data that the form submission generates, but we need to "fill in the form" with the option values, so let's get them.
These are the valid parameters for the Term
:
term_nodes <- html_nodes(eval_pg, "select[name='Term'] > option")
data_frame(
name = html_text(term_nodes),
id = html_attr(term_nodes, "value")
) -> Terms
Terms
## # A tibble: 42 x 2
## name id
## <chr> <chr>
## 1 Summer 2017 1175
## 2 Spring 2017 1171
## 3 Fall 2016 1168
## 4 Summer 2016 1165
## 5 Spring 2016 1161
## 6 Fall 2015 1158
## 7 Summer 2015 1155
## 8 Spring 2015 1151
## 9 Fall 2014 1148
## 10 Summer 2014 1145
# ... with 32 more rows
These are the valid parameters for the Coll
:
college_nodes <- html_nodes(eval_pg, "select[name='Coll'] > option")
data_frame(
name = html_text(college_nodes),
id = html_attr(college_nodes, "value")
) -> Coll
Coll
## # A tibble: 12 x 2
## name id
## <chr> <chr>
## 1 All %
## 2 Communication, Architecture & the Arts CARTA
## 3 Arts, Sciences & Education CASE
## 4 Business CBADM
## 5 Engineering & Computing CENGR
## 6 Honors College HONOR
## 7 Hospitality & Tourism Management SHMGT
## 8 Law CLAW
## 9 Nursing & Health Sciences CNHS
## 10 Public Health & Social Work CPHSW
## 11 International & Public Affairs SIPA
## 12 Undergraduate Education UGRED
Make the request like a browser. The form creates an HTTP GET
request with query parameters which opens up a new browser tab/window. We'll make the same request programmatically using the values obtained ^^:
GET("https://opir.fiu.edu/instructor_evals/instr_eval_result.asp",
query = list(
Term = "1171",
Coll = "CBADM",
Dept = "",
RefNum = "",
Crse = "",
Instr = ""
)) -> res
report <- content(res, as="parsed", encoding="UTF-8")
The report
variable has the parsed, HTML/XML document with all the data you want. Now, we'll extract & iterate over each table vs yank them all out at once. This will let us associate metadata with each table.
We'll automagically get metadata fields using this helper vector:
fields <- c("Term:", "Instructor Name:", "Course:", "Department:", "Section:",
"Ref#:", "Title:", "Completed Forms:")
This finds all the tables:
tables_found <- html_nodes(report, xpath=".//table[contains(., 'Term')]")
This sets up a progress bar (the operation takes ~1-2m):
pb <- progress_estimated(length(tables_found))
Now, we iterate over each table we found.
map(tables_found, ~{
pb$tick()$print() # increment progress
tab <- .x # this is just for naming sanity convenience
# Extract the fields
# - Iterate over each field string
# - Find that table cell
# - Extract the text
# - Remove the field string
# - Clean up whitespace
map(fields, ~{
html_nodes(tab, xpath=sprintf(".//td[contains(., '%s')]", .x)) %>%
html_text(trim = TRUE) %>%
stri_replace_first_regex(.x, "") %>%
stri_trim_both() %>%
as.list() %>%
set_names(.x)
}) %>%
flatten() %>%
as_data_frame() %>%
mcga() -> table_meta
# Extract the actual table
# Remove cruft and just get the rows with header and data, turn it back into a table and
# then make a data frame out of it
html_nodes(tab, xpath=".//tr[contains(@class, 'question') or contains(@class, 'tableback')]") %>%
as.character() %>%
paste0(collapse="") %>%
sprintf("<table>%s</table>", .) %>%
read_html() %>%
html_table(header=TRUE) %>%
.[[1]] %>%
mcga() -> table_vals
# you may want to clean up % columns here
# Associate the table values with the table metadata
table_meta$values <- list(table_vals)
# return the combined table
table_meta
}) %>%
bind_rows() -> scraped_tables # bind them all together
We now have a nice, compact nested data frame:
glimpse(scraped_tables)
## Observations: 595
## Variables: 9
## $ term <chr> "1171 - Spring 2017", "1171 - Spring 2017", "1171 - Spring 2017", "1171 - Spring 2017", "1171...
## $ instructor_name <chr> "Elias, Desiree", "Sueiro, Alexander", "Kim, Myung Sub", "Islam, Mohammad Nazrul", "Ling, Ran...
## $ course <chr> "ACG 2021", "ACG 2021", "ACG 2021", "ACG 2021", "ACG 2021", "ACG 2021", "ACG 20...
## $ department <chr> "SCHACCOUNT", "SCHACCOUNT", "SCHACCOUNT", "SCHACCOUNT", "SCHACCOUNT", "SCHACCOUNT", "SCHACCOU...
## $ section <chr> "RVC -1", "U01 -1", "U02 -1", "U03 -1", "U04 -1", "U05 -1", "U06 -1", "U07 -1", "RVC -1", "P8...
## $ ref <chr> "15164 -1", "15393 -1", "15163 -1", "15345 -1", "15346 -1", "17299 -1", "17300 -1", "33841 -1...
## $ title <chr> "ACC Decisions", "ACC Decisions", "ACC Decisions", "ACC Decisions", "ACC Decisions", "ACC Dec...
## $ completed_forms <chr> "57", "47", "48", "43", "21", "12", "48", "31", "44", "8", "82", "43", "20", "13", "59", "12"...
## $ values <list> [<c("Description of course objectives and assignments", "Communication of ideas and informat...
We can "unnest" one "table" at a time:
unnest(scraped_tables[1,])
## # A tibble: 8 x 15
## term instructor_name course department section ref title completed_forms
## <chr> <chr> <chr> <chr> <chr> <chr> <chr> <chr>
## 1 1171 - Spring 2017 Elias, Desiree ACG 2021 SCHACCOUNT RVC -1 15164 -1 ACC Decisions 57
## 2 1171 - Spring 2017 Elias, Desiree ACG 2021 SCHACCOUNT RVC -1 15164 -1 ACC Decisions 57
## 3 1171 - Spring 2017 Elias, Desiree ACG 2021 SCHACCOUNT RVC -1 15164 -1 ACC Decisions 57
## 4 1171 - Spring 2017 Elias, Desiree ACG 2021 SCHACCOUNT RVC -1 15164 -1 ACC Decisions 57
## 5 1171 - Spring 2017 Elias, Desiree ACG 2021 SCHACCOUNT RVC -1 15164 -1 ACC Decisions 57
## 6 1171 - Spring 2017 Elias, Desiree ACG 2021 SCHACCOUNT RVC -1 15164 -1 ACC Decisions 57
## 7 1171 - Spring 2017 Elias, Desiree ACG 2021 SCHACCOUNT RVC -1 15164 -1 ACC Decisions 57
## 8 1171 - Spring 2017 Elias, Desiree ACG 2021 SCHACCOUNT RVC -1 15164 -1 ACC Decisions 57
## # ... with 7 more variables: question <chr>, no_response <chr>, excellent <chr>, very_good <chr>, good <chr>, fair <chr>,
## # poor <chr>
Focus on "just" the question data:
unnest(scraped_tables[1,]) %>%
select(-c(1:8))
## # A tibble: 8 x 7
## question no_response excellent very_good good fair poor
## <chr> <chr> <chr> <chr> <chr> <chr> <chr>
## 1 Description of course objectives and assignments 0.0% 64.9% 14.0% 14.0% 3.5% 3.5%
## 2 Communication of ideas and information 0.0% 56.1% 17.5% 15.8% 5.3% 5.3%
## 3 Expression of expectations for performance in this class 0.0% 63.2% 12.3% 14.0% 8.8% 1.8%
## 4 Availability to assist students in or out of class 3.5% 50.9% 21.1% 10.5% 14.0% 0.0%
## 5 Respect and concern for students 1.8% 59.6% 10.5% 14.0% 10.5% 3.5%
## 6 Stimulation of interest in course 1.8% 52.6% 12.3% 17.5% 7.0% 8.8%
## 7 Facilitation of learning 0.0% 52.6% 19.3% 10.5% 10.5% 7.0%
## 8 Overall assessment of instructor 0.0% 54.4% 15.8% 12.3% 14.0% 3.5%
Or, "unnest" them all:
glimpse(unnest(scraped_tables))
## Observations: 4,760
## Variables: 15
## $ term <chr> "1171 - Spring 2017", "1171 - Spring 2017", "1171 - Spring 2017", "1171 - Spring 2017", "1171...
## $ instructor_name <chr> "Elias, Desiree", "Elias, Desiree", "Elias, Desiree", "Elias, Desiree", "Elias, Desiree", "El...
## $ course <chr> "ACG 2021", "ACG 2021", "ACG 2021", "ACG 2021", "ACG 2021", "ACG 2021", "ACG 20...
## $ department <chr> "SCHACCOUNT", "SCHACCOUNT", "SCHACCOUNT", "SCHACCOUNT", "SCHACCOUNT", "SCHACCOUNT", "SCHACCOU...
## $ section <chr> "RVC -1", "RVC -1", "RVC -1", "RVC -1", "RVC -1", "RVC -1", "RVC -1", "RVC -1", "U01 -1", "U0...
## $ ref <chr> "15164 -1", "15164 -1", "15164 -1", "15164 -1", "15164 -1", "15164 -1", "15164 -1", "15164 -1...
## $ title <chr> "ACC Decisions", "ACC Decisions", "ACC Decisions", "ACC Decisions", "ACC Decisions", "ACC Dec...
## $ completed_forms <chr> "57", "57", "57", "57", "57", "57", "57", "57", "47", "47", "47", "47", "47", "47", "47", "47...
## $ question <chr> "Description of course objectives and assignments", "Communication of ideas and information",...
## $ no_response <chr> "0.0%", "0.0%", "0.0%", "3.5%", "1.8%", "1.8%", "0.0%", "0.0%", "0.0%", "0.0%", "0.0%", "2.1%...
## $ excellent <chr> "64.9%", "56.1%", "63.2%", "50.9%", "59.6%", "52.6%", "52.6%", "54.4%", "66.0%", "59.6%", "66...
## $ very_good <chr> "14.0%", "17.5%", "12.3%", "21.1%", "10.5%", "12.3%", "19.3%", "15.8%", "23.4%", "23.4%", "23...
## $ good <chr> "14.0%", "15.8%", "14.0%", "10.5%", "14.0%", "17.5%", "10.5%", "12.3%", "8.5%", "8.5%", "8.5%...
## $ fair <chr> "3.5%", "5.3%", "8.8%", "14.0%", "10.5%", "7.0%", "10.5%", "14.0%", "0.0%", "6.4%", "2.1%", "...
## $ poor <chr> "3.5%", "5.3%", "1.8%", "0.0%", "3.5%", "8.8%", "7.0%", "3.5%", "2.1%", "2.1%", "0.0%", "0.0%...
We can also deal with the %
here:
unnest(scraped_tables) %>%
mutate_all(~{gsub("%", "", .x)}) %>%
type_convert() %>%
select(-c(1:8))
## # A tibble: 4,760 x 7
## question no_response excellent very_good good fair poor
## <chr> <dbl> <dbl> <dbl> <dbl> <dbl> <dbl>
## 1 Description of course objectives and assignments 0.0 64.9 14.0 14.0 3.5 3.5
## 2 Communication of ideas and information 0.0 56.1 17.5 15.8 5.3 5.3
## 3 Expression of expectations for performance in this class 0.0 63.2 12.3 14.0 8.8 1.8
## 4 Availability to assist students in or out of class 3.5 50.9 21.1 10.5 14.0 0.0
## 5 Respect and concern for students 1.8 59.6 10.5 14.0 10.5 3.5
## 6 Stimulation of interest in course 1.8 52.6 12.3 17.5 7.0 8.8
## 7 Facilitation of learning 0.0 52.6 19.3 10.5 10.5 7.0
## 8 Overall assessment of instructor 0.0 54.4 15.8 12.3 14.0 3.5
## 9 Description of course objectives and assignments 0.0 66.0 23.4 8.5 0.0 2.1
## 10 Communication of ideas and information 0.0 59.6 23.4 8.5 6.4 2.1
## # ... with 4,750 more rows
Then, you can do interesting things like:
unnest(scraped_tables) %>%
mutate_all(~{gsub("%", "", .x)}) %>%
type_convert() -> scraped_tables
group_by(scraped_tables, course) %>%
filter(question == "Description of course objectives and assignments") %>%
gather(resp_cat, resp_val, no_response, excellent, very_good, good, fair, poor) %>%
mutate(resp_val = resp_val/100) %>%
mutate(resp_cat = factor(resp_cat, levels=unique(resp_cat))) %>%
filter(resp_val > 0) %>%
ungroup() -> description_df
ggplot(description_df, aes(resp_val)) +
geom_density(aes(y=..count..), fill="#b2abd288", color="#2d004b") +
scale_x_percent() +
scale_y_comma() +
facet_wrap(~resp_cat) +
labs(title="Description of course objectives and assignments distribution across courses") +
theme_ipsum_rc(grid="XY")
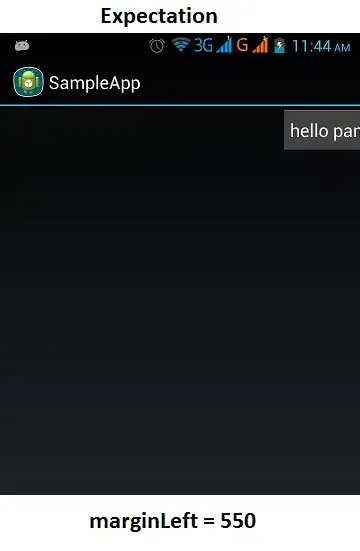
UPDATE
The magrittr
pipes can be daunting at first. Here's a version w/o them:
library(rvest)
library(httr)
library(stringi)
library(hrbrthemes)
library(tidyverse)
mcga <- function(tbl) {
x <- colnames(tbl)
x <- tolower(x)
x <- gsub("[[:punct:][:space:]]+", "_", x)
x <- gsub("_+", "_", x)
x <- gsub("(^_|_$)", "", x)
x <- make.unique(x, sep = "_")
colnames(tbl) <- x
tbl
}
eval_pg <- read_html("https://opir.fiu.edu/instructor_eval.asp")
term_nodes <- html_nodes(eval_pg, "select[name='Term'] > option")
data_frame(
name = html_text(term_nodes),
id = html_attr(term_nodes, "value")
) -> Terms
Terms
college_nodes <- html_nodes(eval_pg, "select[name='Coll'] > option")
data_frame(
name = html_text(college_nodes),
id = html_attr(college_nodes, "value")
) -> Coll
Coll
GET("https://opir.fiu.edu/instructor_evals/instr_eval_result.asp",
query = list(
Term = "1171",
Coll = "CBADM",
Dept = "",
RefNum = "",
Crse = "",
Instr = ""
)) -> res
report <- content(res, as="parsed", encoding="UTF-8")
fields <- c("Term:", "Instructor Name:", "Course:", "Department:", "Section:",
"Ref#:", "Title:", "Completed Forms:")
tables_found <- html_nodes(report, xpath=".//table[contains(., 'Term')]")
pb <- progress_estimated(length(tables_found))
map(tables_found, function(.x) {
pb$tick()$print()
tab <- .x
map(fields, function(.x) {
tmp_field <- html_nodes(tab, xpath=sprintf(".//td[contains(., '%s')]", .x))
tmp_field <- html_text(tmp_field, trim = TRUE)
tmp_field <- stri_replace_first_regex(tmp_field, .x, "")
tmp_field <- stri_trim_both(tmp_field)
tmp_field <- as.list(tmp_field)
tmp_field <- set_names(tmp_field, .x)
tmp_field
}) -> tmp_meta
tmp_meta <- flatten(tmp_meta)
tmp_meta <- as_data_frame(tmp_meta)
table_meta <- mcga(tmp_meta)
tmp_vals <- html_nodes(tab, xpath=".//tr[contains(@class, 'question') or contains(@class, 'tableback')]")
tmp_vals <- as.character(tmp_vals)
tmp_vals <- paste0(tmp_vals, collapse="")
tmp_vals <- sprintf("<table>%s</table>", tmp_vals)
tmp_vals <- read_html(tmp_vals)
tmp_vals <- html_table(tmp_vals, header=TRUE)[[1]]
table_vals <- mcga(tmp_vals)
table_meta$values <- list(table_vals)
table_meta
}) -> list_of_tables
scraped_tables <- bind_rows(list_of_tables)
glimpse(scraped_tables)
unnest(scraped_tables[1,])
tmp_df <- unnest(scraped_tables[1,])
select(tmp_df, -c(1:8))
glimpse(unnest(scraped_tables))
tmp_df <- unnest(scraped_tables)
tmp_df <- mutate_all(tmp_df, function(.x) { gsub("%", "", .x) })
scraped_tables <- type_convert(tmp_df)
(I stripped out the comments since they sections are still the same)