A solution to your problem is achieved by:
- Calculating the kernel density estimate (KDE) of your data,
d
;
- Using
rainbowcolormap(n)
to create a color map m
with n
colors;
- Ploting your data like this:
scatter(x,y,s,d,"fill"); set(gcf(),"color_map",m);
, where s
is the size of the marker in the plot.
Since I could not use the stixbox
toolbox for Scilab, I decided to come up with a workaround for this problem, so prepare yourself of a long answer.
Pure Scilab solution
Firstly, I implemented kernel_density()
on a Scilab macro. Its inputs are x
, an n-by-p data matrix, and h
the bandwidth. What it does is that it counts how many points lie within a circle/sphere/n-sphere of radius h
centered in each data point.
I am not very experienced in this field of Statistics, so I had to read about KDE. It turned out that this solution of mine is actually one KDE method which uses a kernel with constant and equal weight for the neighbors (hence the reason I renamed h
to "bandwidth" instead of just "radius", and why I added a 2*h*n
factor to the calculation).
Also, because of my lack of knowledge, I couldn't implement a way to choose an optimum h
automatically for a given data set, so you'll have to choose it by trial and error. However, reading about the Scipy implementation of gaussian_kde()
, which I saw in the example you provided in your question, and also using hints from this question, and this reference, I came up with a method to reduce to 4 the number of possible h
(if your data has 2 dimensions). Perhaps a real statistician could validate it in the comments, or provide a better way:
- Calculate the covariance matrix of the data set;
- Multiply its square root by Scott's factor:
n ^ (-1 / (p+4))
;
- Plot for all
h
and choose the one that gives the best visualization.
The original kernel_density
function can still be found here and it works fine for around 10³ points. If you're dealing with more than that, keep reading.
C implementation
As noted in the comments section, the Scilab implementation is rather slow. To get better results, I implemented kdec()
in C and linked it to a Scilab macro using ilib_for_link()
. However, this method still have its problems (see warning note at the bottom).
To use this function on Scilab, you should have a compatible C compiler:
- If you use UNIX or UNIX-like system, you don't need to worry.
- If you use Windows, you should follow the instructions of
mingw
toolbox and have it loaded into Scilab environment when you execute kde()
.
First, you have to put kdec.c
in the current Scilab directory.
//kdec.c
#include <math.h>
void kdec(double f[], double x[], double *h, int *n, int *p){
/* x[]: (n*p)-by-1 array of data
* *h: bandwitdh
* *n: the number of points
* *p: the number of dimensions
* f[]: the output
*
* the local neighborhood density can be defined as (for constant weight):
* f(x0) = sum_from i_to n of K(||x_i - x_0|| <= h) / 2hn
* where: x0 is the observed point, which can have p-dimensions;
* K(a) = {1 if a == True
* {0 if a == False
*/
int n_ = *n; int p_ = *p; double h_ = *h;
int d, j, k;
double dif, norm;
for(j = 0; j < n_; j++){
f[j] = 0;
for(k = 0; k < n_; k++){
norm = 0;
for(d = 0; d < p_; d++){
dif = x[k + d*n_] - x[j + d*n_];
norm = norm + dif * dif;
}
norm = sqrt(norm);
if (norm <= h_){
f[j] = f[j] + 1;
}
}
f[j] = f[j] / (2 * (h_) * (n_));
}
}
Then, set kde.sci
to call the kdec
C function and wrap in the new Scilab kde
function.
//kde.sci
if ~isdef('kde') then
ilib_for_link('kdec','kdec.c',[],"c") //compile and create the new shared library
exec('loader.sce',-1); //load library
end
//create a wrapper function to improve interface with interface 'kdec'
function varargout = kde(x,h)
//x: n-by-p matrix of data, each column is a dimension
//h: bandwitdh
[n, p] = size(x); //n: number of points
//p: number of dimensions
x = x(1:$);
if length(h) ~= 1 then
error("kde(x,h): x should be n-by-p matrx; " +...
"h shoud be scalar, positive, and real");
end
f = call('kdec'...
, x , 2, 'd'...
, abs(h), 3, 'd'...
, n , 4, 'i'...
, p , 5, 'i'...
,'out'...
,[n,1] , 1, 'd' );
varargout = list(f)
endfunction
Since I did not get any better in Statistics, you still need to set h
manually. However, after testing it some many times, it seems like the best result for 2D data is given by:
scotts_factor = n ^ (-1 / (p+4))
h = sqrt(abs(cov(A))) .* scotts_factor;
h = h(2);
Here is some test:
exec('kde.sci',-1);
//create data set
n = 1d4;
p = 2;
A = grand((n/2), 1, "nor", 0, 1);
A = [A, A * 3 + grand((n/2), 1, "nor", 0, 1)];
A = [ A ; [ A(:,1) * 0.8 , A(:,2) * 1.3 + 10 ] ];
//calculating bandwidth
scotts_factor = n ^ (-1 / (p+4))
h = sqrt(abs(cov(A))) .* scotts_factor;
h = h(2);
//calculate density
d = kde(A, h);
[d, idx] = gsort(d); //sorting data to plot higher-density points
idx = idx($:-1:1); //over lower-density ones
d = d($:-1:1); //(reversing densities matrix)
A = A(idx,:); //(reordering data matrix)
//plotting
scf(); clf();
scatter(A(:,1), A(:,2), 10, d, "fill");
m = rainbowcolormap(32); //create the rainbow color map
m = m($:-1:1,:); //reverse it to get hotter colors on higher densities
set(gcf(),'color_map',m); //set the desired color map
The output is:
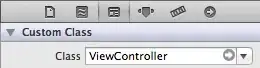
A warning note
Even after implementing it in C, it is still a high-cost function. Because of the two nested for-loops, it is O(n²).
I did a few measurements and these were the results:
n (points) | 10^3 | 5*10^3 | 10^4 | 10^5
-------------+---------+--------+--------+---------
t (seconds) | 0.13751 | 1.2772 | 4.4545 | 323.34
It took more than 5min to run kde()
for 100k points. Since you said you wanted to evaluate 1M points, I wouldn't recommend this solution either. Still, compare it to the pure Scilab solution: it takes around 5s for the latter to work on only 10³ points(!). This is already a huge improvement, but I'm afraid my solution won't get any better. Perhaps you should try reducing the number of samples, or look for other computing tools, such as R.