If your GPU util is below 80%, this is generally the sign of an input pipeline bottleneck. What this means is that the GPU sits idle much of the time, waiting for the CPU to prepare the data: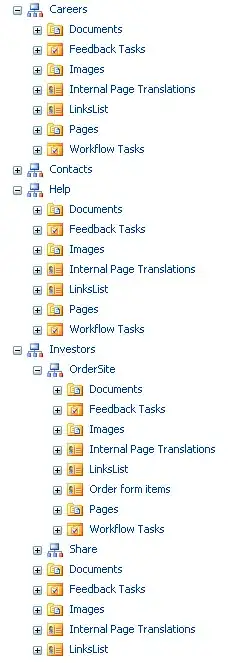
What you want is the CPU to keep preparing batches while the GPU is training to keep the GPU fed. This is called prefetching: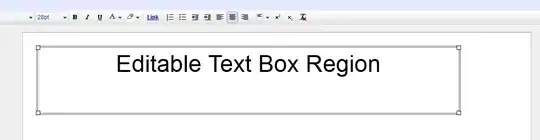
Great, but if the batch preparation is still way longer than the model training, the GPU will still remain idle, waiting for the CPU to finish the next batch. To make the batch preparation faster we can parallelize the different preprocessing operations:
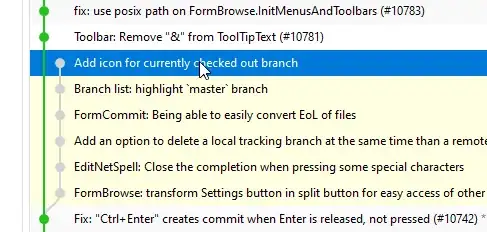
We can go even further by parallelizing I/O:
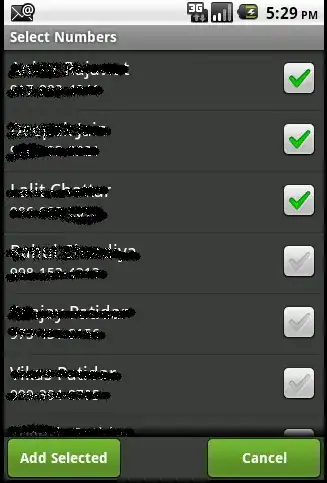
Now to implement this in Keras, you need to use the Tensorflow Data API with Tensorflow version >= 1.9.0. Here is an example:
Let's assume, for the sake of this example that you have two numpy arrays x and y. You can use tf.data for any type of data but this is simpler to understand.
def preprocessing(x, y):
# Can only contain TF operations
...
return x, y
dataset = tf.data.Dataset.from_tensor_slices((x, y)) # Creates a dataset object
dataset = dataset.map(preprocessing, num_parallel_calls=64) # parallel preprocessing
dataset = dataset.batch(batch_size)
dataset = dataset.prefetch(None) # Will automatically prefetch batches
....
model = tf.keras.model(...)
model.fit(x=dataset) # Since tf 1.9.0 you can pass a dataset object
tf.data is very flexible, but as anything in Tensorflow (except eager), it uses a static graph. This can be a pain sometimes but the speed up is worth it.
To go further, you can have a look at the performance guide and the Tensorflow data guide.