I am not sure if this is better than rejection sampling, but here is a solution for uniform sampling of a circle segment (with center angle <= pi) involving the numerical computation of an inverse function. (The uniform sampling of the intersection of two circles can then be composed of the sampling of segments, sectors and triangles - depending on how the intersection can be split into simpler figures.)
First we need to know how to generate a random value Z
with given distribution F
, i.e. we want
P(Z < x) = F(x) <=> (x = F^-1(y))
P(Z < F^-1(y)) = F(F^-1(y)) = y <=> (F is monotonous)
P(F(Z) < y) = y
This means: if Z
has the requested distribution F
, then F(Z)
is distributed uniformly. The other way round:
Z = F^-1(Y),
where Y
is distributed uniformly in [0,1]
, has the requested distribution.
If F
is of the form
/ 0, x < a
F(x) = | (F0(x)-F0(a)) / (F0(b)-F0(a)), a <= x <= b
\ 1, b < x
then we can choose a Y0
uniformly in [F(a),F(b)]
and set Z = F0^-1(Y0)
.
We choose to parametrize the segment by (theta,r)
, where the center angle theta
is measured from one segment side. When the segment's center angle is alpha
, the area of the segment intersected with a sector of angle theta
starting where the segment starts is (for the unit circle, theta
in [0,alpha/2]
)
F0_theta(theta) = 0.5*(theta - d*(s - d*tan(alpha/2-theta)))
where s = AB/2 = sin(alpha/2)
and d = dist(M,AB) = cos(alpha/2)
(the distance of the circle center to the segment). (The case alpha/2 <= theta <= alpha
is symmetric and not considered here.)
We need a random theta
with P(theta < x) = F_theta(x)
. The inverse of F_theta
cannot be computed symbolically - it must be determined by some optimization algorithm (e.g. Newton-Raphson).
Once theta
is fixed we need a random radius r
in the range
[r_min, 1], r_min = d/cos(alpha/2-theta).
For x
in [0, 1-r_min]
the distribution must be
F0_r(x) = (x+r_min)^2 - r_min^2 = x^2 + 2*x*r_min.
Here the inverse can be computed symbolically:
F0_r^-1(y) = -r_min + sqrt(r_min^2+y)
Here is an implementation in Python for proof of concept:
from math import sin,cos,tan,sqrt
from scipy.optimize import newton
# area of segment of unit circle
# alpha: center angle of segment (0 <= alpha <= pi)
def segmentArea(alpha):
return 0.5*(alpha - sin(alpha))
# generate a function that gives the area of a segment of a unit circle
# intersected with a sector of given angle, where the sector starts at one end of the segment.
# The returned function is valid for [0,alpha/2].
# For theta=alpha/2 the returned function gives half of the segment area.
# alpha: center angle of segment (0 <= alpha <= pi)
def segmentAreaByAngle_gen(alpha):
alpha_2 = 0.5*alpha
s,d = sin(alpha_2),cos(alpha_2)
return lambda theta: 0.5*(theta - d*(s - d*tan(alpha_2-theta)))
# generate derivative function generated by segmentAreaByAngle_gen
def segmentAreaByAngleDeriv_gen(alpha):
alpha_2 = 0.5*alpha
d = cos(alpha_2)
return lambda theta: (lambda dr = d/cos(alpha_2-theta): 0.5*(1 - dr*dr))()
# generate inverse of function generated by segmentAreaByAngle_gen
def segmentAreaByAngleInv_gen(alpha):
x0 = sqrt(0.5*segmentArea(alpha)) # initial guess by approximating half of segment with right-angled triangle
return lambda area: newton(lambda theta: segmentAreaByAngle_gen(alpha)(theta) - area, x0, segmentAreaByAngleDeriv_gen(alpha))
# for a segment of the unit circle in canonical position
# (i.e. symmetric to x-axis, on positive side of x-axis)
# generate uniformly distributed random point in upper half
def randomPointInSegmentHalf(alpha):
FInv = segmentAreaByAngleInv_gen(alpha)
areaRandom = random.uniform(0,0.5*segmentArea(alpha))
thetaRandom = FInv(areaRandom)
alpha_2 = 0.5*alpha
d = cos(alpha_2)
rMin = d/cos(alpha_2-thetaRandom)
secAreaRandom = random.uniform(0, 1-rMin*rMin)
rRandom = sqrt(rMin*rMin + secAreaRandom)
return rRandom*cos(alpha_2-thetaRandom), rRandom*sin(alpha_2-thetaRandom)
The visualisation seems to verify uniform distribution (of the upper half of a segment with center angle pi/2
):
import matplotlib.pyplot as plot
segmentPoints = [randomPointInSegmentHalf(pi/2) for _ in range(500)]
plot.scatter(*zip(*segmentPoints))
plot.show()
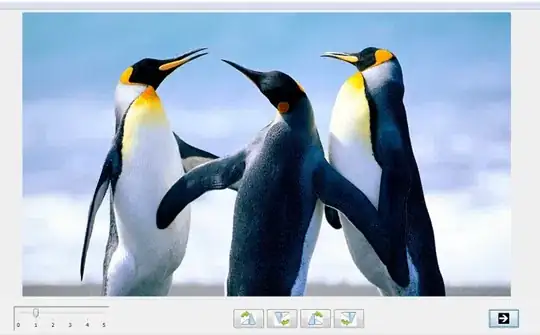