Shuffling complexity
The time complexity of np.shuffle is O(n) as explained here, so at least in the programs below it should not be a bottleneck, but let's explore different aspects of the question below.
Problem formalization and complexity
If I understand correctly, your problem can be formulated as a bipartite graph with N_u user nodes, N_s website nodes and N_v edges between them, reflecting the visits, see panel (A) below.
Then counting the number of users who visited the same pairs of websites (your counterdict dictionary) simply corresponds to the
weighted bipartite network projection onto the website nodes, see panel (B) below.
The complexity of the weighted bipartite network projection for the brute-force approach is O(N_u^2*N_s). Consequently, when iterating over multiple randomizations, the O(N_v) from shuffling should be neglible (unless of course N_v > N_u^2*N_s). There are also approaches for sampling bipartite network projections in case of very large graphs.
In the small dummy example below, using bipartite network projection is around 150 times faster than your implementation (0.00024 vs 0.03600 seconds) and yields identical results.
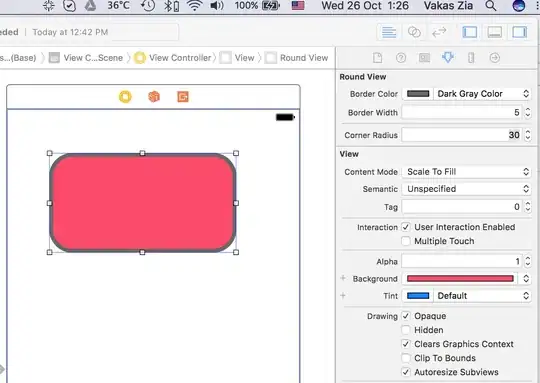
The code 1
# import modules
import collections
import itertools
import time
import matplotlib.pyplot as plt
import numpy as np
import networkx as nx
import pandas as pd
import pymc3 as pm
# generate fake data for demonstration purposes
np.random.seed(0)
nvisits = 24
nusers = 12
nsites = 6
userz = np.random.choice(['U'+str(user).zfill(3) for user in range(nusers)], nvisits)
sitez = np.random.choice(range(nsites), nvisits)
users = np.unique(userz)
sites = np.unique(sitez)
# copy original implementation from the question
def get_site_pairs(users, sites, userz, sitez):
dct = dict()
dct['user'] = userz
dct['site'] = sitez
name=pd.DataFrame(dct)
groups=name.groupby('user')
pairs = []
for ui in users:
userdata = groups.get_group(ui)
userdata=userdata.drop_duplicates()
site_list=userdata['site'].values
pair=list(itertools.combinations(site_list, 2))
for j in pair:
pairs.append(sorted(j))
site_exp=pd.DataFrame(pairs, columns=['node1', 'node2'], dtype=str)
site_exp['pair'] = site_exp['node1']+'<--->'+site_exp['node2']
counterdict=collections.Counter(site_exp['pair'].values)
counterdict=pd.DataFrame(list(counterdict.items()), columns=['pair','site_obs'])
return counterdict
temp = time.time()
counterdict = get_site_pairs(users, sites, userz, sitez)
print (time.time() - temp)
# 0.03600 seconds
# implement bipartite-graph based algorithm
def get_site_graph(users, sites, userz, sitez):
graph = nx.Graph()
graph.add_nodes_from(users, bipartite=0)
graph.add_nodes_from(sites, bipartite=1)
graph.add_edges_from(zip(userz, sitez))
projection = nx.algorithms.bipartite.projection.weighted_projected_graph(graph, sites)
return graph, projection
temp = time.time()
graph, projection = get_site_graph(users, sites, userz, sitez)
print (time.time() - temp)
# 0.00024 seconds
# verify equality of results
for idr, row in counterdict.iterrows():
u, v = np.array(row['pair'].split('<--->')).astype(np.int)
pro = projection[u][v]
assert row['site_obs'] == pro['weight']
# prepare graph layouts for plotting
layers = nx.bipartite_layout(graph, userz)
circle = nx.circular_layout(projection)
width = np.array(list(nx.get_edge_attributes(projection, 'weight').values()))
width = 0.2 + 0.8 * width / max(width)
degrees = graph.degree()
# plot graphs
fig = plt.figure(figsize=(16, 9))
plt.subplot(131)
plt.title('(A)\nbipartite graph', loc='center')
nx.draw_networkx(graph, layers, width=2)
plt.axis('off')
plt.subplot(132)
plt.title('(B)\none-mode projection (onto sites)', loc='center')
nx.draw_networkx(projection, circle, edge_color=plt.cm.Greys(width), width=2)
plt.axis('off')
plt.subplot(133)
plt.title('(C)\nrandomization setup', loc='center')
nx.draw_networkx(graph, layers, width=2)
plt.text(*(layers['U000']-[0.1, 0]), '$n_u=%s$' % degrees['U000'], ha='right')
plt.text(*(layers[0]+[0.1, 0]), '$n_s=%s$' % degrees[0], ha='left')
plt.text(*(layers[1]+[0.1, 0]), '$n_t=%s$' % degrees[1], ha='left')
plt.text(0.3, -1, '$N_v=%s$' % nvisits)
plt.plot([0.3]*2, [-1, 1], lw=160, color='white')
plt.axis('off')
Network randomization and PyMC3 simulation
When randomizing the user list, as mentioned in the question, we can get a distribution of site-site connections. For networks of moderate size this should be reasonably fast, see argument regarding shuffling complexity above and code example below.
If the network is too large, sampling may be an option and the graph formalization helps to set up the sampling scenario, see panel (C) above. For given n_u and n_s edge randomization corresponds to random draws from a multivariate hypergeometric distribution.
Unfortunately, PyMC3 does not yet support hypergeometic distributions. In case this helps, I added a small example using PyMC3 and sampling from a simple binomial distribution below. The black histograms show the distribution of site-site connections n_{s,t} from full network randomization and bipartite projection.
The gray vertical line indicates that the maximum n_{s,t} <= min(N_u, n_s, n_t).
The red dots are from the binomial approximation which assumes there are nvisits*(nvisits-1)/2 pairs of edges to be distributed and the chance of connecting nodes s and t via user u is p_s * p_u * p_t * p_u, with p_x = n_x / N_x. Here, all edges are assumed to be independent and the result obviously yields an approximation only.
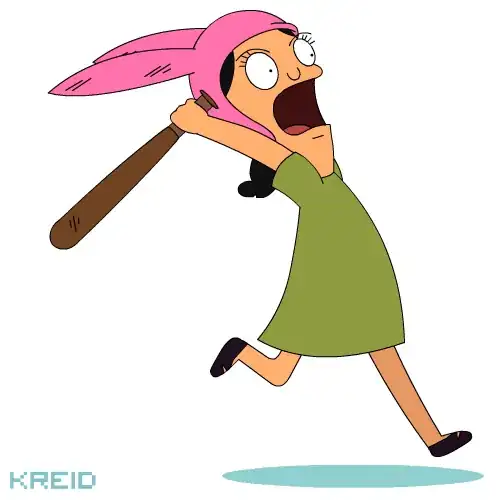
The code 2
# randomize user visits and store frequencies of site-site connections
niters = 1000
matrix = np.zeros((niters, nsites, nsites))
siten = collections.Counter(sitez)
for i in range(niters):
np.random.shuffle(userz)
graph, projection = get_site_graph(users, sites, userz, sitez)
edges = projection.edges(data=True)
for u, v, d in edges:
matrix[i, u, v] = d['weight']
# define PyMC3 function for sampling from binomial distribution
def sample_pymc3(prob, number, bins, draws=1000):
with pm.Model() as model:
nst = pm.Binomial('nst', n=number, p=prob)
trace = pm.sample(draws=draws, step=pm.Metropolis())
nst = trace.get_values('nst')
freqs = [np.mean(nst == val) for val in bins]
return freqs
# define auxiliary variables
# probability to select site s by chance
probs = [np.mean(sitez == s) for s in sites]
# probability to select user u by chance
probu = [np.mean(userz == u) for u in users]
# plot connectivity statistics
nsitez = min(5, nsites)
bins = np.arange(9)
number = nvisits*(nvisits-1)/2
fig, axis = plt.subplots(nrows=nsitez,
ncols=nsitez,
figsize=(16, 9))
for s in sites[:nsitez]:
for t in sites[:nsitez]:
# prepare axis
axia = axis[s, t]
if t <= s:
axia.set_axis_off()
continue
# plot histogram
axia.hist(matrix[:, s, t], bins=bins, histtype='step', density=True,
zorder=-10, align='left', color='black', lw=2)
axia.plot([min(siten[s], siten[t], nusers)+0.5]*2, [0, 0.5], lw=4, color='gray')
# approximate probabilities using PyMC3
prob = np.sum([probs[s] * pru * probs[t] * pru for pru in probu])
freqs = sample_pymc3(prob, number, bins)
freqs = sample_pymc3(prob, number, bins)
axia.scatter(bins, freqs, color='red')
# set axes
nst = '$n_{s=%s,t=%s}$' % (s, t)
axia.set_xlabel(nst)
if t == s+1:
axia.set_ylabel('frequency')
plt.suptitle('distribution of the number $n_{s,t}$\nof connections between site $s$ and $t$')
plt.tight_layout(rect=[-0.2, -0.2, 1, 0.9])