Here is a way to have multiple graphs in one plot grouped into one single run, using add_custom_scalar on PyTorch.
What I get:
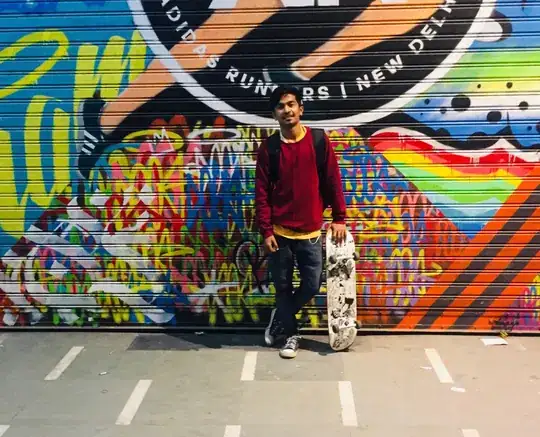
The corresponding complete running code:
from torch.utils.tensorboard import SummaryWriter
import math
layout = {
"ABCDE": {
"loss": ["Multiline", ["loss/train", "loss/validation"]],
"accuracy": ["Multiline", ["accuracy/train", "accuracy/validation"]],
},
}
writer = SummaryWriter()
writer.add_custom_scalars(layout)
epochs = 10
batch_size = 50
for epoch in range(epochs):
for index in range(batch_size):
global_batch_index = epoch * batch_size + index
train_loss = math.exp(-0.01 * global_batch_index)
train_accuracy = 1 - math.exp(-0.01 * global_batch_index)
writer.add_scalar("loss/train", train_loss, global_batch_index)
writer.add_scalar("accuracy/train", train_accuracy, global_batch_index)
validation_loss = train_loss + 0.1
validation_accuracy = train_accuracy - 0.1
writer.add_scalar("loss/validation", validation_loss, global_batch_index)
writer.add_scalar("accuracy/validation", validation_accuracy, global_batch_index)
writer.close()
Please note the used tab on top left on the window is not SCALARS
but CUSTOM SCALARS