When working with hydro-meteorological data, I usually use xts
and hydroTSM
packages as they have many functions for data aggregation.
You didn't provide any data so I created one for demonstration purpose
library(xts)
library(hydroTSM)
# Generate random data
set.seed(2018)
date = seq(from = as.Date("2016-01-01"), to = as.Date("2018-12-31"),
by = "days")
temperature = runif(length(date), -15, 35)
dat <- data.frame(date, temperature)
# Convert to xts object for xts & hydroTSM functions
dat_xts <- xts(dat[, -1], order.by = dat$date)
# All daily, monthly & annual series in one plot
hydroplot(dat_xts, pfreq = "dma", var.type = "Temperature")

# Weekly average
dat_weekly <- apply.weekly(dat_xts, FUN = mean)
plot(dat_weekly)
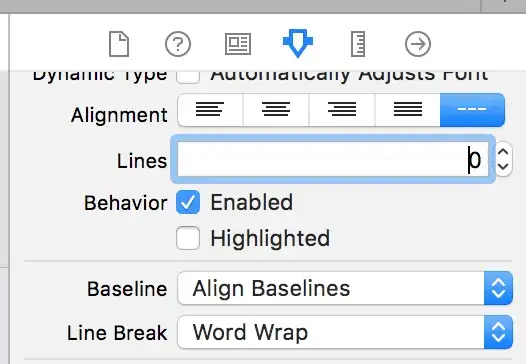
# Monthly average
dat_monthly <- daily2monthly(dat_xts, FUN = mean, na.rm = TRUE)
plot.zoo(dat_monthly, xaxt = "n", xlab = "")
axis.Date(1, at = pretty(index(dat_monthly)),
labels = format(pretty(index(dat_monthly)), format = "%b-%Y"),
las = 1, cex.axis = 1.1)
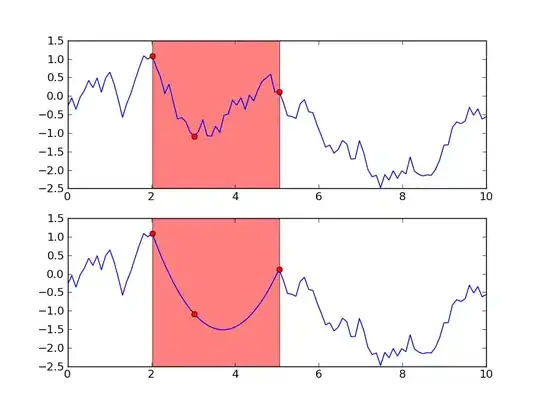
# Seasonal average: need to specify the months
dat_seasonal <- dm2seasonal(dat_xts, season = "DJF", FUN = mean, na.rm = TRUE)
plot(dat_seasonal)

# Annual average
dat_annual <- daily2annual(dat_xts, FUN = mean, na.rm = TRUE)
plot(dat_annual)

Edit: using OP's data
df <- readr::read_csv2("Temp_2014_Hour.csv")
str(df)
# Convert DATE to Date object & put in a new column
df$date <- as.Date(df$DATE, format = "%d/%m/%Y")
dat <- df[, c("date", "VALUE")]
str(dat)
dat_xts <- xts(dat[, -1], order.by = dat$date)
Created on 2018-02-28 by the reprex package (v0.2.0).