One way to accomplish this is using morphological operations and contour detection.
With the former you essentially "bleed" all characters into a big chunky blob. With the latter, you locate these blobs in your image and extract the ones that seem interesting (meaning: big enough).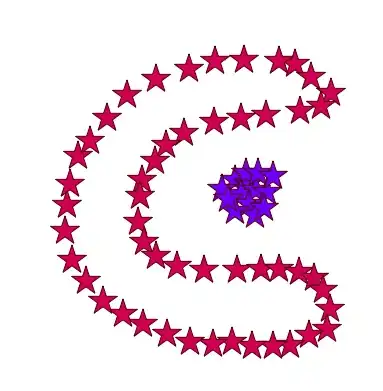
Script used:
import cv2
import sys
SCALE = 4
AREA_THRESHOLD = 427505.0 / 2
def show_scaled(name, img):
try:
h, w = img.shape
except ValueError:
h, w, _ = img.shape
cv2.imshow(name, cv2.resize(img, (w // SCALE, h // SCALE)))
def main():
img = cv2.imread(sys.argv[1])
img = img[10:-10, 10:-10] # remove the border, it confuses contour detection
gray = cv2.cvtColor(img, cv2.COLOR_BGR2GRAY)
show_scaled("original", gray)
# black and white, and inverted, because
# white pixels are treated as objects in
# contour detection
thresholded = cv2.adaptiveThreshold(
gray, 255,
cv2.ADAPTIVE_THRESH_MEAN_C, cv2.THRESH_BINARY_INV,
25,
15
)
show_scaled('thresholded', thresholded)
# I use a kernel that is wide enough to connect characters
# but not text blocks, and tall enough to connect lines.
kernel = cv2.getStructuringElement(cv2.MORPH_RECT, (13, 33))
closing = cv2.morphologyEx(thresholded, cv2.MORPH_CLOSE, kernel)
im2, contours, hierarchy = cv2.findContours(closing, cv2.RETR_EXTERNAL, cv2.CHAIN_APPROX_SIMPLE)
show_scaled("closing", closing)
for contour in contours:
convex_contour = cv2.convexHull(contour)
area = cv2.contourArea(convex_contour)
if area > AREA_THRESHOLD:
cv2.drawContours(img, [convex_contour], -1, (255,0,0), 3)
show_scaled("contours", img)
cv2.imwrite("/tmp/contours.png", img)
cv2.waitKey()
if __name__ == '__main__':
main()
Then all you need is to compute the bounding box of the contour, and cut it from the original image. Add a bit of a margin and feed the whole thing to tesseract.