Indeed, numpy.linalg.svd
and numpy.linalg.eigh
do not call the same routine of Lapack. On the one hand, numpy.linalg.eigh
refers to LAPACK's dsyevd()
while numpy.linalg.svd
makes use LAPACK's dgesdd()
.
The common point between these routines is the use of Cuppen's divide and conquer algorithm, first designed to solve tridiagonal eigenvalue problems. For instance, dsyevd()
only handles Hermitian matrix and performs the following steps, only if eigenvectors are required:
Reduce matrix to tridiagonal form using DSYTRD()
Compute the eigenvectors of the tridiagonal matrix using the divide and conquer algorithm, through DSTEDC()
Apply the Householder reflection reported by DSYTRD() using DORMTR().
On the contrary, to compute the SVD, dgesdd()
performs the following steps, in the case job==A (U and VT required):
- Bidiagonalize A using
dgebrd()
- Compute the SVD of the bidiagonal matrix using divide and conquer algorithm using
DBDSDC()
- Revert the bidiagonalization using using the matrices P and Q returned by
dgebrd()
applying dormbr()
twice, once for U and once for V
While the actual operations performed by LAPACK are very different, the strategies are globally similar. It may stem from the fact that computing the SVD of a general matrix A is similar to performing the eigendecomposition of the symmetric matrix A^T.A.
Regarding accuracy and performances of lapack divide and conquer SVD, see This survey of SVD methods:
- They often achieve the accuracy of QR-based SVD, though it is not proven
- The worst case is O(n^3) if no deflation occurs, but often proves better than that
- The memory requirement is 8 times the size of the matrix, which can become prohibitive
Regarding the symmetric eigenvalue problem, the complexity is 4/3n^3 (but often proves better than that) and the memory footprint is about 2n^2 plus the size of the matrix. Hence, the best choice is likely numpy.linalg.eigh
if your matrix is symmetric.
The actual complexity can be computed for your particular matrices using the following code:
import numpy as np
from matplotlib import pyplot as plt
from scipy.optimize import curve_fit
# see https://stackoverflow.com/questions/41109122/fitting-a-curve-to-a-power-law-distribution-with-curve-fit-does-not-work
def func_powerlaw(x, m, c):
return np.log(np.abs( x**m * c))
import time
start = time.time()
print("hello")
end = time.time()
print(end - start)
timeev=[]
timesvd=[]
size=[]
for n in range(10,600):
print n
size.append(n)
A=np.zeros((n,n))
#populate A, 1D diffusion.
for j in range(n):
A[j,j]=2.
if j>0:
A[j-1,j]=-1.
if j<n-1:
A[j+1,j]=-1.
#EIG
Aev=A.copy()
start = time.time()
w,v=np.linalg.eigh(Aev,'L')
end = time.time()
timeev.append(end-start)
Asvd=A.copy()
start = time.time()
u,s,vh=np.linalg.svd(Asvd)
end = time.time()
timesvd.append(end-start)
poptev, pcov = curve_fit(func_powerlaw, size[len(size)/2:], np.log(timeev[len(size)/2:]),p0=[2.1,1e-7],maxfev = 8000)
print poptev
poptsvd, pcov = curve_fit(func_powerlaw, size[len(size)/2:], np.log(timesvd[len(size)/2:]),p0=[2.1,1e-7],maxfev = 8000)
print poptsvd
plt.figure()
fig, ax = plt.subplots()
plt.plot(size,timeev,label="eigh")
plt.plot(size,[np.exp(func_powerlaw(x, poptev[0], poptev[1])) for x in size],label="eigh-adjusted complexity: "+str(poptev[0]))
plt.plot(size,timesvd,label="svd")
plt.plot(size,[np.exp(func_powerlaw(x, poptsvd[0], poptsvd[1])) for x in size],label="svd-adjusted complexity: "+str(poptsvd[0]))
ax.set_xlabel('n')
ax.set_ylabel('time, s')
#plt.legend(loc="upper left")
ax.legend(loc="lower right")
ax.set_yscale("log", nonposy='clip')
fig.tight_layout()
plt.savefig('eigh.jpg')
plt.show()
For such 1D diffusion matrices, eigh outperforms svd, but the actual complexity are similar, slightly lower than n^3, something like n^2.5.
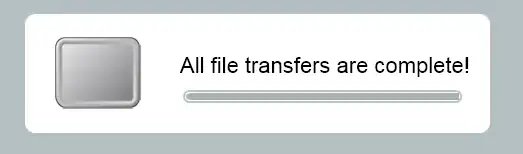
Checking of the accuracy could be performed as well.