Claim: in a 2D space, a set of points belong the same cluster, if (and only if) BOTH
their projections on OX are clustered, and their projections on OY are clustered.
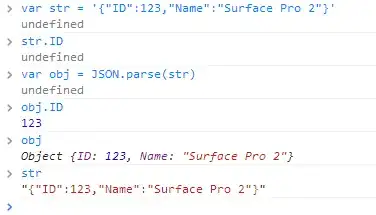
on OX, the projections of A,B,C,D are clustered; on OY, the projections of A,B,C,E are clustered;
overall, A,B and C are clustered.
Determining which points are clustered, on a 1D space:
Let us have some points spread on the real axis. Intuitively, two points belong
the same cluster if they are separated by a 'small distance'; if they are separated
by a 'large distance', they belong different clusters. Now we must determine what is
a 'large distance' and what is a 'small distance'.
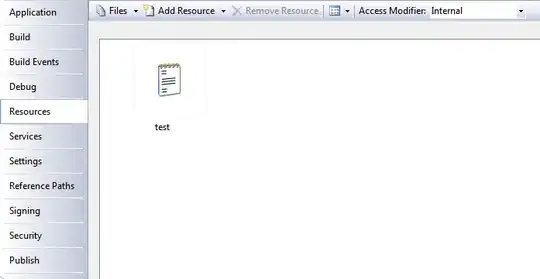
Let us sort all the distances, and look for a threshold.
For the set of points with OX coordinates being [0,1,3,4,14,15,16,19,29,30,31], the distances
between consecutive points will be [1,2,1,10,1,1,3,10,1,1]. The same set of distances, when sorted,
will look like [1,1,1,1,1,1,2,3,10,10]. There is a threshold between 3 and 10, so we shall denote all
distances <=3 as 'small distances', and all others as 'large distances'.
How do we pick the threshold? By doing the difference between two neighboring elements. In our
example, 10-3=7 is the larges difference.
If there are multiple thresholds of equal values, pick
the right-most; picking the right most threshold results in having few 'large distances', otherwise,
many of the distances will be considered large, and, accordingly ,there will be many clusters.
But it is up to your business requirements. You can group 30 locations as 3 clusters each of 10 locations,
or 10 clusters of 3 locations each.
If the sorted distances are something like [2,4,6,8,10] (no candidate for threshold), then pick some percentile
like the 75-percentile: the top quarter will be 'large distance', all the others - 'small distances'
Grouping points into clusters, according to their projections on OX:
Now that we know how to cluster real numbers, let us take the OX projections of the points, and cluster them.
Let us have the following points, along with their OX coordinates:
P1(101), P2(12), P3(201), P4(13), P5(202), P6(11), P7(102);
Same points, sorted by their OX coordinate:
P6(11), P2(12), P4(13), P1(101), P7(102), P3(201), P5(202);
Next, we shall do another grouping, by the OY projections.
Observation 1: When the point's indexes are sorted, the OX projections are not; when we sort the OX projections,
now the indexes will not be sorted.
Observation 2: when doing the clustering using the OX projections, we should not expect to obtain the same
clusters as when we cluster using the OY projections. In fact, we will intersect the obtained results.
The clustering results are different because a point's OY coordinate is totally independent from its OX coordinate.
Thus, a totally different set of values on the OY axis.
Determining the actual clusters:
We have previously done some clustering on both the OX and OY projections, obtaining
different groupings of the same points. Now we will intersect these clusters, looking for common points.
Coming back to our first picture, clustering after OX gives (A,B,C,D) cluster, after OY we get (A,B,C,E),
the intersection of these sets will be (A,B,C) - the final cluster. But this was a simple example.
The general strategy is to do a carthesian product of the clusters on OX, with the clusters obtained on OY.
For each such element of the carthesian product, we will intersect the elements in the OX cluster, with the elements on the OY cluster.
If we got 3 clusters on OX and 4 clusters on OY, the carthesian product will have 12 elements. Let's pick one of them.
It is a pair of two clusters A and B: A is a cluster on OX, B is a cluster on OY. If A and B have some points in common,
then these points are indeed a cluster.
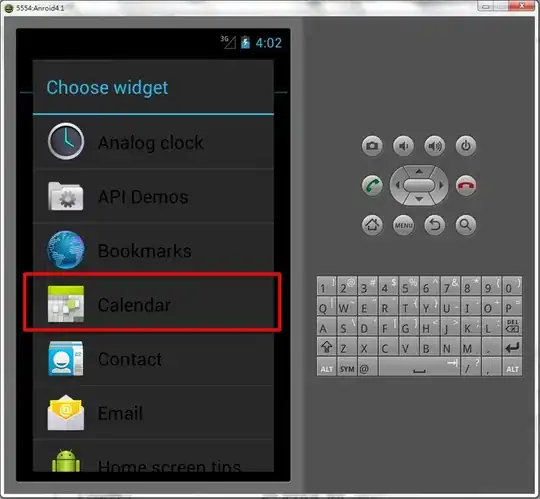
In the above example, from 7 points, we have obtained only one single cluster. Not impressive. But we
can further join neighbor clusters. Let's not forget that the orange segments represent 'small distances',
while the black segments represent 'large distances'. In the above picture, from the 'cluster' P1 to the clusters
P5 or P3+P7, there is only one 'large distance'. From the cluster P3+P7, to the cluster P4, there are two large distances plus
one small distance.
disclaimer: this procedure treats the world map as a rectangle (meridians are parallel lines which will never intersect).
Also, instead of viewing the meridians of 1 degree and 179 degrees as close to each other, they will appear really far apart.
(the distance between them will be 178 degrees, instead of 2 degrees)
However, this is not an issue, because most of the time, the act of delivery has a regional nature, or is, at most, country-level.
As long as your country is not traversed by the 180 meridian, you are just fine.